A Graph Auto-encoder Model of Derivational Morphology
Valentin Hofmann, Hinrich Schütze, Janet Pierrehumbert
Phonology, Morphology and Word Segmentation Long Paper
Session 2A: Jul 6
(08:00-09:00 GMT / 08:00-09:00 GMT)
Session 3B: Jul 6
(13:00-14:00 GMT / 13:00-14:00 GMT)
Abstract:
There has been little work on modeling the morphological well-formedness (MWF) of derivatives, a problem judged to be complex and difficult in linguistics. We present a graph auto-encoder that learns embeddings capturing information about the compatibility of affixes and stems in derivation. The auto-encoder models MWF in English surprisingly well by combining syntactic and semantic information with associative information from the mental lexicon.
You can open the
pre-recorded video
in a separate window.
NOTE: The SlidesLive video may display a random order of the authors.
The correct author list is shown at the top of this webpage.
Similar Papers
Line Graph Enhanced AMR-to-Text Generation with Mix-Order Graph Attention Networks
Yanbin Zhao, Lu Chen, Zhi Chen, Ruisheng Cao, Su Zhu, Kai Yu,
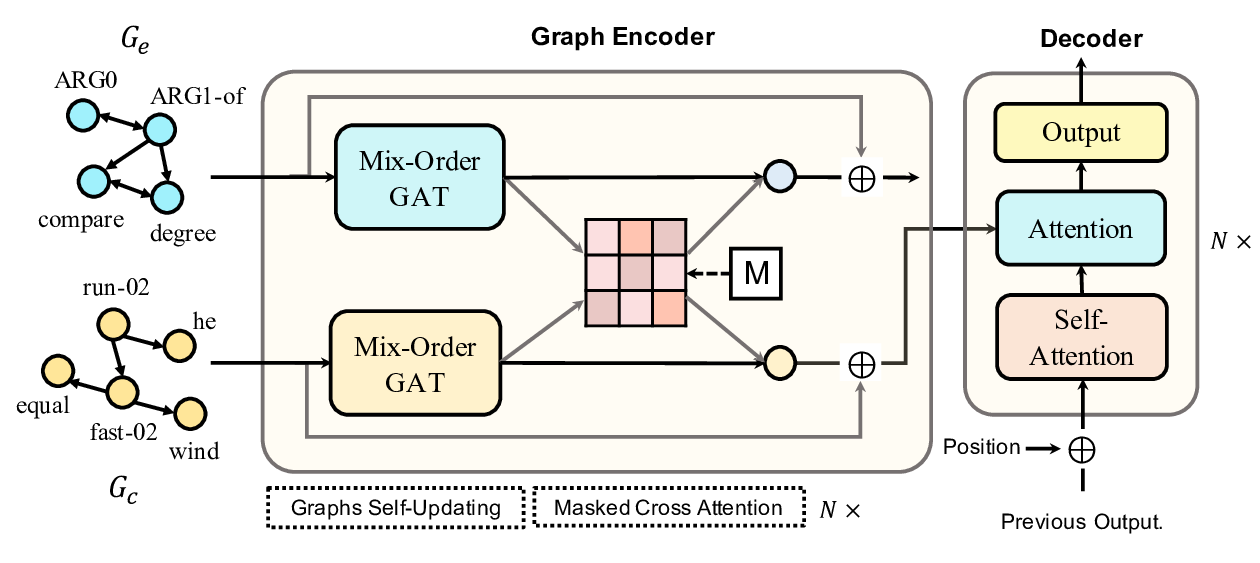
Does Multi-Encoder Help? A Case Study on Context-Aware Neural Machine Translation
Bei Li, Hui Liu, Ziyang Wang, Yufan Jiang, Tong Xiao, Jingbo Zhu, Tongran Liu, Changliang Li,
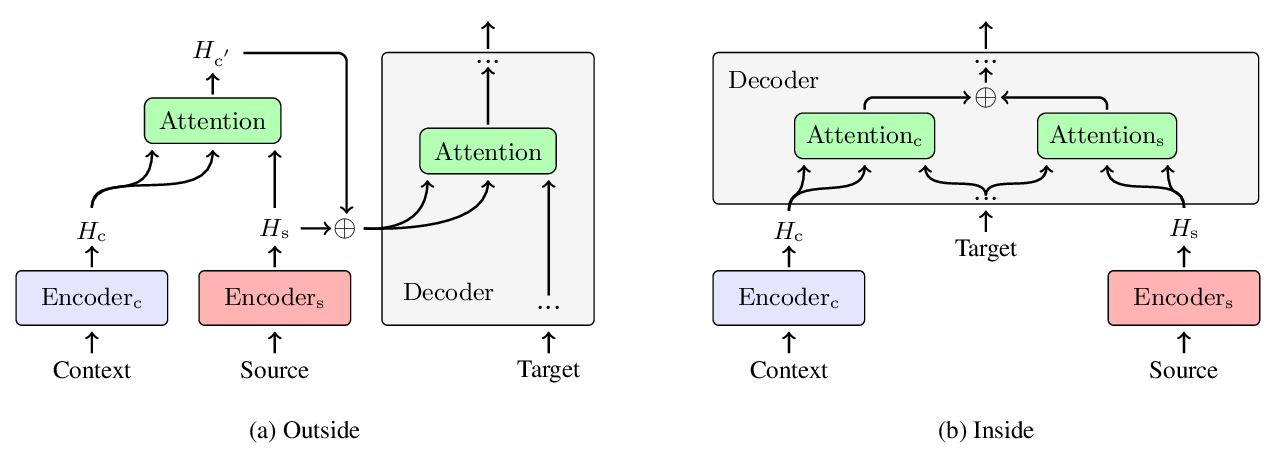
Multilingual Universal Sentence Encoder for Semantic Retrieval
Yinfei Yang, Daniel Cer, Amin Ahmad, Mandy Guo, Jax Law, Noah Constant, Gustavo Hernandez Abrego, Steve Yuan, Chris Tar, Yun-hsuan Sung, Brian Strope, Ray Kurzweil,
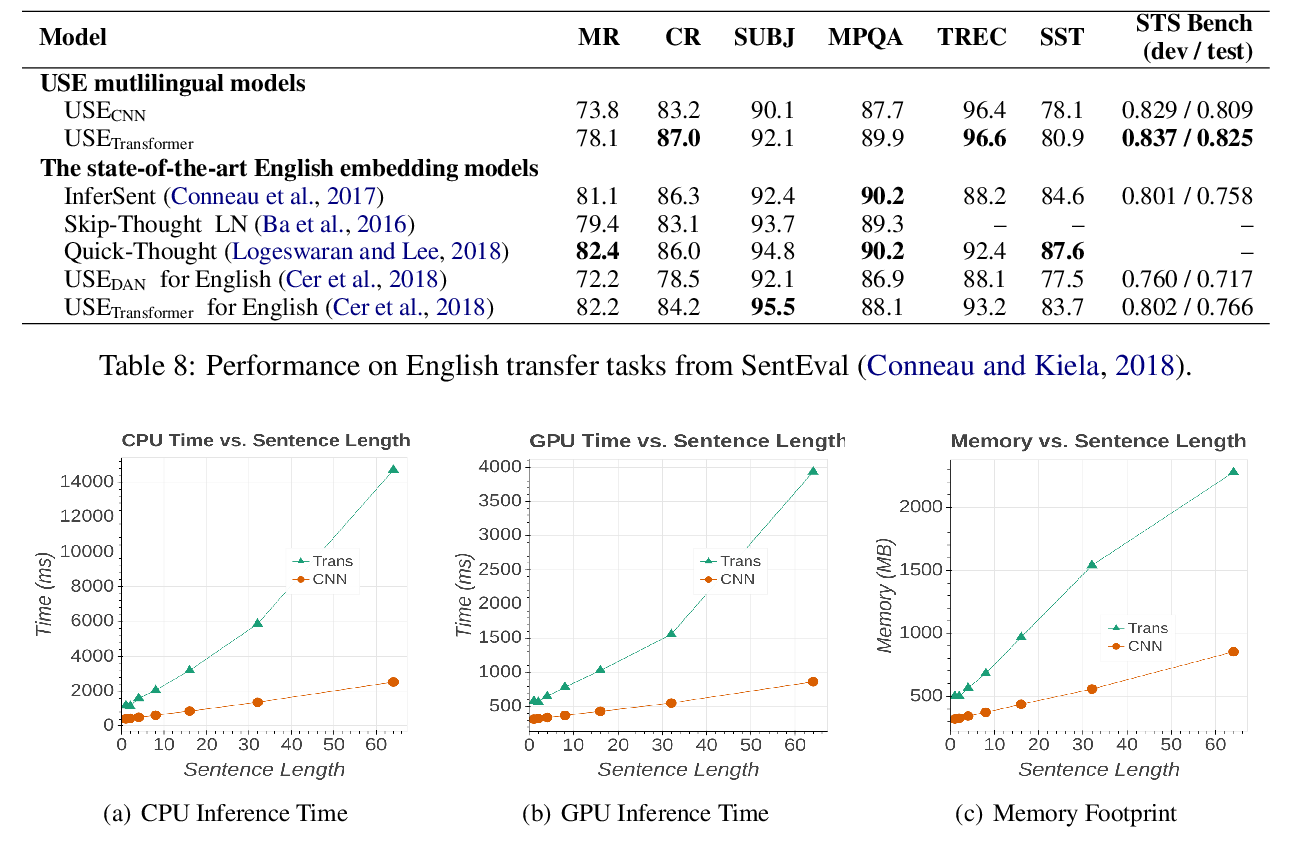