MuTual: A Dataset for Multi-Turn Dialogue Reasoning
Leyang Cui, Yu Wu, Shujie Liu, Yue Zhang, Ming Zhou
Dialogue and Interactive Systems Long Paper
Session 2B: Jul 6
(09:00-10:00 GMT)
Session 3A: Jul 6
(12:00-13:00 GMT)
Abstract:
Non-task oriented dialogue systems have achieved great success in recent years due to largely accessible conversation data and the development of deep learning techniques. Given a context, current systems are able to yield a relevant and fluent response, but sometimes make logical mistakes because of weak reasoning capabilities. To facilitate the conversation reasoning research, we introduce MuTual, a novel dataset for Multi-Turn dialogue Reasoning, consisting of 8,860 manually annotated dialogues based on Chinese student English listening comprehension exams. Compared to previous benchmarks for non-task oriented dialogue systems, MuTual is much more challenging since it requires a model that be able to handle various reasoning problems. Empirical results show that state-of-the-art methods only reach 71%, which is far behind human performance of 94%, indicating that there is ample room for improving reasoning ability.
You can open the
pre-recorded video
in a separate window.
NOTE: The SlidesLive video may display a random order of the authors.
The correct author list is shown at the top of this webpage.
Similar Papers
DIALOGPT : Large-Scale Generative Pre-training for Conversational Response Generation
Yizhe Zhang, Siqi Sun, Michel Galley, Yen-Chun Chen, Chris Brockett, Xiang Gao, Jianfeng Gao, Jingjing Liu, Bill Dolan,
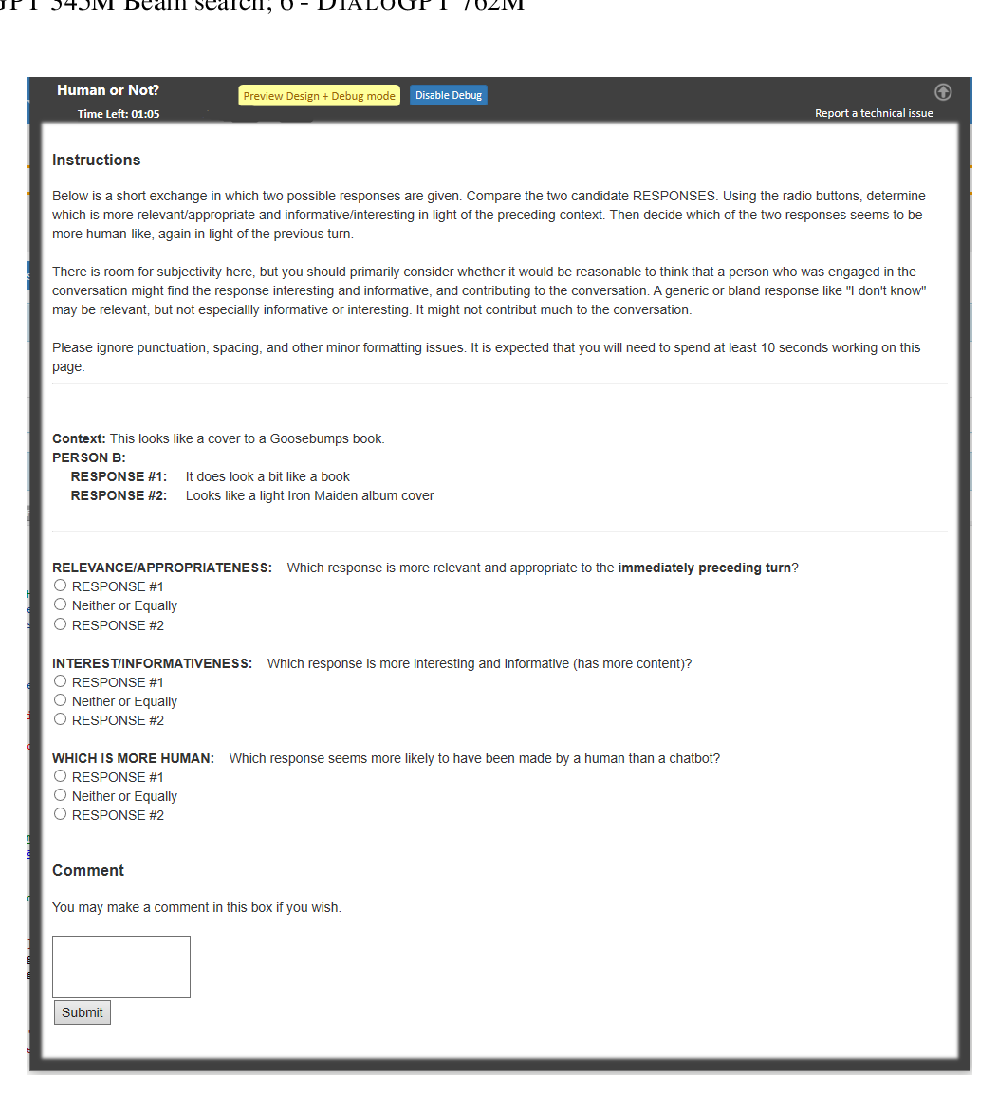
Semantic Graphs for Generating Deep Questions
Liangming Pan, Yuxi Xie, Yansong Feng, Tat-Seng Chua, Min-Yen Kan,
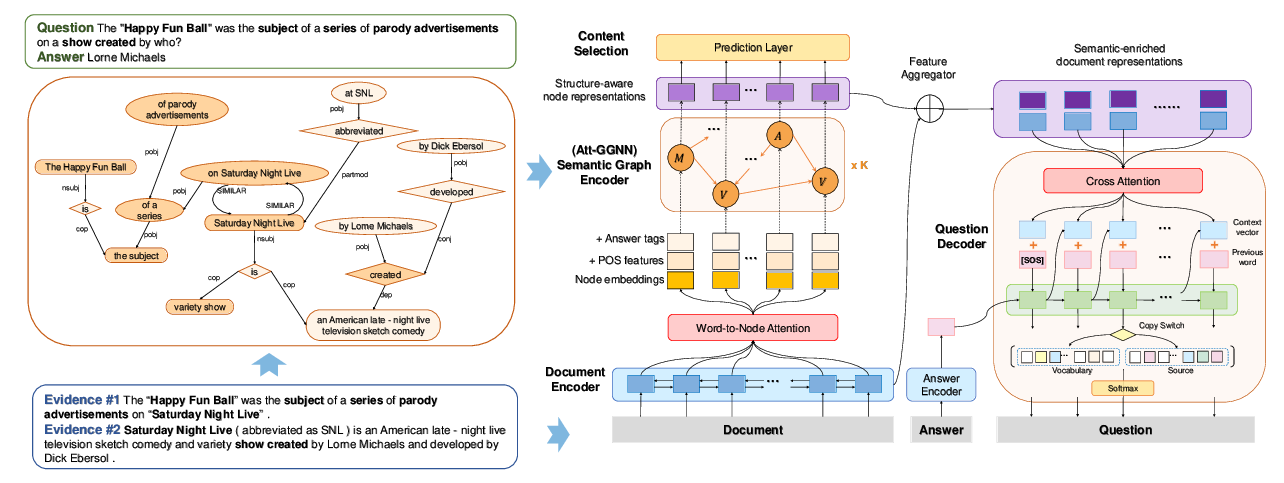
INFOTABS: Inference on Tables as Semi-structured Data
Vivek Gupta, Maitrey Mehta, Pegah Nokhiz, Vivek Srikumar,
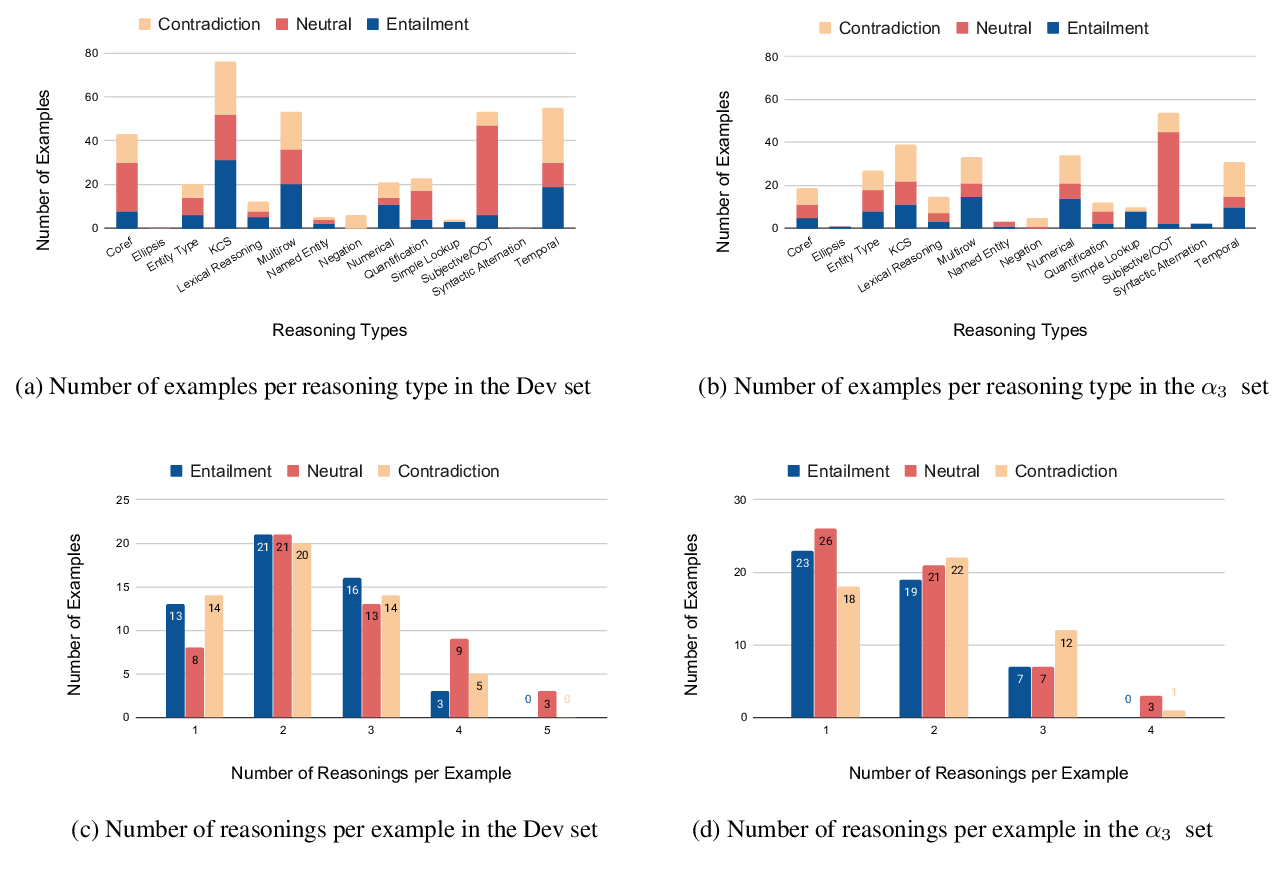
Words Aren't Enough, Their Order Matters: On the Robustness of Grounding Visual Referring Expressions
Arjun Akula, Spandana Gella, Yaser Al-Onaizan, Song-Chun Zhu, Siva Reddy,
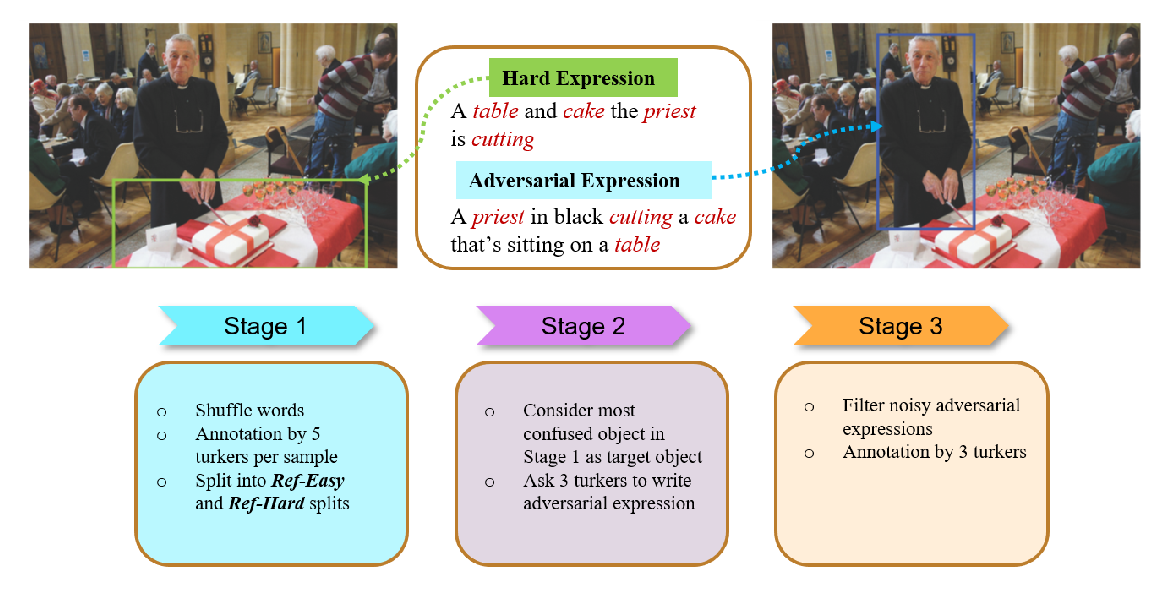