NAT: Noise-Aware Training for Robust Neural Sequence Labeling
Marcin Namysl, Sven Behnke, Joachim Köhler
Information Extraction Long Paper
Session 2B: Jul 6
(09:00-10:00 GMT / 09:00-10:00 GMT)
Session 3B: Jul 6
(13:00-14:00 GMT / 13:00-14:00 GMT)
Abstract:
Sequence labeling systems should perform reliably not only under ideal conditions but also with corrupted inputs---as these systems often process user-generated text or follow an error-prone upstream component. To this end, we formulate the noisy sequence labeling problem, where the input may undergo an unknown noising process and propose two Noise-Aware Training (NAT) objectives that improve robustness of sequence labeling performed on perturbed input: Our data augmentation method trains a neural model using a mixture of clean and noisy samples, whereas our stability training algorithm encourages the model to create a noise-invariant latent representation. We employ a vanilla noise model at training time. For evaluation, we use both the original data and its variants perturbed with real OCR errors and misspellings. Extensive experiments on English and German named entity recognition benchmarks confirmed that NAT consistently improved robustness of popular sequence labeling models, preserving accuracy on the original input. We make our code and data publicly available for the research community.
You can open the
pre-recorded video
in a separate window.
NOTE: The SlidesLive video may display a random order of the authors.
The correct author list is shown at the top of this webpage.
Similar Papers
Noise-Based Augmentation Techniques for Emotion Datasets: What do we Recommend?
Mimansa Jaiswal, Emily Mower Provost,
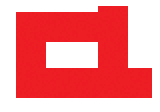
Does Multi-Encoder Help? A Case Study on Context-Aware Neural Machine Translation
Bei Li, Hui Liu, Ziyang Wang, Yufan Jiang, Tong Xiao, Jingbo Zhu, Tongran Liu, Changliang Li,
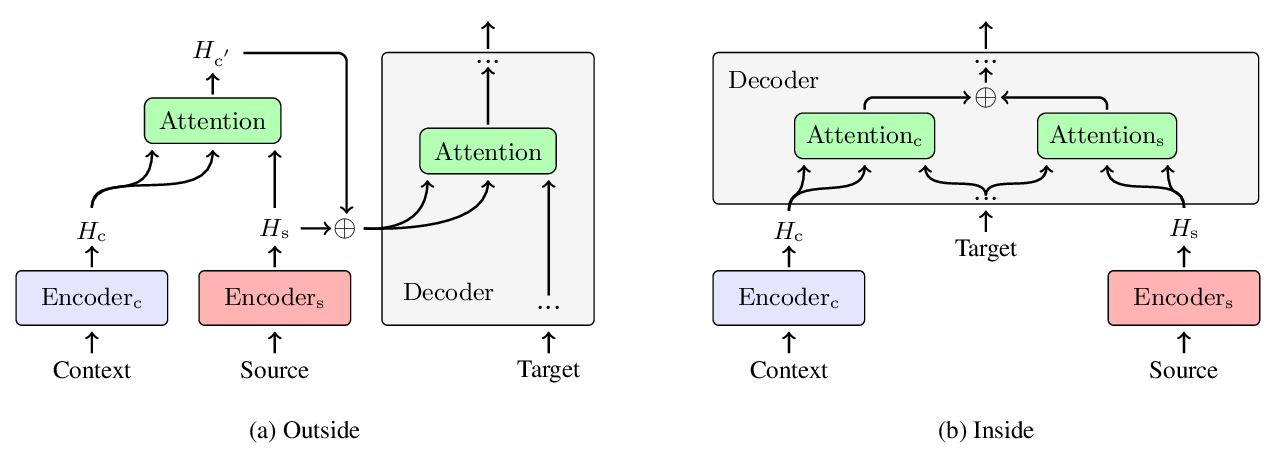
SeqVAT: Virtual Adversarial Training for Semi-Supervised Sequence Labeling
Luoxin Chen, Weitong Ruan, Xinyue Liu, Jianhua Lu,
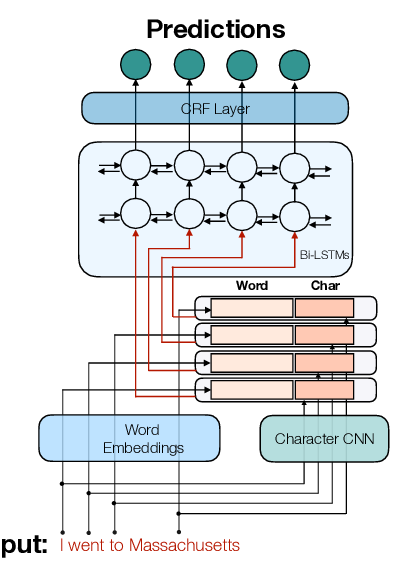