Multi-Hypothesis Machine Translation Evaluation
Marina Fomicheva, Lucia Specia, Francisco Guzmán
Resources and Evaluation Long Paper
Session 2A: Jul 6
(08:00-09:00 GMT)
Session 3A: Jul 6
(12:00-13:00 GMT)
Abstract:
Reliably evaluating Machine Translation (MT) through automated metrics is a long-standing problem. One of the main challenges is the fact that multiple outputs can be equally valid. Attempts to minimise this issue include metrics that relax the matching of MT output and reference strings, and the use of multiple references. The latter has been shown to significantly improve the performance of evaluation metrics. However, collecting multiple references is expensive and in practice a single reference is generally used. In this paper, we propose an alternative approach: instead of modelling linguistic variation in human reference we exploit the MT model uncertainty to generate multiple diverse translations and use these: (i) as surrogates to reference translations; (ii) to obtain a quantification of translation variability to either complement existing metric scores or (iii) replace references altogether. We show that for a number of popular evaluation metrics our variability estimates lead to substantial improvements in correlation with human judgements of quality by up 15%.
You can open the
pre-recorded video
in a separate window.
NOTE: The SlidesLive video may display a random order of the authors.
The correct author list is shown at the top of this webpage.
Similar Papers
On the Limitations of Cross-lingual Encoders as Exposed by Reference-Free Machine Translation Evaluation
Wei Zhao, Goran Glavaš, Maxime Peyrard, Yang Gao, Robert West, Steffen Eger,
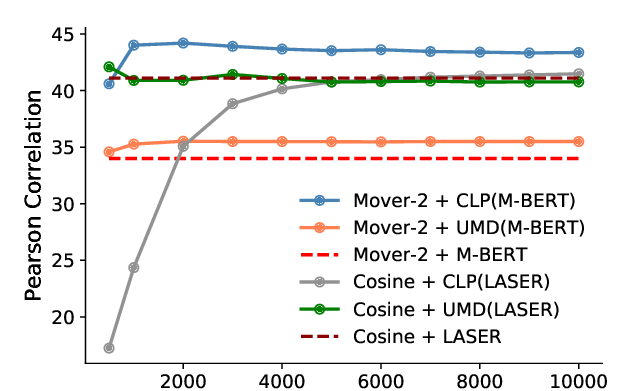
uBLEU: Uncertainty-Aware Automatic Evaluation Method for Open-Domain Dialogue Systems
Tsuta Yuma, Naoki Yoshinaga, Masashi Toyoda,
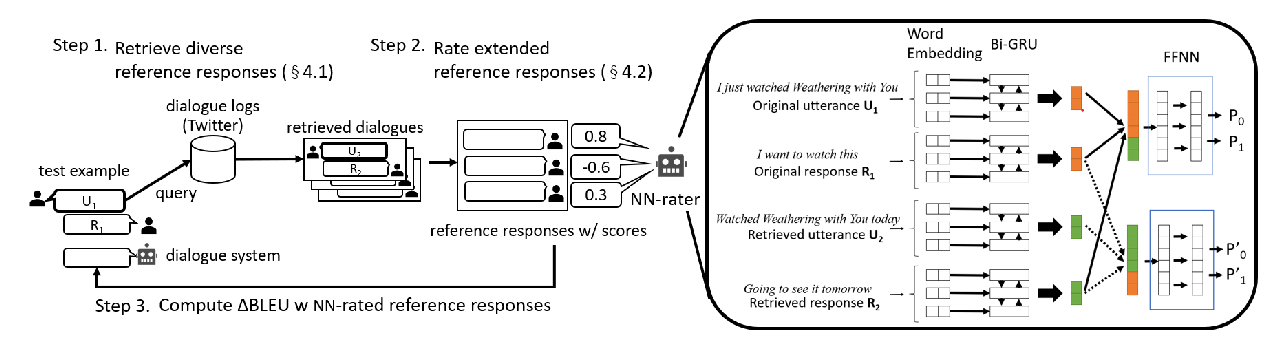
Improving Image Captioning Evaluation by Considering Inter References Variance
Yanzhi Yi, Hangyu Deng, Jinglu Hu,
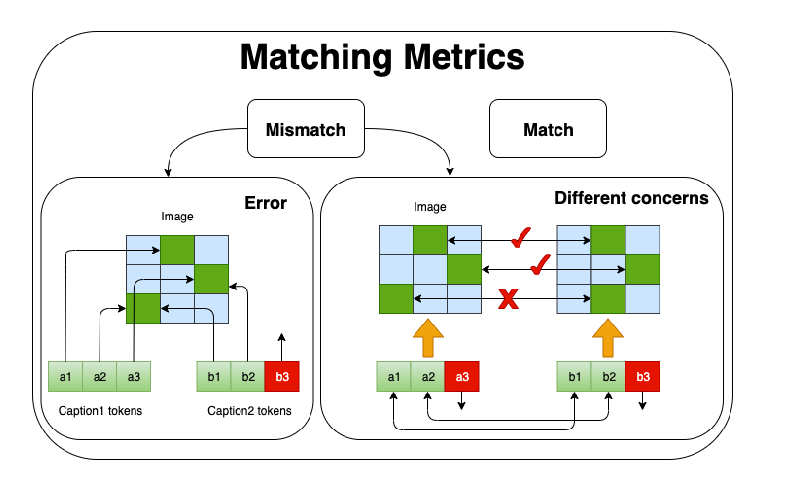
On The Evaluation of Machine Translation SystemsTrained With Back-Translation
Sergey Edunov, Myle Ott, Marc'Aurelio Ranzato, Michael Auli,
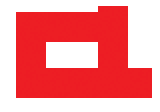