On the Limitations of Cross-lingual Encoders as Exposed by Reference-Free Machine Translation Evaluation
Wei Zhao, Goran Glavaš, Maxime Peyrard, Yang Gao, Robert West, Steffen Eger
Machine Translation Long Paper
Session 2B: Jul 6
(09:00-10:00 GMT)
Session 3B: Jul 6
(13:00-14:00 GMT)
Abstract:
Evaluation of cross-lingual encoders is usually performed either via zero-shot cross-lingual transfer in supervised downstream tasks or via unsupervised cross-lingual textual similarity. In this paper, we concern ourselves with reference-free machine translation (MT) evaluation where we directly compare source texts to (sometimes low-quality) system translations, which represents a natural adversarial setup for multilingual encoders. Reference-free evaluation holds the promise of web-scale comparison of MT systems. We systematically investigate a range of metrics based on state-of-the-art cross-lingual semantic representations obtained with pretrained M-BERT and LASER. We find that they perform poorly as semantic encoders for reference-free MT evaluation and identify their two key limitations, namely, (a) a semantic mismatch between representations of mutual translations and, more prominently, (b) the inability to punish "translationese", i.e., low-quality literal translations. We propose two partial remedies: (1) post-hoc re-alignment of the vector spaces and (2) coupling of semantic-similarity based metrics with target-side language modeling. In segment-level MT evaluation, our best metric surpasses reference-based BLEU by 5.7 correlation points.
You can open the
pre-recorded video
in a separate window.
NOTE: The SlidesLive video may display a random order of the authors.
The correct author list is shown at the top of this webpage.
Similar Papers
Jointly Learning to Align and Summarize for Neural Cross-Lingual Summarization
Yue Cao, Hui Liu, Xiaojun Wan,
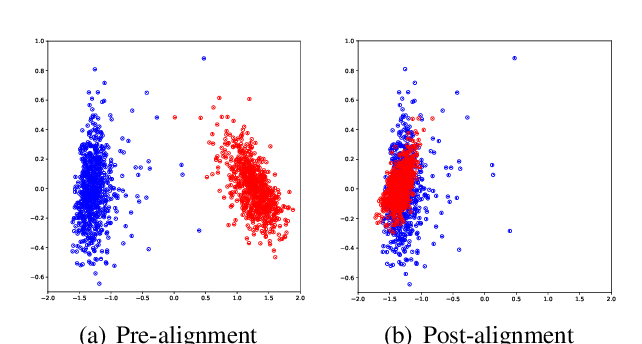
On the Cross-lingual Transferability of Monolingual Representations
Mikel Artetxe, Sebastian Ruder, Dani Yogatama,
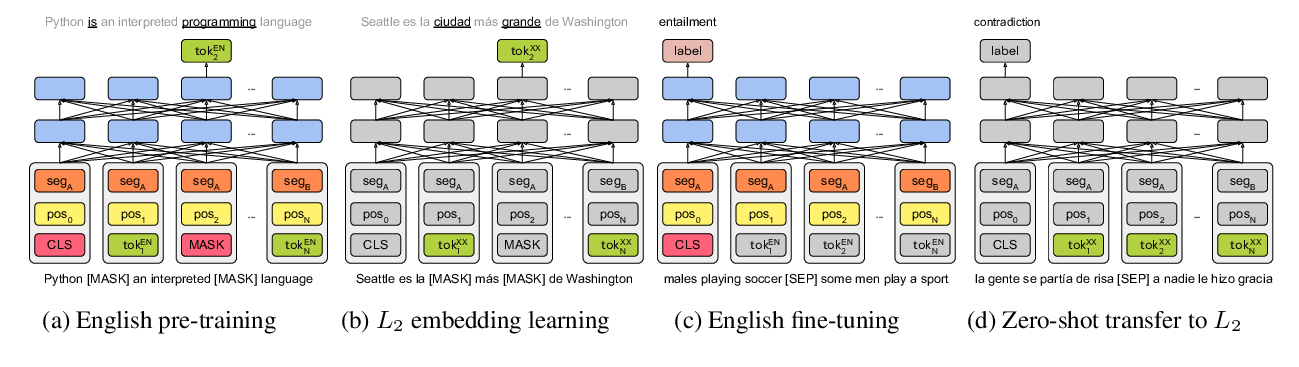
A Call for More Rigor in Unsupervised Cross-lingual Learning
Mikel Artetxe, Sebastian Ruder, Dani Yogatama, Gorka Labaka, Eneko Agirre,
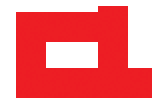