ERASER: A Benchmark to Evaluate Rationalized NLP Models
Jay DeYoung, Sarthak Jain, Nazneen Fatema Rajani, Eric Lehman, Caiming Xiong, Richard Socher, Byron C. Wallace
Interpretability and Analysis of Models for NLP Long Paper
Session 8A: Jul 7
(12:00-13:00 GMT)
Session 10A: Jul 7
(20:00-21:00 GMT)
Abstract:
State-of-the-art models in NLP are now predominantly based on deep neural networks that are opaque in terms of how they come to make predictions. This limitation has increased interest in designing more interpretable deep models for NLP that reveal the `reasoning' behind model outputs. But work in this direction has been conducted on different datasets and tasks with correspondingly unique aims and metrics; this makes it difficult to track progress. We propose the Evaluating Rationales And Simple English Reasoning (ERASER\, a benchmark to advance research on interpretable models in NLP. This benchmark comprises multiple datasets and tasks for which human annotations of ``rationales'' (supporting evidence) have been collected. We propose several metrics that aim to capture how well the rationales provided by models align with human rationales, and also how faithful these rationales are (i.e., the degree to which provided rationales influenced the corresponding predictions). Our hope is that releasing this benchmark facilitates progress on designing more interpretable NLP systems. The benchmark, code, and documentation are available at https://www.eraserbenchmark.com/
You can open the
pre-recorded video
in a separate window.
NOTE: The SlidesLive video may display a random order of the authors.
The correct author list is shown at the top of this webpage.
Similar Papers
Learning to Faithfully Rationalize by Construction
Sarthak Jain, Sarah Wiegreffe, Yuval Pinter, Byron C. Wallace,
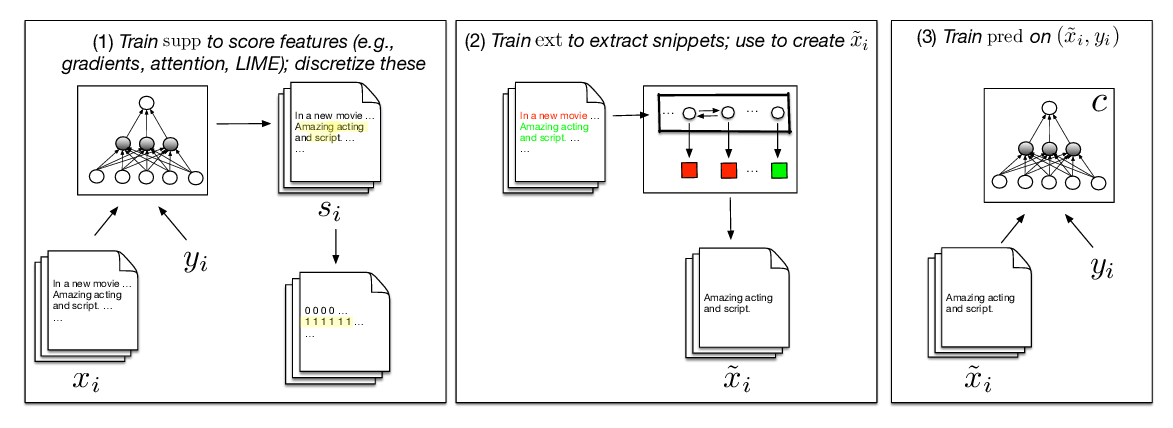
Predicting Performance for Natural Language Processing Tasks
Mengzhou Xia, Antonios Anastasopoulos, Ruochen Xu, Yiming Yang, Graham Neubig,
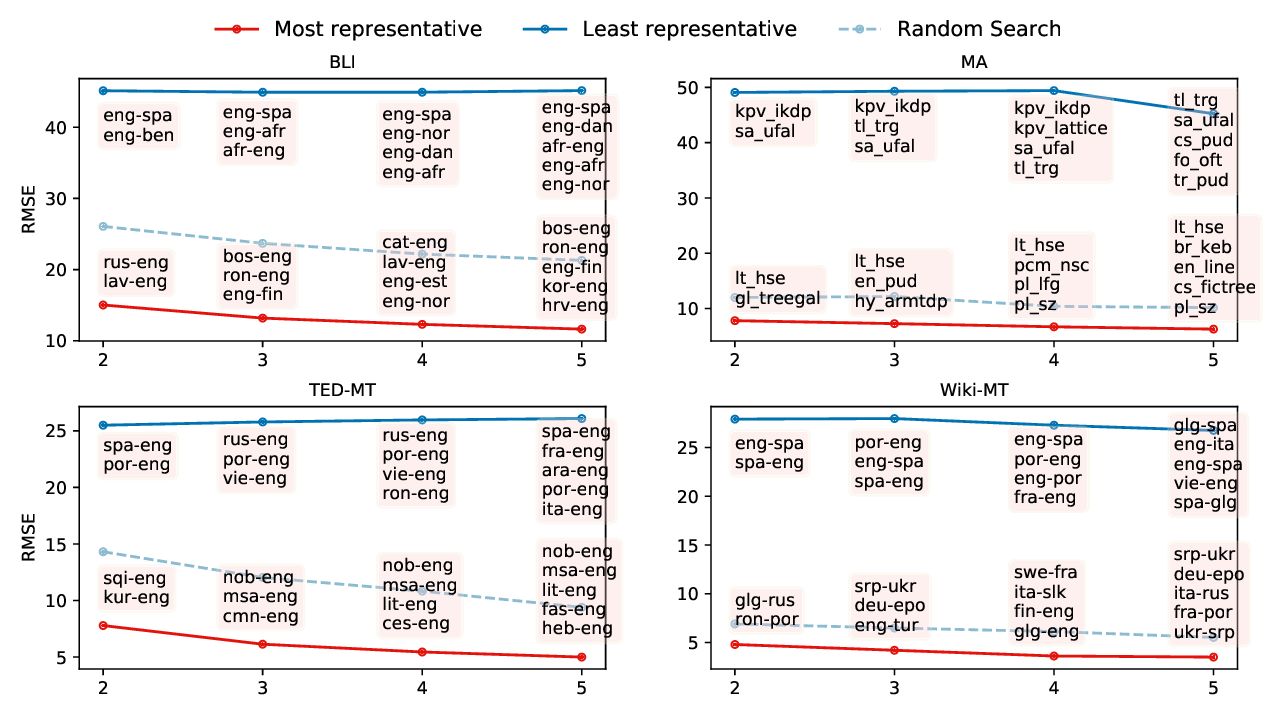
PuzzLing Machines: A Challenge on Learning From Small Data
Gözde Gül Şahin, Yova Kementchedjhieva, Phillip Rust, Iryna Gurevych,
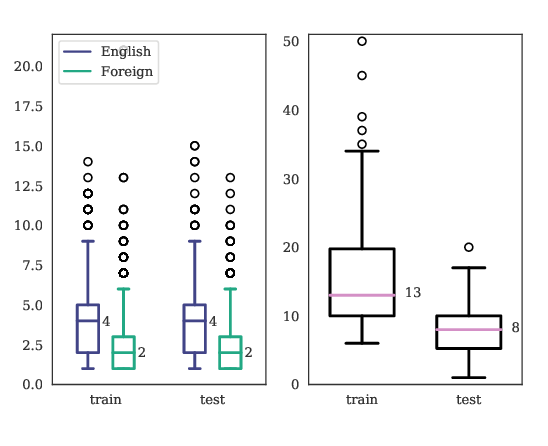
Towards Transparent and Explainable Attention Models
Akash Kumar Mohankumar, Preksha Nema, Sharan Narasimhan, Mitesh M. Khapra, Balaji Vasan Srinivasan, Balaraman Ravindran,
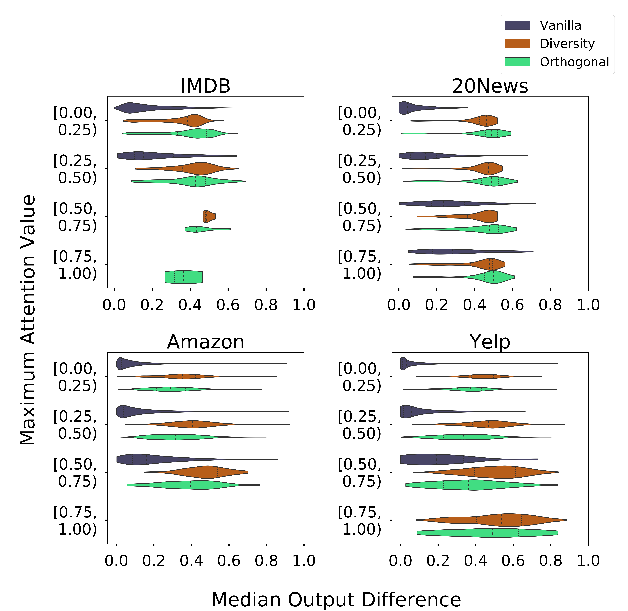