Document Modeling with Graph Attention Networks for Multi-grained Machine Reading Comprehension
Bo Zheng, Haoyang Wen, Yaobo Liang, Nan Duan, Wanxiang Che, Daxin Jiang, Ming Zhou, Ting Liu
Question Answering Long Paper
Session 11B: Jul 8
(06:00-07:00 GMT)
Session 13B: Jul 8
(13:00-14:00 GMT)
Abstract:
Natural Questions is a new challenging machine reading comprehension benchmark with two-grained answers, which are a long answer (typically a paragraph) and a short answer (one or more entities inside the long answer). Despite the effectiveness of existing methods on this benchmark, they treat these two sub-tasks individually during training while ignoring their dependencies. To address this issue, we present a novel multi-grained machine reading comprehension framework that focuses on modeling documents at their hierarchical nature, which are different levels of granularity: documents, paragraphs, sentences, and tokens. We utilize graph attention networks to obtain different levels of representations so that they can be learned simultaneously. The long and short answers can be extracted from paragraph-level representation and token-level representation, respectively. In this way, we can model the dependencies between the two-grained answers to provide evidence for each other. We jointly train the two sub-tasks, and our experiments show that our approach significantly outperforms previous systems at both long and short answer criteria.
You can open the
pre-recorded video
in a separate window.
NOTE: The SlidesLive video may display a random order of the authors.
The correct author list is shown at the top of this webpage.
Similar Papers
RikiNet: Reading Wikipedia Pages for Natural Question Answering
Dayiheng Liu, Yeyun Gong, Jie Fu, Yu Yan, Jiusheng Chen, Daxin Jiang, Jiancheng Lv, Nan Duan,
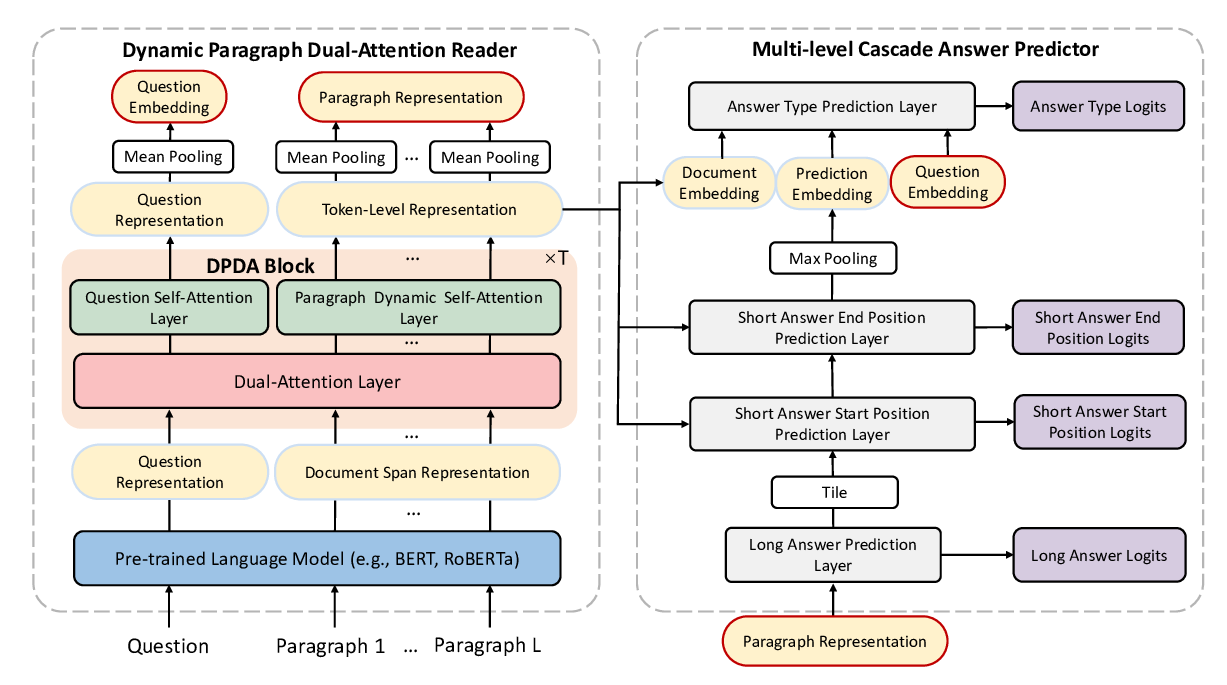
STARC: Structured Annotations for Reading Comprehension
Yevgeni Berzak, Jonathan Malmaud, Roger Levy,
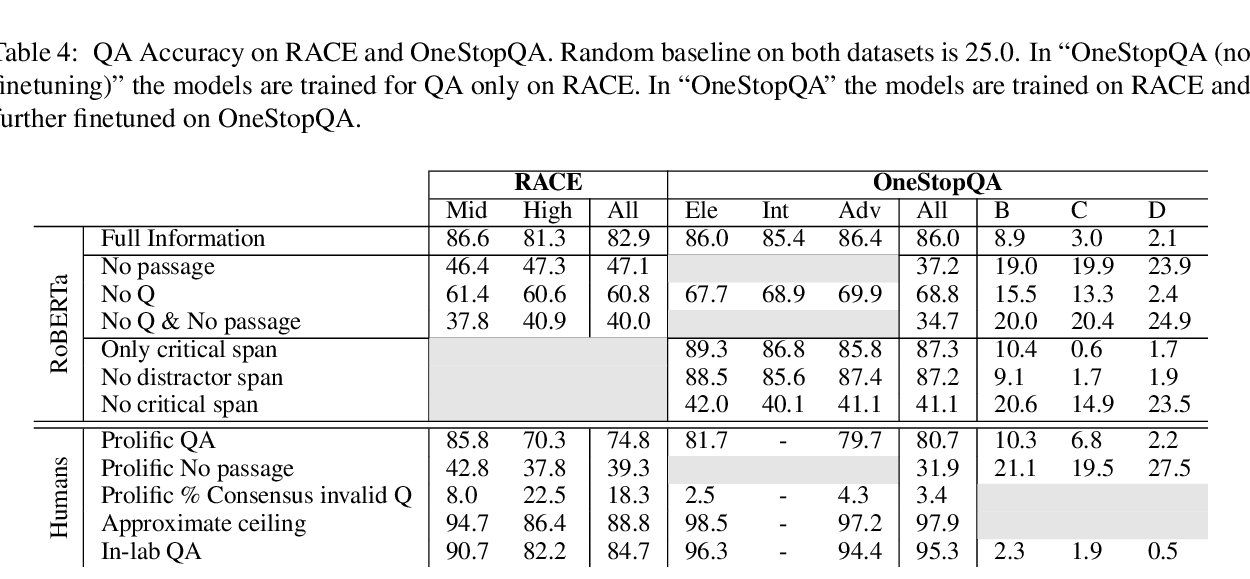
Span Selection Pre-training for Question Answering
Michael Glass, Alfio Gliozzo, Rishav Chakravarti, Anthony Ferritto, Lin Pan, G P Shrivatsa Bhargav, Dinesh Garg, Avi Sil,
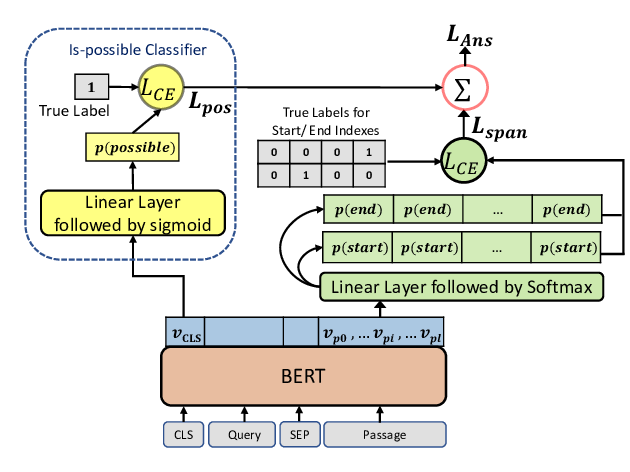
Learning Source Phrase Representations for Neural Machine Translation
Hongfei Xu, Josef van Genabith, Deyi Xiong, Qiuhui Liu, Jingyi Zhang,
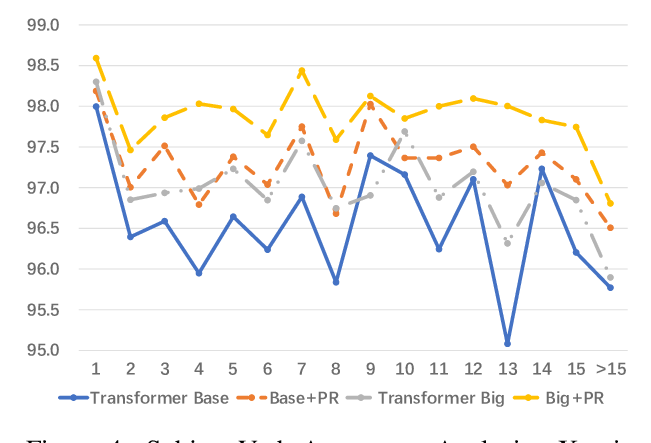