Evaluating and Enhancing the Robustness of Neural Network-based Dependency Parsing Models with Adversarial Examples
Xiaoqing Zheng, Jiehang Zeng, Yi Zhou, Cho-Jui Hsieh, Minhao Cheng, Xuanjing Huang
Machine Learning for NLP Long Paper
Session 11B: Jul 8
(06:00-07:00 GMT)
Session 12B: Jul 8
(09:00-10:00 GMT)
Abstract:
Despite achieving prominent performance on many important tasks, it has been reported that neural networks are vulnerable to adversarial examples. Previously studies along this line mainly focused on semantic tasks such as sentiment analysis, question answering and reading comprehension. In this study, we show that adversarial examples also exist in dependency parsing: we propose two approaches to study where and how parsers make mistakes by searching over perturbations to existing texts at sentence and phrase levels, and design algorithms to construct such examples in both of the black-box and white-box settings. Our experiments with one of state-of-the-art parsers on the English Penn Treebank (PTB) show that up to 77% of input examples admit adversarial perturbations, and we also show that the robustness of parsing models can be improved by crafting high-quality adversaries and including them in the training stage, while suffering little to no performance drop on the clean input data.
You can open the
pre-recorded video
in a separate window.
NOTE: The SlidesLive video may display a random order of the authors.
The correct author list is shown at the top of this webpage.
Similar Papers
A Geometry-Inspired Attack for Generating Natural Language Adversarial Examples
Zhao Meng, Roger Wattenhofer,
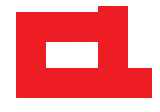
Word-level Textual Adversarial Attacking as Combinatorial Optimization
Yuan Zang, Fanchao Qi, Chenghao Yang, Zhiyuan Liu, Meng Zhang, Qun Liu, Maosong Sun,
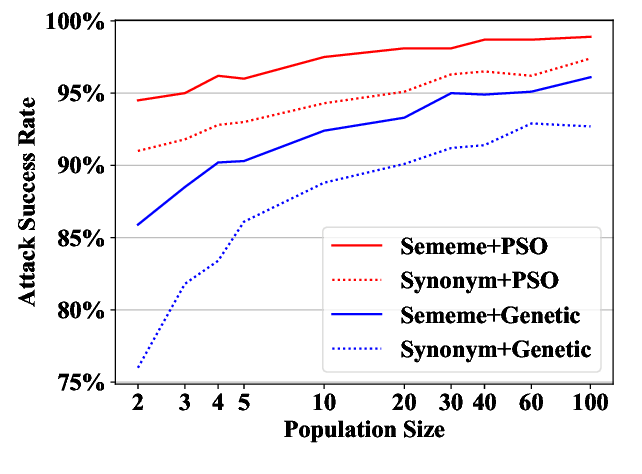
GAN-BERT: Generative Adversarial Learning for Robust Text Classification with a Bunch of Labeled Examples
Danilo Croce, Giuseppe Castellucci, Roberto Basili,
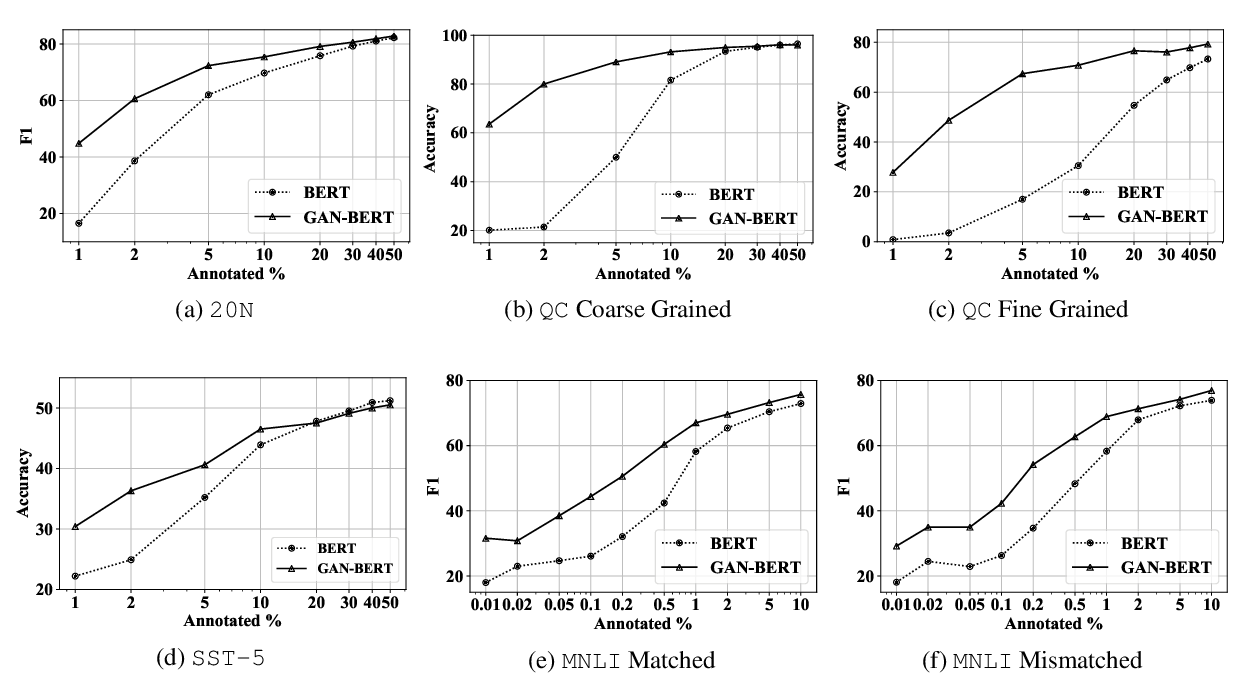