Learning Dialog Policies from Weak Demonstrations
Gabriel Gordon-Hall, Philip John Gorinski, Shay B. Cohen
Dialogue and Interactive Systems Long Paper
Session 2B: Jul 6
(09:00-10:00 GMT)
Session 3B: Jul 6
(13:00-14:00 GMT)
Abstract:
Deep reinforcement learning is a promising approach to training a dialog manager, but current methods struggle with the large state and action spaces of multi-domain dialog systems. Building upon Deep Q-learning from Demonstrations (DQfD), an algorithm that scores highly in difficult Atari games, we leverage dialog data to guide the agent to successfully respond to a user's requests. We make progressively fewer assumptions about the data needed, using labeled, reduced-labeled, and even unlabeled data to train expert demonstrators. We introduce Reinforced Fine-tune Learning, an extension to DQfD, enabling us to overcome the domain gap between the datasets and the environment. Experiments in a challenging multi-domain dialog system framework validate our approaches, and get high success rates even when trained on out-of-domain data.
You can open the
pre-recorded video
in a separate window.
NOTE: The SlidesLive video may display a random order of the authors.
The correct author list is shown at the top of this webpage.
Similar Papers
Conversation Learner - A Machine Teaching Tool for Building Dialog Managers for Task-Oriented Dialog Systems
Swadheen Shukla, Lars Liden, Shahin Shayandeh, Eslam Kamal, Jinchao Li, Matt Mazzola, Thomas Park, Baolin Peng, Jianfeng Gao,
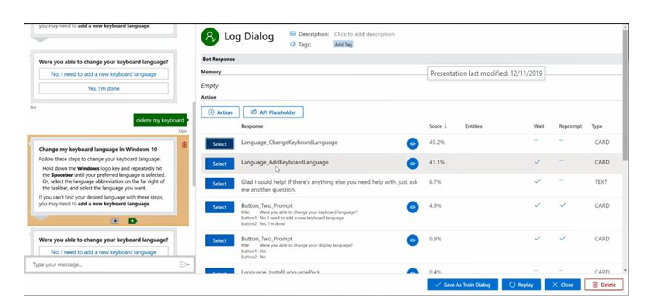
Multi-Agent Task-Oriented Dialog Policy Learning with Role-Aware Reward Decomposition
Ryuichi Takanobu, Runze Liang, Minlie Huang,
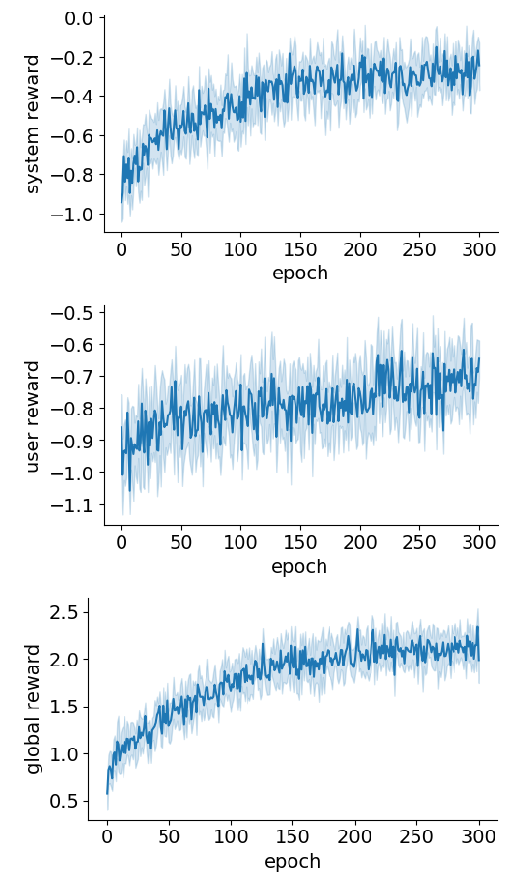
Conversational Graph Grounded Policy Learning for Open-Domain Conversation Generation
Jun Xu, Haifeng Wang, Zheng-Yu Niu, Hua Wu, Wanxiang Che, Ting Liu,
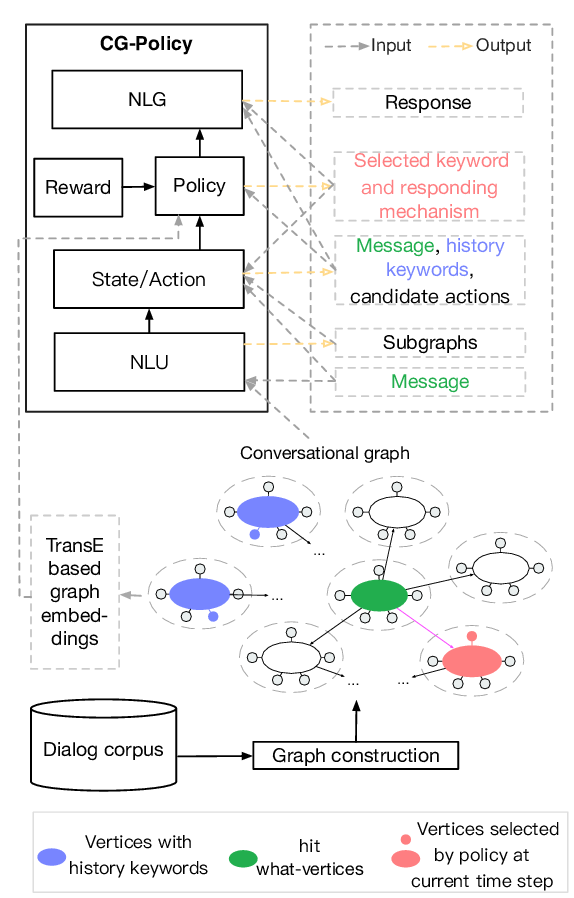