Towards Faithful Neural Table-to-Text Generation with Content-Matching Constraints
Zhenyi Wang, Xiaoyang Wang, Bang An, Dong Yu, Changyou Chen
Generation Long Paper
Session 2A: Jul 6
(08:00-09:00 GMT)
Session 3B: Jul 6
(13:00-14:00 GMT)
Abstract:
Text generation from a knowledge base aims to translate knowledge triples to natural language descriptions. Most existing methods ignore the faithfulness between a generated text description and the original table, leading to generated information that goes beyond the content of the table. In this paper, for the first time, we propose a novel Transformer-based generation framework to achieve the goal. The core techniques in our method to enforce faithfulness include a new table-text optimal-transport matching loss and a table-text embedding similarity loss based on the Transformer model. Furthermore, to evaluate faithfulness, we propose a new automatic metric specialized to the table-to-text generation problem. We also provide detailed analysis on each component of our model in our experiments. Automatic and human evaluations show that our framework can significantly outperform state-of-the-art by a large margin.
You can open the
pre-recorded video
in a separate window.
NOTE: The SlidesLive video may display a random order of the authors.
The correct author list is shown at the top of this webpage.
Similar Papers
FEQA: A Question Answering Evaluation Framework for Faithfulness Assessment in Abstractive Summarization
Esin Durmus, He He, Mona Diab,
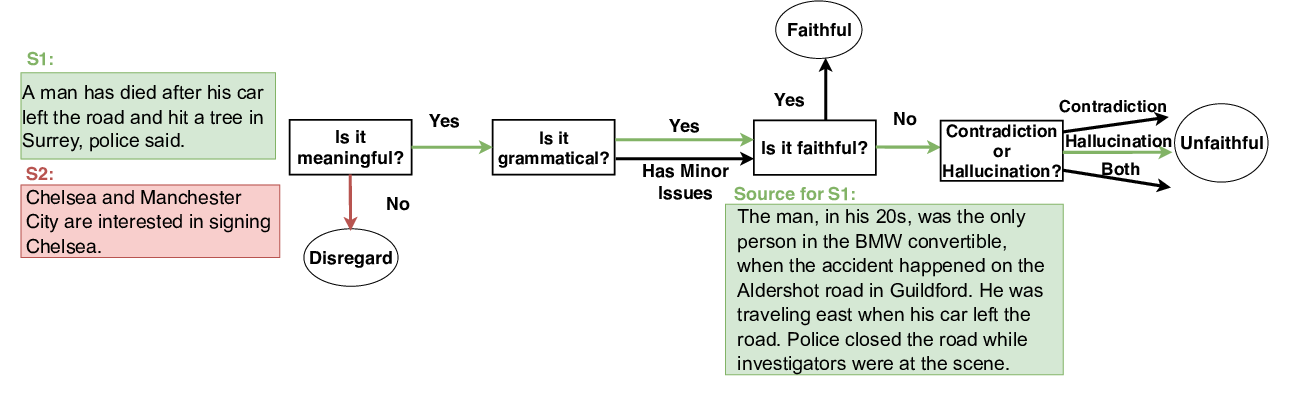
Structural Information Preserving for Graph-to-Text Generation
Linfeng Song, Ante Wang, Jinsong Su, Yue Zhang, Kun Xu, Yubin Ge, Dong Yu,
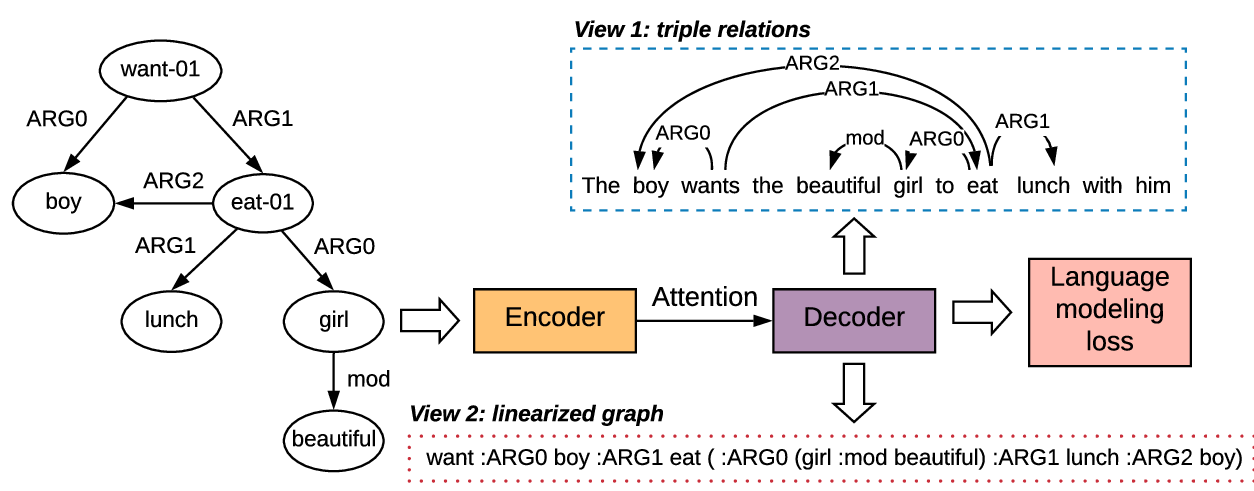
Two Birds, One Stone: A Simple, Unified Model for Text Generation from Structured and Unstructured Data
Hamidreza Shahidi, Ming Li, Jimmy Lin,
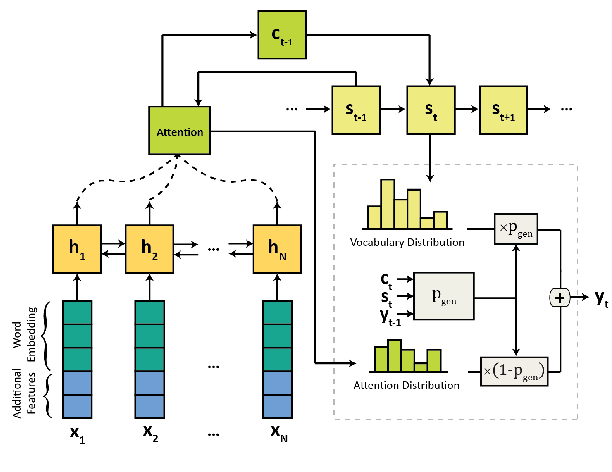
Hooks in the Headline: Learning to Generate Headlines with Controlled Styles
Di Jin, Zhijing Jin, Joey Tianyi Zhou, Lisa Orii, Peter Szolovits,
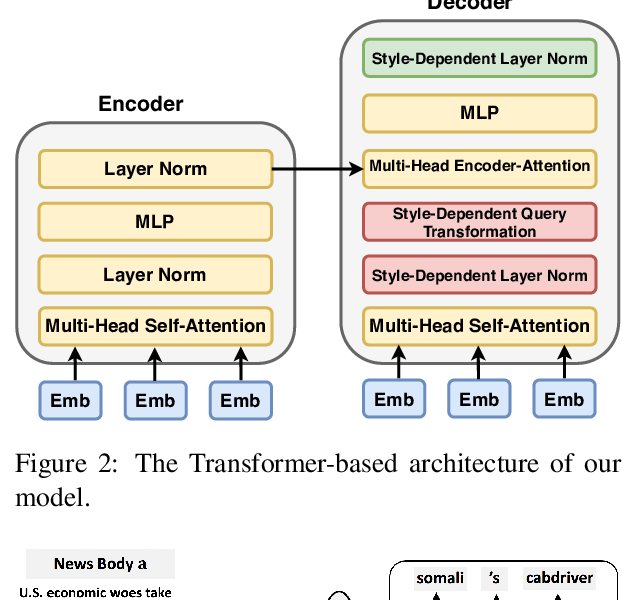