Enhancing Cross-target Stance Detection with Transferable Semantic-Emotion Knowledge
Bowen Zhang, Min Yang, Xutao Li, Yunming Ye, Xiaofei Xu, Kuai Dai
Sentiment Analysis, Stylistic Analysis, and Argument Mining Long Paper
Session 6A: Jul 7
(05:00-06:00 GMT)
Session 7A: Jul 7
(08:00-09:00 GMT)
Abstract:
Stance detection is an important task, which aims to classify the attitude of an opinionated text towards a given target. Remarkable success has been achieved when sufficient labeled training data is available. However, annotating sufficient data is labor-intensive, which establishes significant barriers for generalizing the stance classifier to the data with new targets. In this paper, we proposed a Semantic-Emotion Knowledge Transferring (SEKT) model for cross-target stance detection, which uses the external knowledge (semantic and emotion lexicons) as a bridge to enable knowledge transfer across different targets. Specifically, a semantic-emotion heterogeneous graph is constructed from external semantic and emotion lexicons, which is then fed into a graph convolutional network to learn multi-hop semantic connections between words and emotion tags. Then, the learned semantic-emotion graph representation, which serves as prior knowledge bridging the gap between the source and target domains, is fully integrated into the bidirectional long short-term memory (BiLSTM) stance classifier by adding a novel knowledge-aware memory unit to the BiLSTM cell. Extensive experiments on a large real-world dataset demonstrate the superiority of SEKT against the state-of-the-art baseline methods.
You can open the
pre-recorded video
in a separate window.
NOTE: The SlidesLive video may display a random order of the authors.
The correct author list is shown at the top of this webpage.
Similar Papers
Dependency Graph Enhanced Dual-transformer Structure for Aspect-based Sentiment Classification
Hao Tang, Donghong Ji, Chenliang Li, Qiji Zhou,
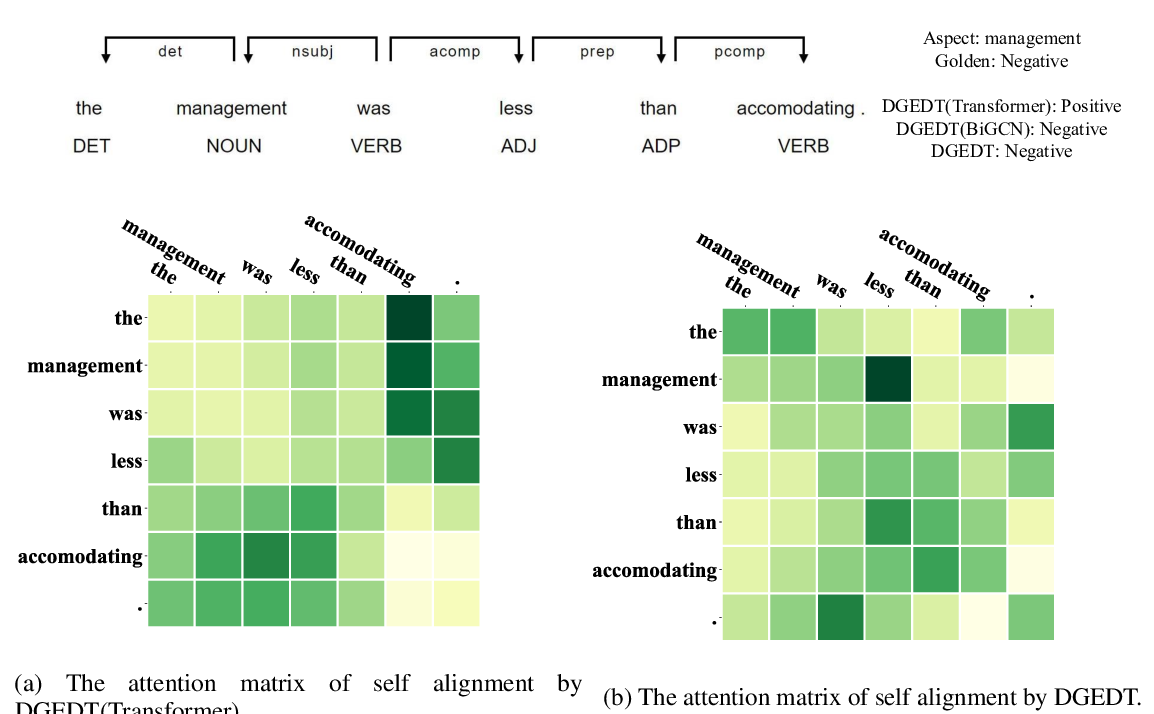
Will-They-Won't-They: A Very Large Dataset for Stance Detection on Twitter
Costanza Conforti, Jakob Berndt, Mohammad Taher Pilehvar, Chryssi Giannitsarou, Flavio Toxvaerd, Nigel Collier,
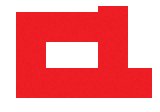
Dynamic Fusion Network for Multi-Domain End-to-end Task-Oriented Dialog
Libo Qin, Xiao Xu, Wanxiang Che, Yue Zhang, Ting Liu,
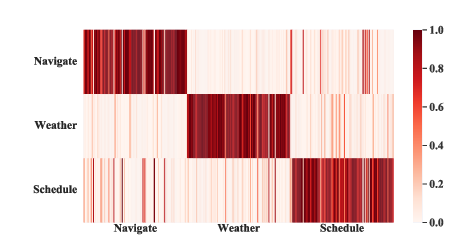
Learning to Tag OOV Tokens by Integrating Contextual Representation and Background Knowledge
Keqing He, Yuanmeng Yan, Weiran XU,
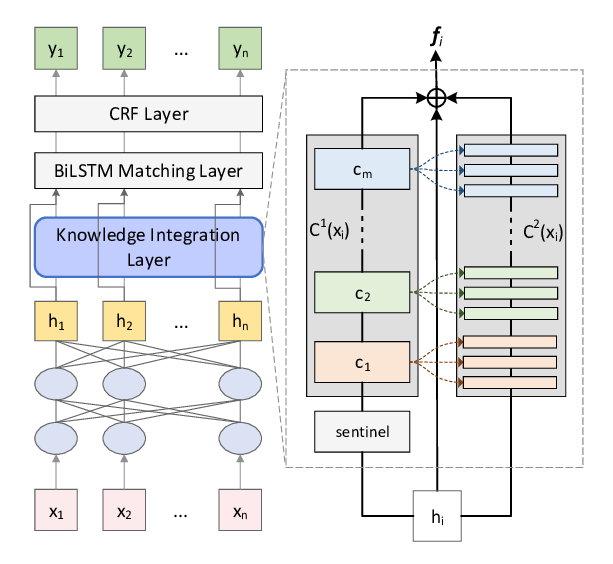