A Geometry-Inspired Attack for Generating Natural Language Adversarial Examples
Zhao Meng, Roger Wattenhofer
Student Research Workshop SRW Paper
Session 4B: Jul 6
(18:00-19:00 GMT)
Session 14B: Jul 8
(18:00-19:00 GMT)
Abstract:
Generating adversarial examples for natural language is hard, as natural language consists of discrete symbols and examples are often of variable lengths. In this paper, we propose a geometry-inspired attack for generating natural language adversarial examples. Our attack generates adversarial examples by iteratively approximating the decision boundary of deep neural networks. Experiments on two datasets with two different models show that our attack fools the models with high success rates, while only replacing a few words. Human evaluation shows that adversarial examples generated by our attack are hard for humans to recognize. Further experiments show that adversarial training can improve model robustness against our attack.
You can open the
pre-recorded video
in a separate window.
NOTE: The SlidesLive video may display a random order of the authors.
The correct author list is shown at the top of this webpage.
Similar Papers
Word-level Textual Adversarial Attacking as Combinatorial Optimization
Yuan Zang, Fanchao Qi, Chenghao Yang, Zhiyuan Liu, Meng Zhang, Qun Liu, Maosong Sun,
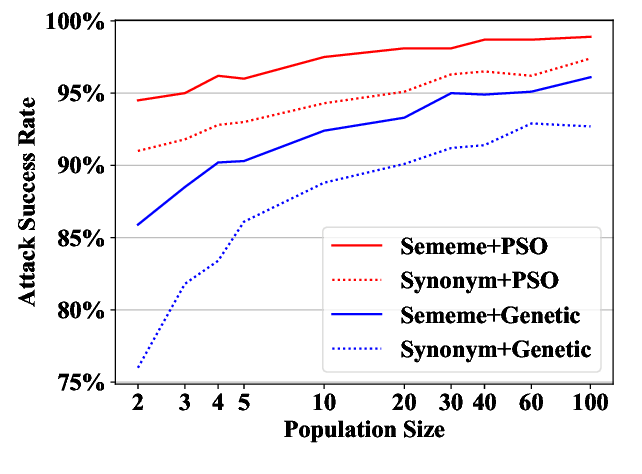
A Reinforced Generation of Adversarial Examples for Neural Machine Translation
Wei Zou, Shujian Huang, Jun Xie, Xinyu Dai, Jiajun Chen,
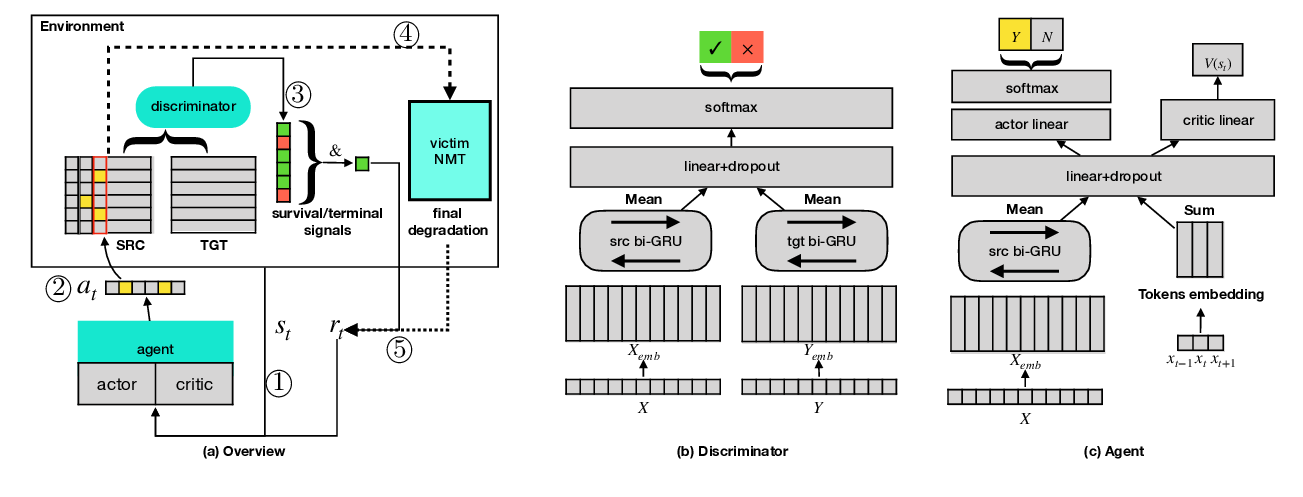
Evaluating and Enhancing the Robustness of Neural Network-based Dependency Parsing Models with Adversarial Examples
Xiaoqing Zheng, Jiehang Zeng, Yi Zhou, Cho-Jui Hsieh, Minhao Cheng, Xuanjing Huang,
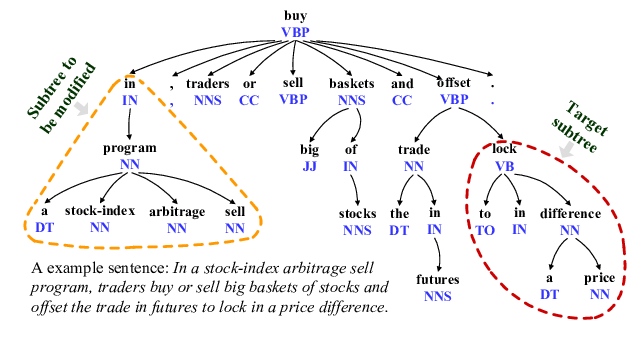
Robust Encodings: A Framework for Combating Adversarial Typos
Erik Jones, Robin Jia, Aditi Raghunathan, Percy Liang,
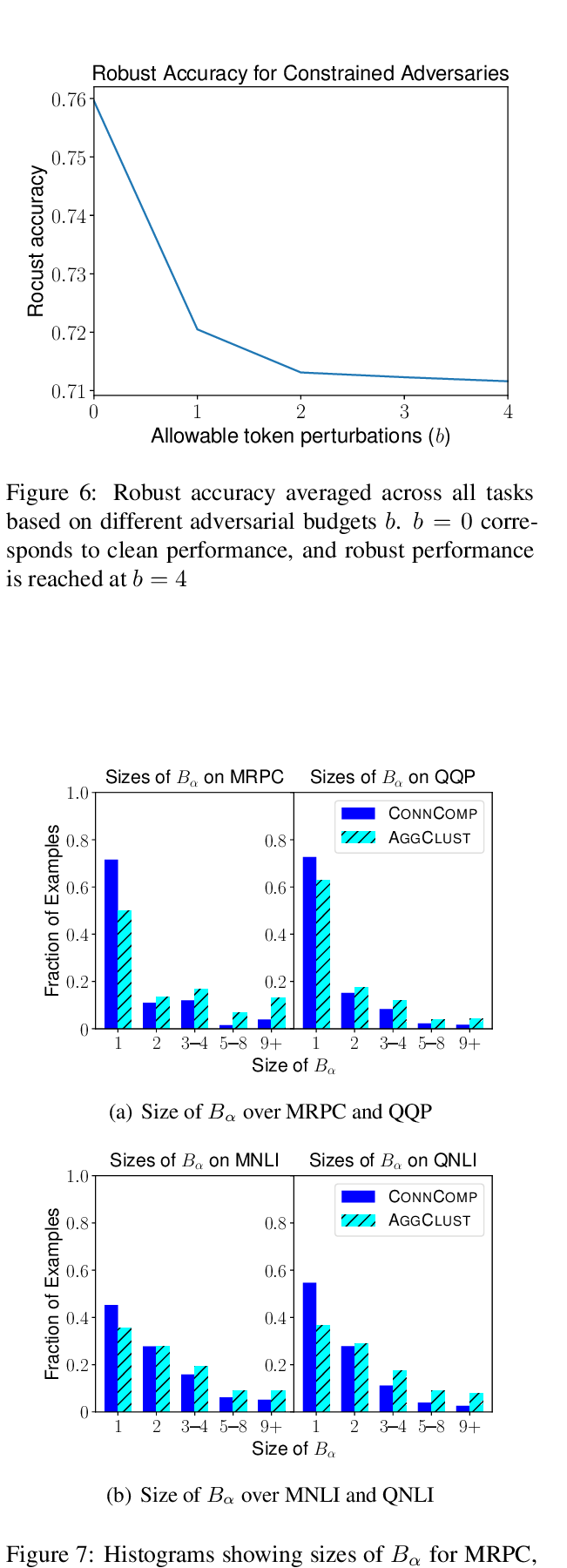