Label Noise in Context
Michael Desmond, Catherine Finegan-Dollak, Jeff Boston, Matt Arnold
System Demonstrations Demo Paper
Demo Session 3B-2: Jul 7
(12:45-13:45 GMT)
Demo Session 5A-2: Jul 7
(20:00-21:00 GMT)
Abstract:
Label noise—incorrectly or ambiguously labeled training examples—can negatively impact model performance. Although noise detection techniques have been around for decades, practitioners rarely apply them, as manual noise remediation is a tedious process. Examples incorrectly flagged as noise waste reviewers’ time, and correcting label noise without guidance can be difficult. We propose LNIC, a noise-detection method that uses an example’s neighborhood within the training set to (a) reduce false positives and (b) provide an explanation as to why the ex- ample was flagged as noise. We demonstrate on several short-text classification datasets that LNIC outperforms the state of the art on measures of precision and F0.5-score. We also show how LNIC’s training set context helps a reviewer to understand and correct label noise in a dataset. The LNIC tool lowers the barriers to label noise remediation, increasing its utility for NLP practitioners.
You can open the
pre-recorded video
in a separate window.
NOTE: The SlidesLive video may display a random order of the authors.
The correct author list is shown at the top of this webpage.
Similar Papers
Noise-Based Augmentation Techniques for Emotion Datasets: What do we Recommend?
Mimansa Jaiswal, Emily Mower Provost,
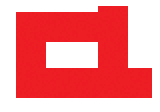
NAT: Noise-Aware Training for Robust Neural Sequence Labeling
Marcin Namysl, Sven Behnke, Joachim Köhler,
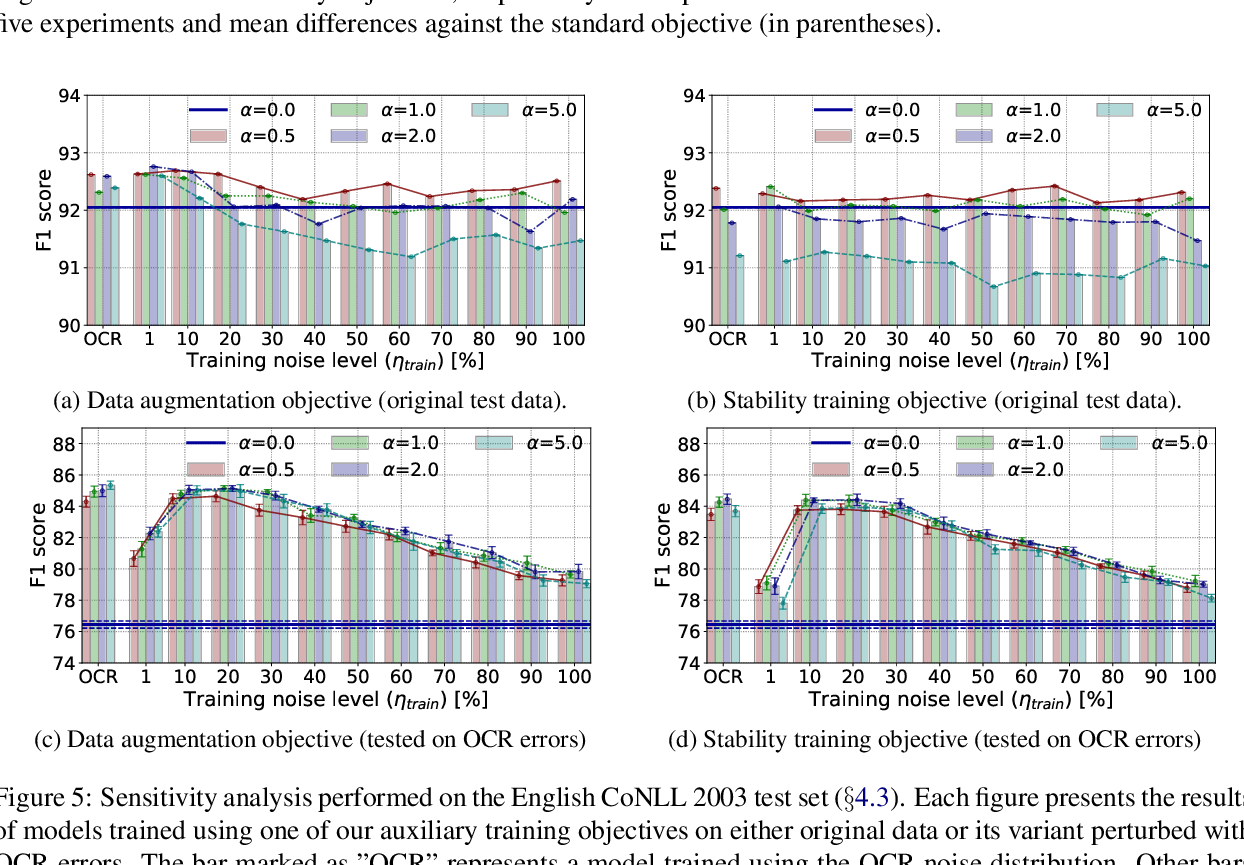
Does Multi-Encoder Help? A Case Study on Context-Aware Neural Machine Translation
Bei Li, Hui Liu, Ziyang Wang, Yufan Jiang, Tong Xiao, Jingbo Zhu, Tongran Liu, Changliang Li,
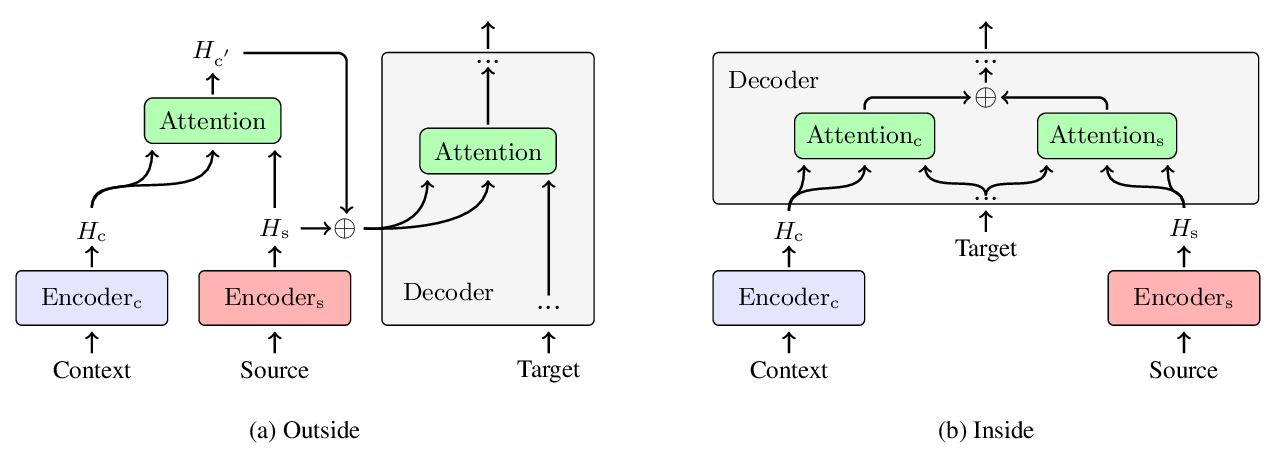
LEAN-LIFE: A Label-Efficient Annotation Framework Towards Learning from Explanation
Dong-Ho Lee, Rahul Khanna, Bill Yuchen Lin, Seyeon Lee, Qinyuan Ye, Elizabeth Boschee, Leonardo Neves, Xiang Ren,
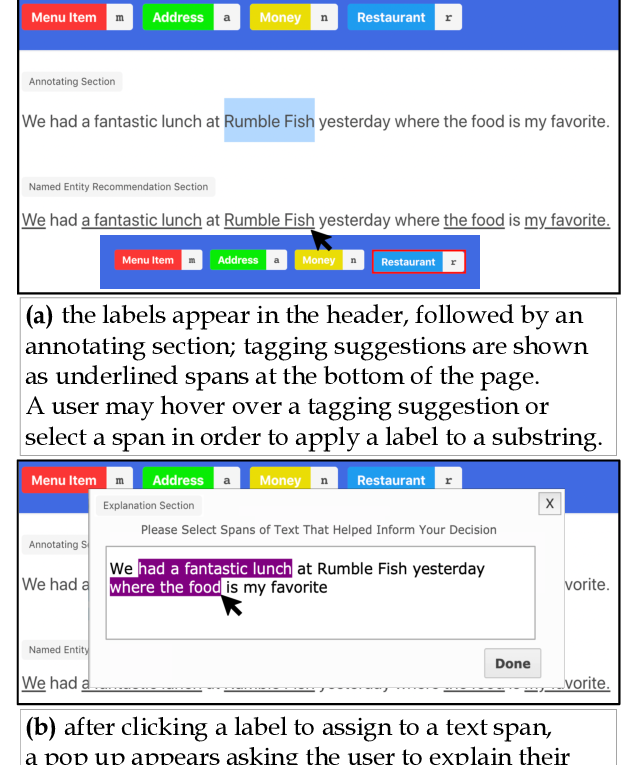