Structural Information Preserving for Graph-to-Text Generation
Linfeng Song, Ante Wang, Jinsong Su, Yue Zhang, Kun Xu, Yubin Ge, Dong Yu
Generation Long Paper
Session 14A: Jul 8
(17:00-18:00 GMT)
Session 15B: Jul 8
(21:00-22:00 GMT)
Abstract:
The task of graph-to-text generation aims at producing sentences that preserve the meaning of input graphs. As a crucial defect, the current state-of-the-art models may mess up or even drop the core structural information of input graphs when generating outputs. We propose to tackle this problem by leveraging richer training signals that can guide our model for preserving input information. In particular, we introduce two types of autoencoding losses, each individually focusing on different aspects (a.k.a. views) of input graphs. The losses are then back-propagated to better calibrate our model via multi-task training. Experiments on two benchmarks for graph-to-text generation show the effectiveness of our approach over a state-of-the-art baseline.
You can open the
pre-recorded video
in a separate window.
NOTE: The SlidesLive video may display a random order of the authors.
The correct author list is shown at the top of this webpage.
Similar Papers
Line Graph Enhanced AMR-to-Text Generation with Mix-Order Graph Attention Networks
Yanbin Zhao, Lu Chen, Zhi Chen, Ruisheng Cao, Su Zhu, Kai Yu,
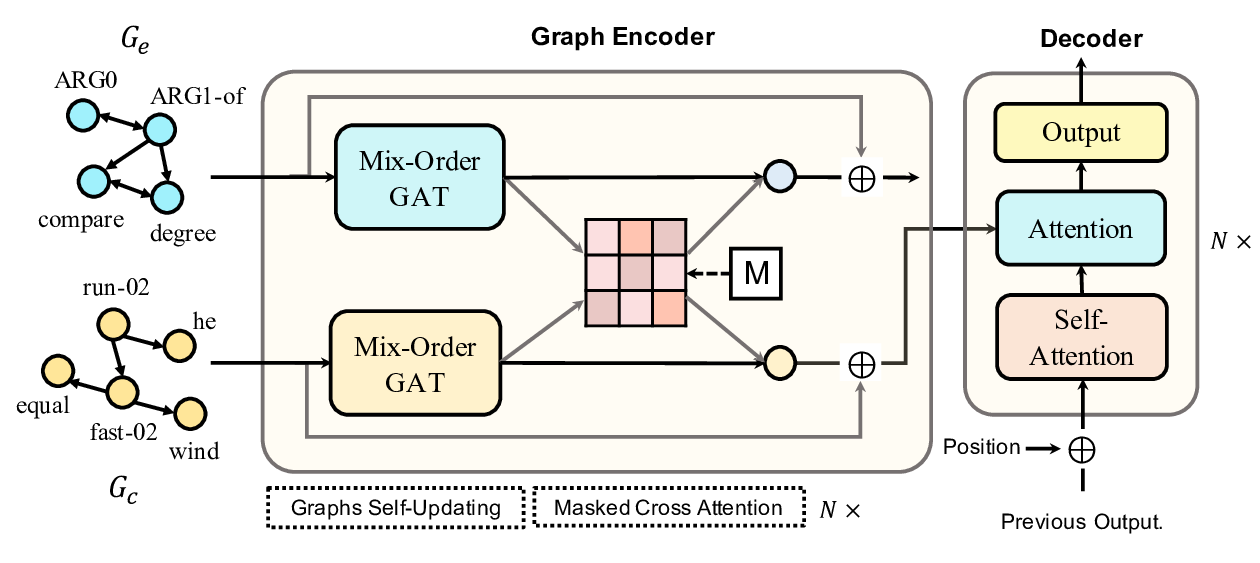
Bridging the Structural Gap Between Encoding and Decoding for Data-To-Text Generation
Chao Zhao, Marilyn Walker, Snigdha Chaturvedi,
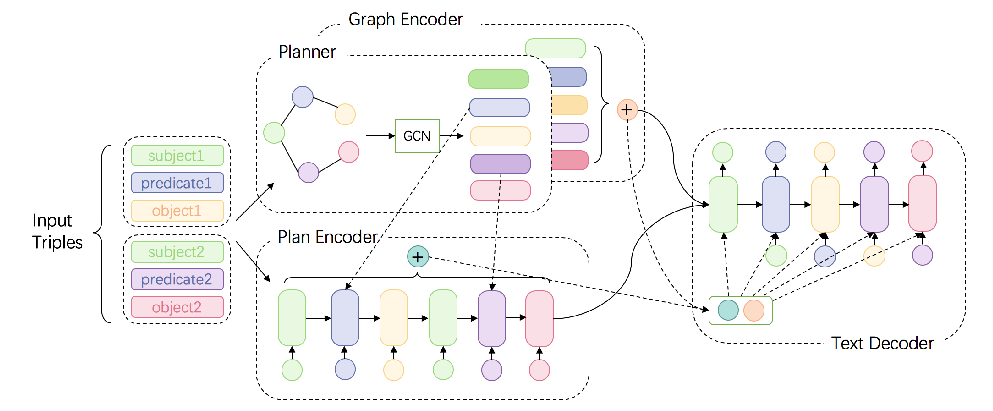
Heterogeneous Graph Transformer for Graph-to-Sequence Learning
Shaowei Yao, Tianming Wang, Xiaojun Wan,
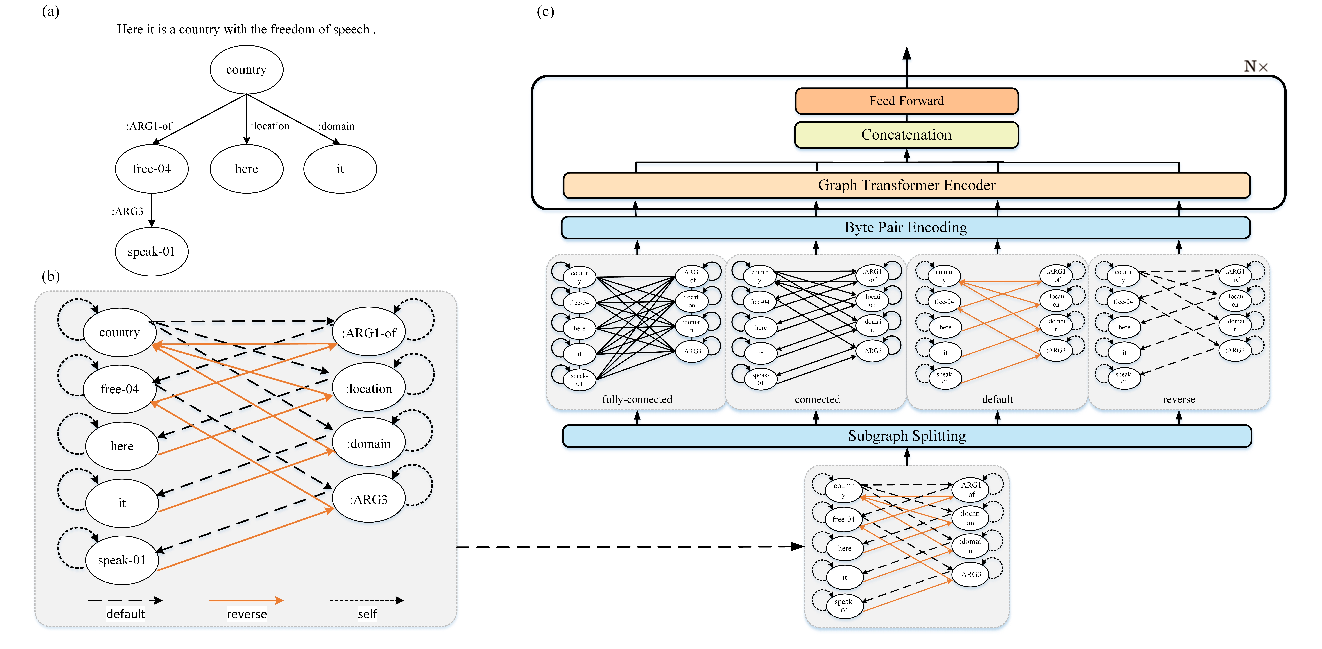