SpellGCN: Incorporating Phonological and Visual Similarities into Language Models for Chinese Spelling Check
Xingyi Cheng, Weidi Xu, Kunlong Chen, Shaohua Jiang, Feng Wang, Taifeng Wang, Wei Chu, Yuan Qi
NLP Applications Long Paper
Session 1B: Jul 6
(06:00-07:00 GMT)
Session 2B: Jul 6
(09:00-10:00 GMT)
Abstract:
Chinese Spelling Check (CSC) is a task to detect and correct spelling errors in Chinese natural language. Existing methods have made attempts to incorporate the similarity knowledge between Chinese characters. However, they take the similarity knowledge as either an external input resource or just heuristic rules. This paper proposes to incorporate phonological and visual similarity knowledge into language models for CSC via a specialized graph convolutional network (SpellGCN). The model builds a graph over the characters, and SpellGCN is learned to map this graph into a set of inter-dependent character classifiers. These classifiers are applied to the representations extracted by another network, such as BERT, enabling the whole network to be end-to-end trainable. Experiments are conducted on three human-annotated datasets. Our method achieves superior performance against previous models by a large margin.
You can open the
pre-recorded video
in a separate window.
NOTE: The SlidesLive video may display a random order of the authors.
The correct author list is shown at the top of this webpage.
Similar Papers
Line Graph Enhanced AMR-to-Text Generation with Mix-Order Graph Attention Networks
Yanbin Zhao, Lu Chen, Zhi Chen, Ruisheng Cao, Su Zhu, Kai Yu,
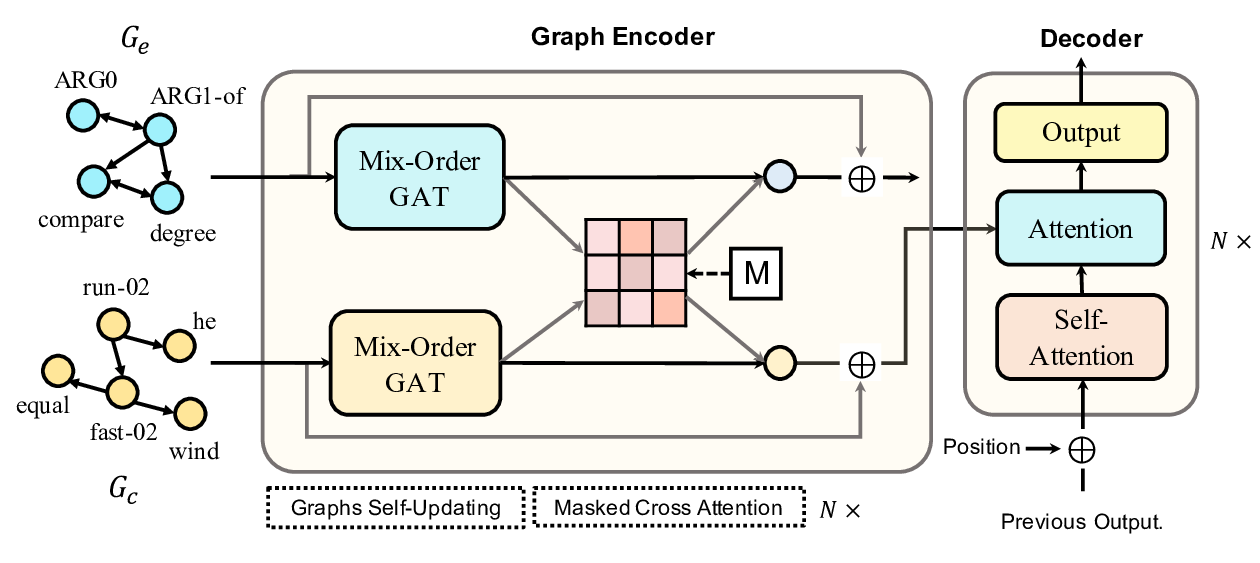
Neural Graph Matching Networks for Chinese Short Text Matching
Lu Chen, Yanbin Zhao, Boer Lv, Lesheng Jin, Zhi Chen, Su Zhu, Kai Yu,
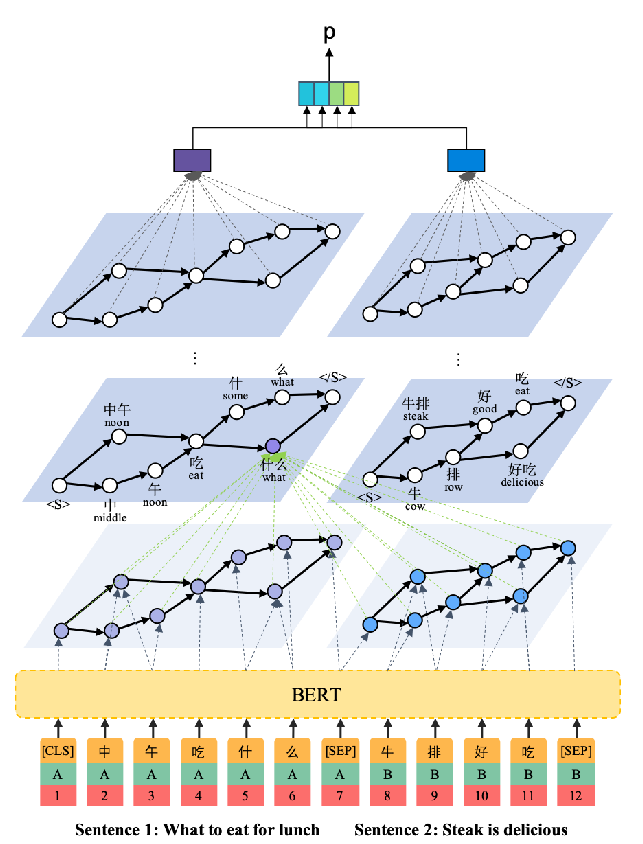
Enhancing Cross-target Stance Detection with Transferable Semantic-Emotion Knowledge
Bowen Zhang, Min Yang, Xutao Li, Yunming Ye, Xiaofei Xu, Kuai Dai,
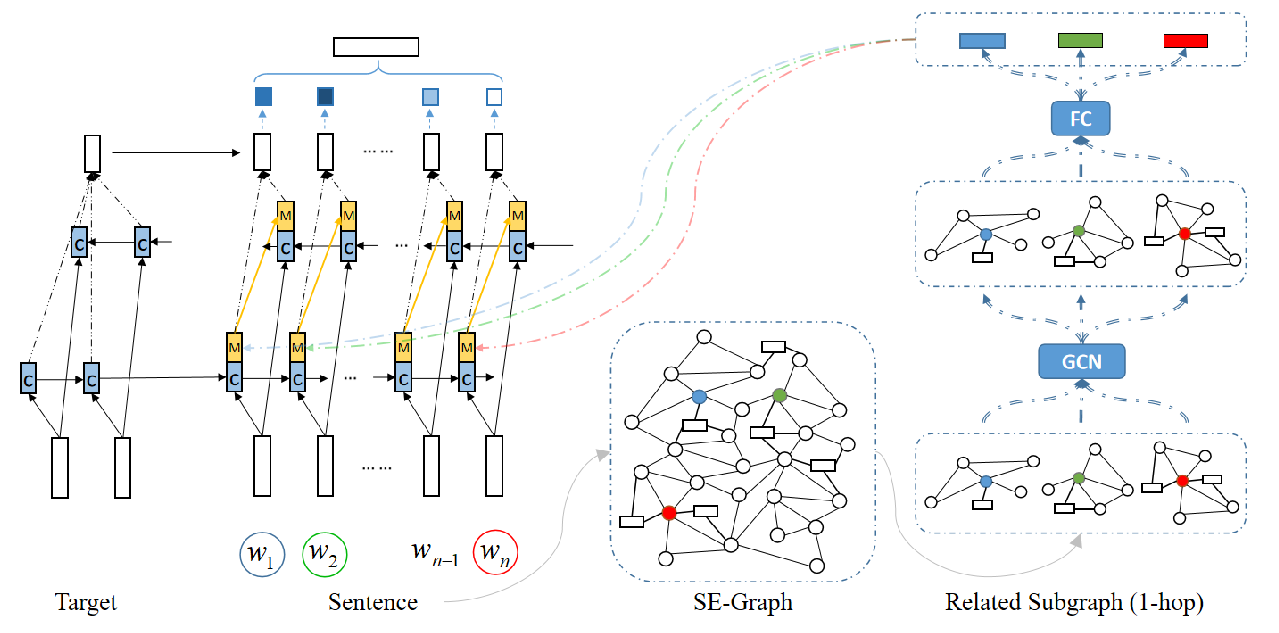
Don't Eclipse Your Arts Due to Small Discrepancies: Boundary Repositioning with a Pointer Network for Aspect Extraction
Zhenkai Wei, Yu Hong, Bowei Zou, Meng Cheng, Jianmin Yao,
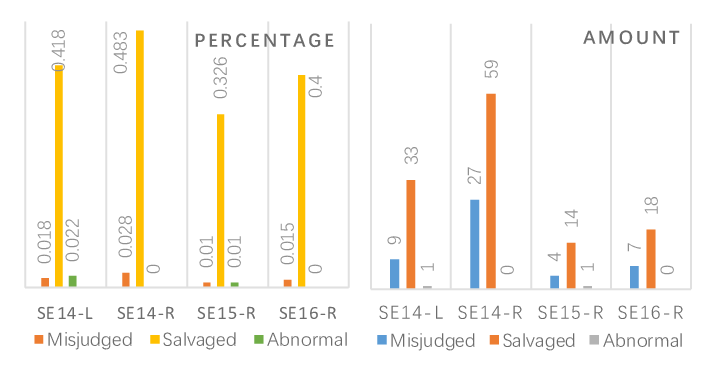