Does Multi-Encoder Help? A Case Study on Context-Aware Neural Machine Translation
Bei Li, Hui Liu, Ziyang Wang, Yufan Jiang, Tong Xiao, Jingbo Zhu, Tongran Liu, Changliang Li
Machine Translation Short Paper
Session 6B: Jul 7
(06:00-07:00 GMT)
Session 7B: Jul 7
(09:00-10:00 GMT)
Abstract:
In encoder-decoder neural models, multiple encoders are in general used to represent the contextual information in addition to the individual sentence. In this paper, we investigate multi-encoder approaches in document-level neural machine translation (NMT). Surprisingly, we find that the context encoder does not only encode the surrounding sentences but also behaves as a noise generator. This makes us rethink the real benefits of multi-encoder in context-aware translation - some of the improvements come from robust training. We compare several methods that introduce noise and/or well-tuned dropout setup into the training of these encoders. Experimental results show that noisy training plays an important role in multi-encoder-based NMT, especially when the training data is small. Also, we establish a new state-of-the-art on IWSLT Fr-En task by careful use of noise generation and dropout methods.
You can open the
pre-recorded video
in a separate window.
NOTE: The SlidesLive video may display a random order of the authors.
The correct author list is shown at the top of this webpage.
Similar Papers
A Simple and Effective Unified Encoder for Document-Level Machine Translation
Shuming Ma, Dongdong Zhang, Ming Zhou,
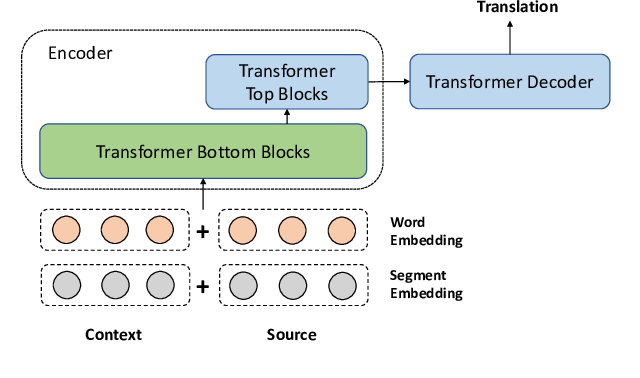
Using Context in Neural Machine Translation Training Objectives
Danielle Saunders, Felix Stahlberg, Bill Byrne,
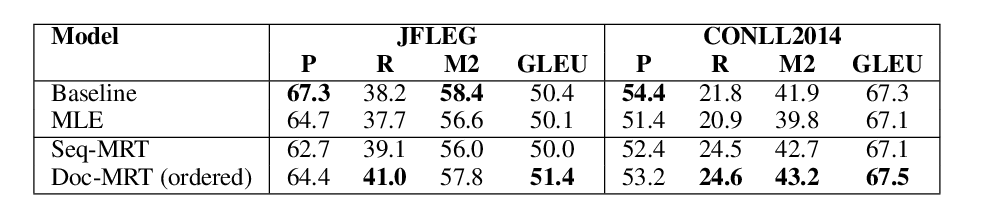
Noise-Based Augmentation Techniques for Emotion Datasets: What do we Recommend?
Mimansa Jaiswal, Emily Mower Provost,
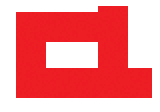
A Novel Graph-based Multi-modal Fusion Encoder for Neural Machine Translation
Yongjing Yin, Fandong Meng, Jinsong Su, Chulun Zhou, Zhengyuan Yang, Jie Zhou, Jiebo Luo,
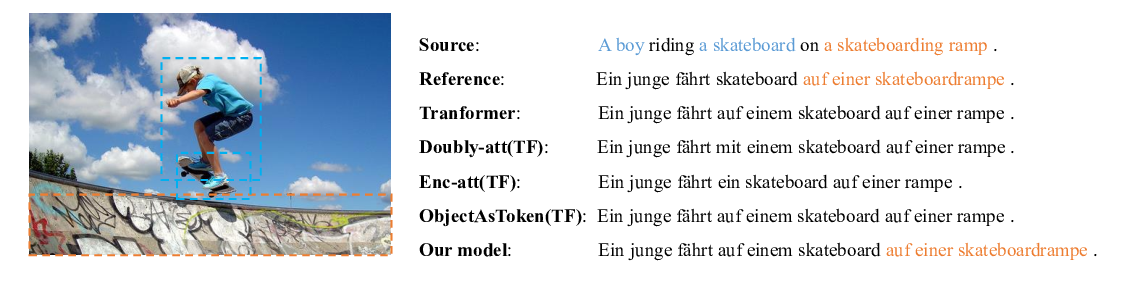