Rationalizing Medical Relation Prediction from Corpus-level Statistics
Zhen Wang, Jennifer Lee, Simon Lin, Huan Sun
Information Extraction Long Paper
Session 14A: Jul 8
(17:00-18:00 GMT)
Session 15B: Jul 8
(21:00-22:00 GMT)
Abstract:
Nowadays, the interpretability of machine learning models is becoming increasingly important, especially in the medical domain. Aiming to shed some light on how to rationalize medical relation prediction, we present a new interpretable framework inspired by existing theories on how human memory works, e.g., theories of recall and recognition. Given the corpus-level statistics, i.e., a global co-occurrence graph of a clinical text corpus, to predict the relations between two entities, we first recall rich contexts associated with the target entities, and then recognize relational interactions between these contexts to form model rationales, which will contribute to the final prediction. We conduct experiments on a real-world public clinical dataset and show that our framework can not only achieve competitive predictive performance against a comprehensive list of neural baseline models, but also present rationales to justify its prediction. We further collaborate with medical experts deeply to verify the usefulness of our model rationales for clinical decision making.
You can open the
pre-recorded video
in a separate window.
NOTE: The SlidesLive video may display a random order of the authors.
The correct author list is shown at the top of this webpage.
Similar Papers
Clinical-Coder: Assigning Interpretable ICD-10 Codes to Chinese Clinical Notes
Pengfei Cao, Chenwei Yan, Xiangling Fu, Yubo Chen, Kang Liu, Jun Zhao, Shengping Liu, Weifeng Chong,
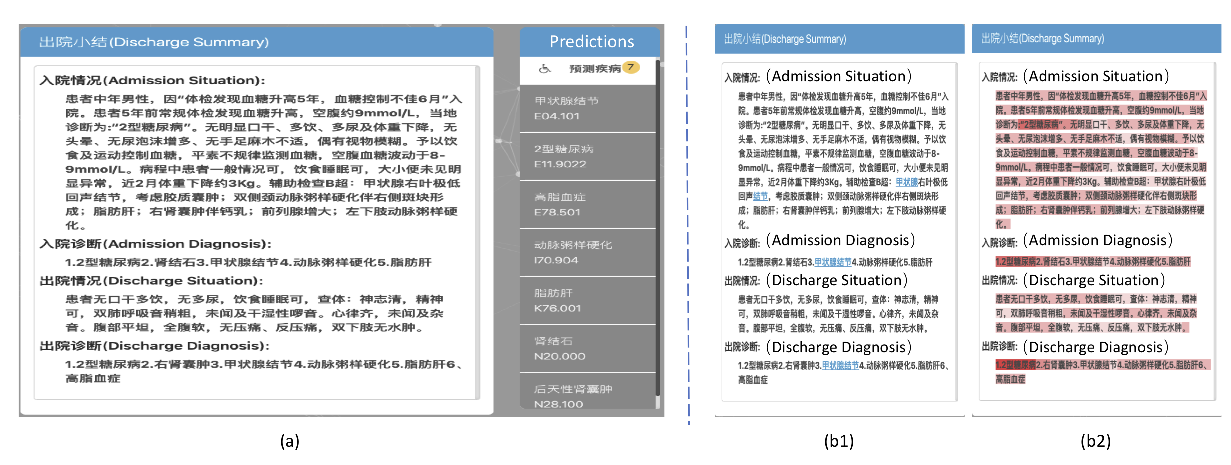
ERASER: A Benchmark to Evaluate Rationalized NLP Models
Jay DeYoung, Sarthak Jain, Nazneen Fatema Rajani, Eric Lehman, Caiming Xiong, Richard Socher, Byron C. Wallace,
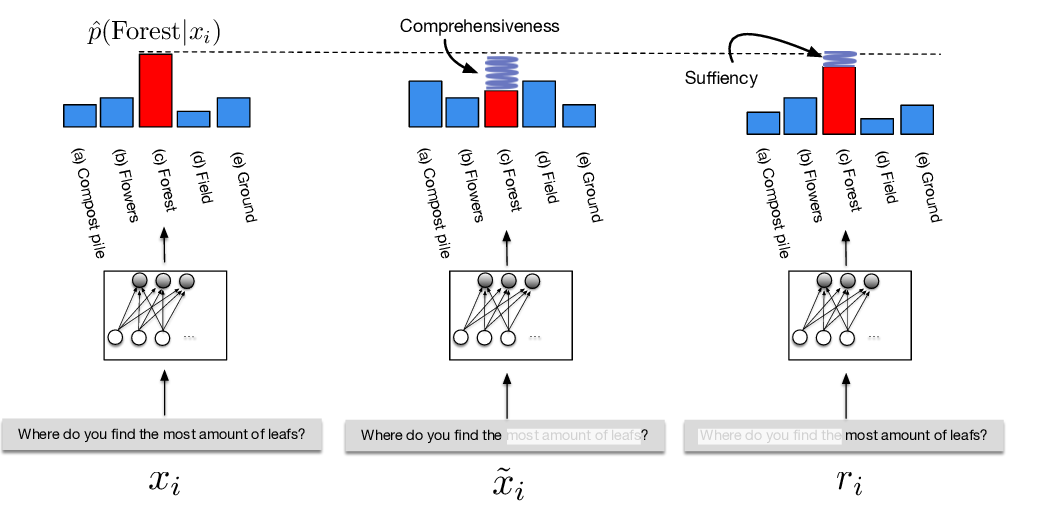
MIE: A Medical Information Extractor towards Medical Dialogues
Yuanzhe Zhang, Zhongtao Jiang, Tao Zhang, Shiwan Liu, Jiarun Cao, Kang Liu, Shengping Liu, Jun Zhao,
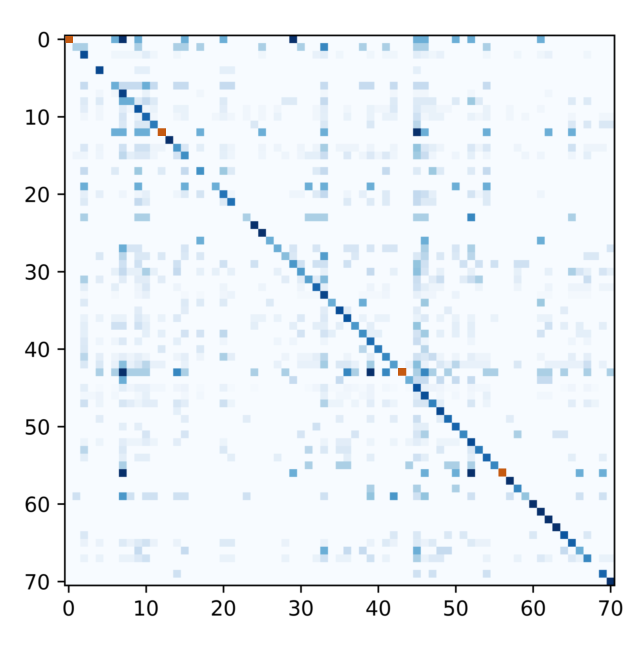
Towards Interpretable Clinical Diagnosis with Bayesian Network Ensembles Stacked on Entity-Aware CNNs
Jun Chen, Xiaoya Dai, Quan Yuan, Chao Lu, Haifeng Huang,
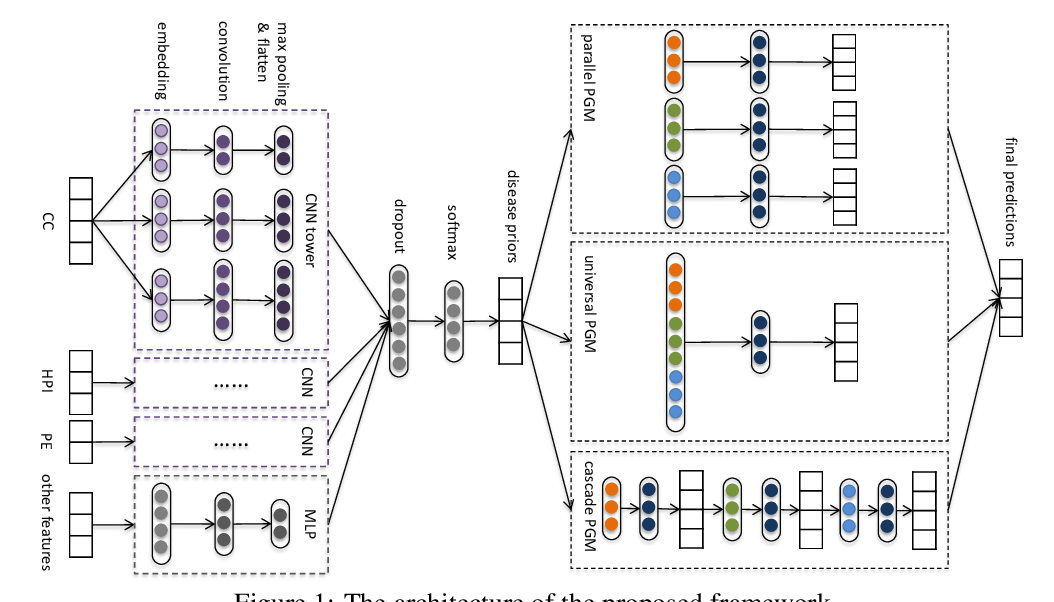