Effective Estimation of Deep Generative Language Models
Tom Pelsmaeker, Wilker Aziz
Machine Learning for NLP Long Paper
Session 12B: Jul 8
(09:00-10:00 GMT)
Session 13A: Jul 8
(12:00-13:00 GMT)
Abstract:
Advances in variational inference enable parameterisation of probabilistic models by deep neural networks. This combines the statistical transparency of the probabilistic modelling framework with the representational power of deep learning. Yet, due to a problem known as posterior collapse, it is difficult to estimate such models in the context of language modelling effectively. We concentrate on one such model, the variational auto-encoder, which we argue is an important building block in hierarchical probabilistic models of language. This paper contributes a sober view of the problem, a survey of techniques to address it, novel techniques, and extensions to the model. To establish a ranking of techniques, we perform a systematic comparison using Bayesian optimisation and find that many techniques perform reasonably similar, given enough resources. Still, a favourite can be named based on convenience. We also make several empirical observations and recommendations of best practices that should help researchers interested in this exciting field.
You can open the
pre-recorded video
in a separate window.
NOTE: The SlidesLive video may display a random order of the authors.
The correct author list is shown at the top of this webpage.
Similar Papers
Not All Claims are Created Equal: Choosing the Right Statistical Approach to Assess Hypotheses
Erfan Sadeqi Azer, Daniel Khashabi, Ashish Sabharwal, Dan Roth,
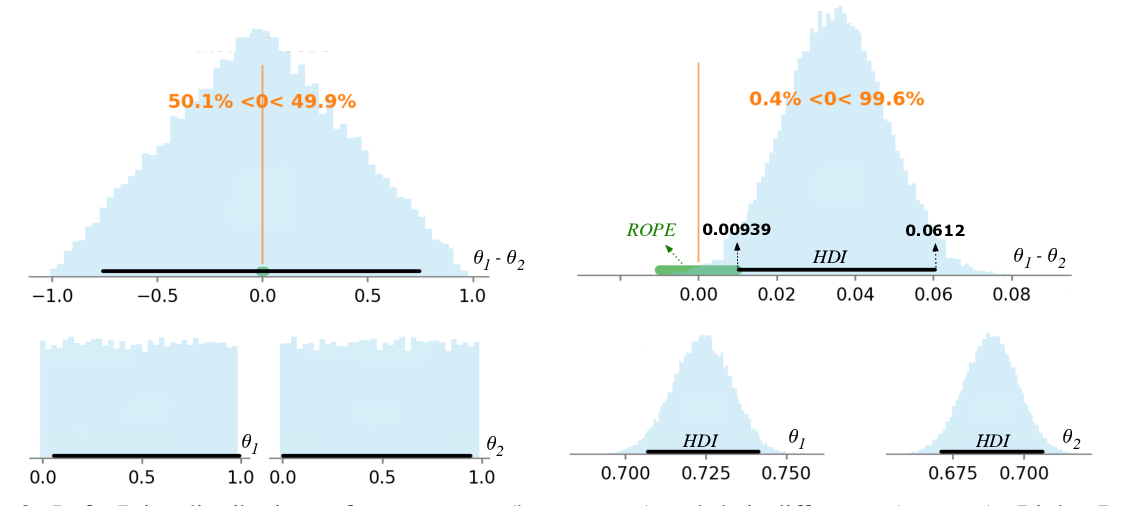
Generalized Entropy Regularization or: There's Nothing Special about Label Smoothing
Clara Meister, Elizabeth Salesky, Ryan Cotterell,
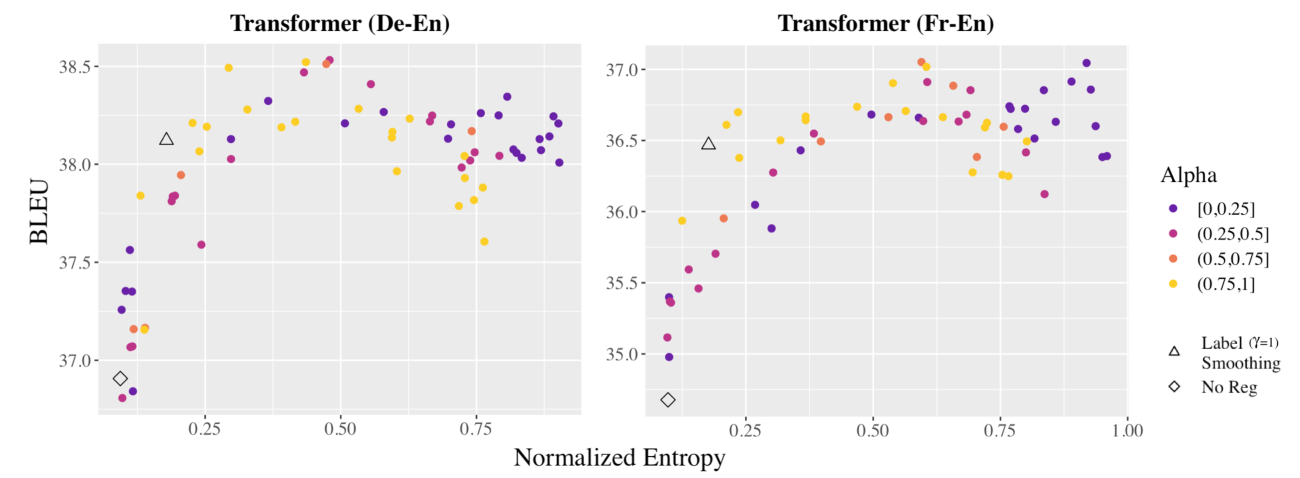
Predictive Biases in Natural Language Processing Models: A Conceptual Framework and Overview
Deven Santosh Shah, H. Andrew Schwartz, Dirk Hovy,
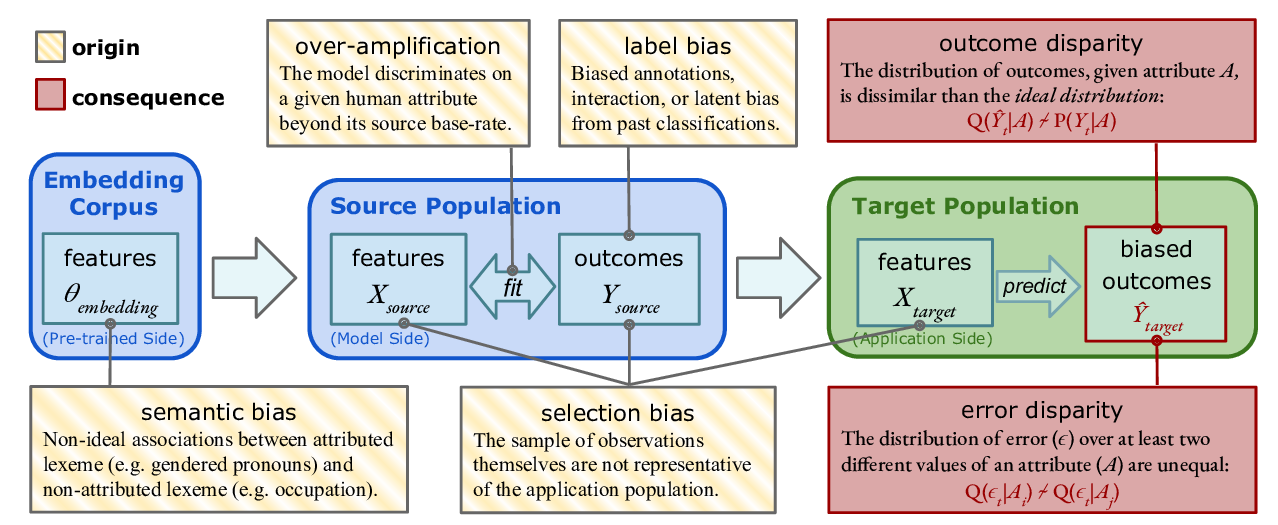