SUPERT: Towards New Frontiers in Unsupervised Evaluation Metrics for Multi-Document Summarization
Yang Gao, Wei Zhao, Steffen Eger
Summarization Short Paper
Session 2A: Jul 6
(08:00-09:00 GMT)
Session 3B: Jul 6
(13:00-14:00 GMT)
Abstract:
We study unsupervised multi-document summarization evaluation metrics, which require neither human-written reference summaries nor human annotations (e.g. preferences, ratings, etc.). We propose SUPERT, which rates the quality of a summary by measuring its semantic similarity with a pseudo reference summary, i.e. selected salient sentences from the source documents, using contextualized embeddings and soft token alignment techniques. Compared to the state-of-the-art unsupervised evaluation metrics, SUPERT correlates better with human ratings by 18- 39%. Furthermore, we use SUPERT as rewards to guide a neural-based reinforcement learning summarizer, yielding favorable performance compared to the state-of-the-art unsupervised summarizers. All source code is available at https://github.com/yg211/acl20-ref-free-eval.
You can open the
pre-recorded video
in a separate window.
NOTE: The SlidesLive video may display a random order of the authors.
The correct author list is shown at the top of this webpage.
Similar Papers
Facet-Aware Evaluation for Extractive Summarization
Yuning Mao, Liyuan Liu, Qi Zhu, Xiang Ren, Jiawei Han,
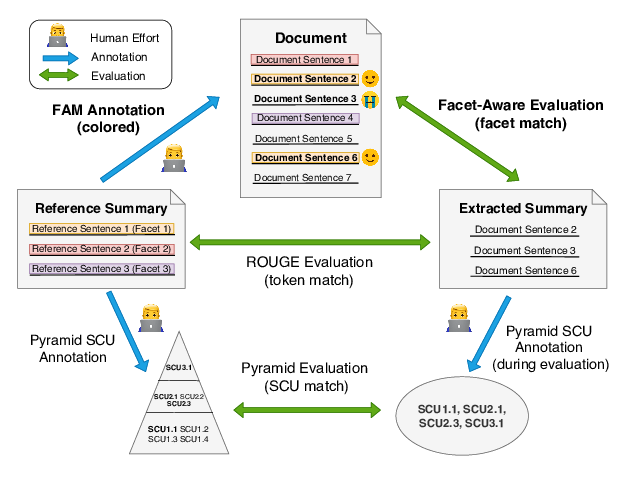
Designing Precise and Robust Dialogue Response Evaluators
Tianyu Zhao, Divesh Lala, Tatsuya Kawahara,
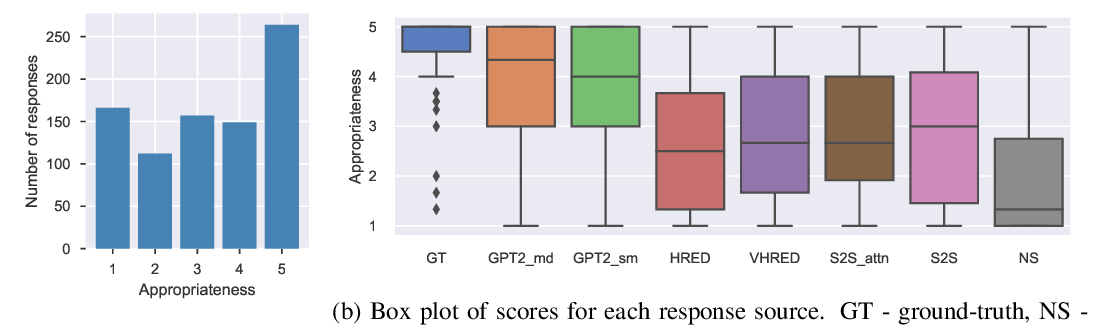
On Faithfulness and Factuality in Abstractive Summarization
Joshua Maynez, Shashi Narayan, Bernd Bohnet, Ryan McDonald,
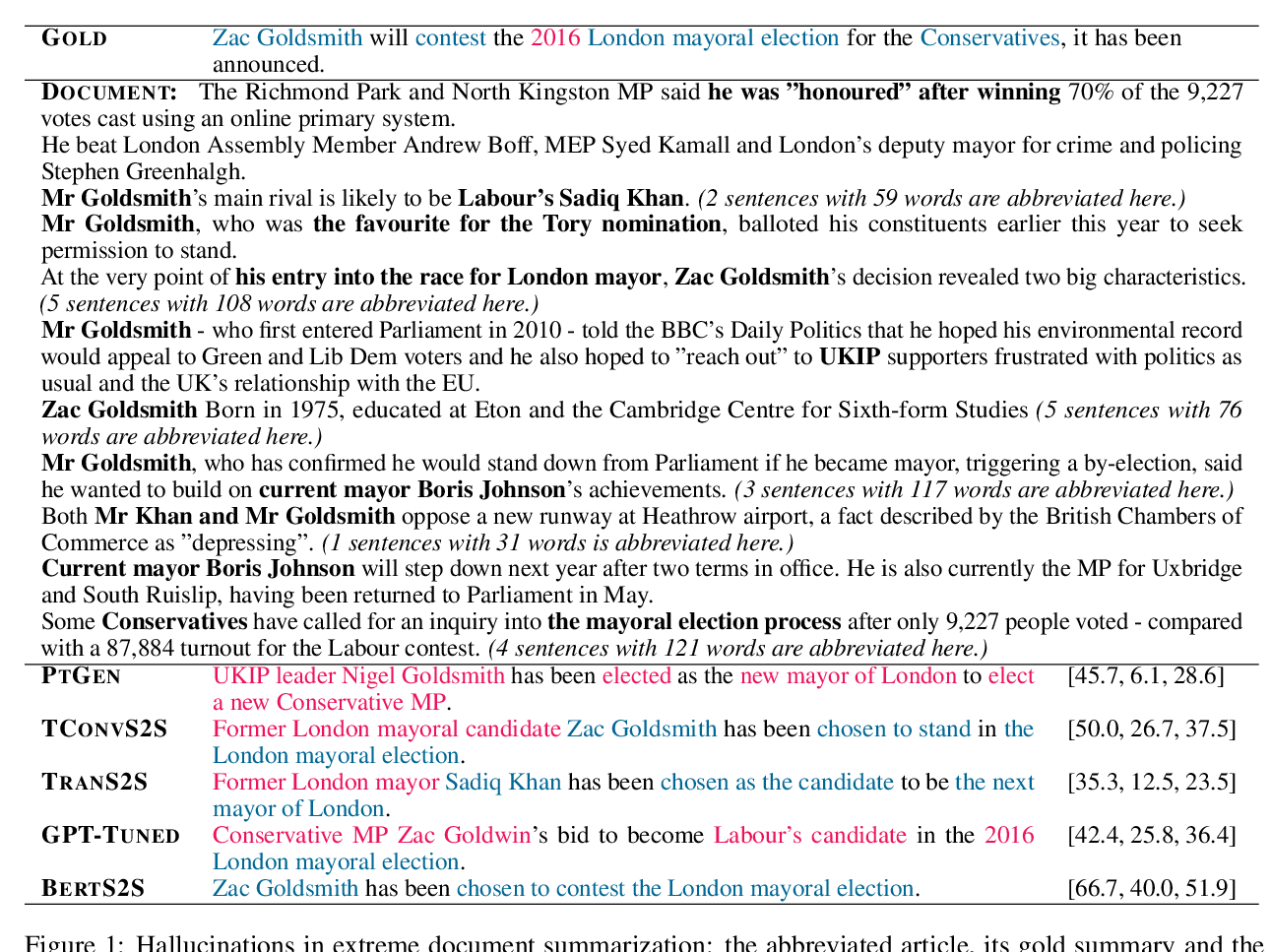
Personalized PageRank with Syntagmatic Information for Multilingual Word Sense Disambiguation
Federico Scozzafava, Marco Maru, Fabrizio Brignone, Giovanni Torrisi, Roberto Navigli,
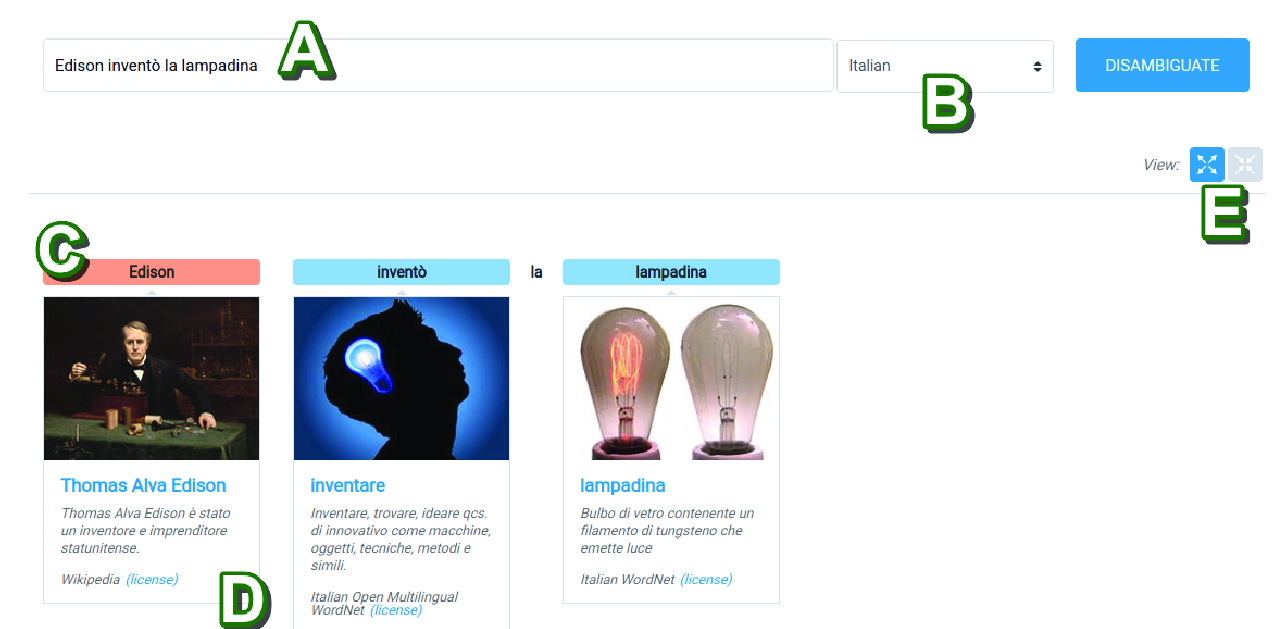