You Don't Have Time to Read This: An Exploration of Document Reading Time Prediction
Orion Weller, Jordan Hildebrandt, Ilya Reznik, Christopher Challis, E. Shannon Tass, Quinn Snell, Kevin Seppi
Cognitive Modeling and Psycholinguistics Short Paper
Session 3A: Jul 6
(12:00-13:00 GMT / 12:00-13:00 GMT)
Session 4A: Jul 6
(17:00-18:00 GMT / 17:00-18:00 GMT)
Abstract:
Predicting reading time has been a subject of much previous work, focusing on how different words affect human processing, measured by reading time. However, previous work has dealt with a limited number of participants as well as word level only predictions (i.e. predicting the time to read a single word). We seek to extend these works by examining whether or not document level predictions are effective, given additional information such as subject matter, font characteristics, and readability metrics. We perform a novel experiment to examine how different features of text contribute to the time it takes to read, distributing and collecting data from over a thousand participants. We then employ a large number of machine learning methods to predict a user's reading time. We find that despite extensive research showing that word level reading time can be most effectively predicted by neural networks, larger scale text can be easily and most accurately predicted by one factor, the number of words.
You can open the
pre-recorded video
in a separate window.
NOTE: The SlidesLive video may display a random order of the authors.
The correct author list is shown at the top of this webpage.
Similar Papers
STARC: Structured Annotations for Reading Comprehension
Yevgeni Berzak, Jonathan Malmaud, Roger Levy,
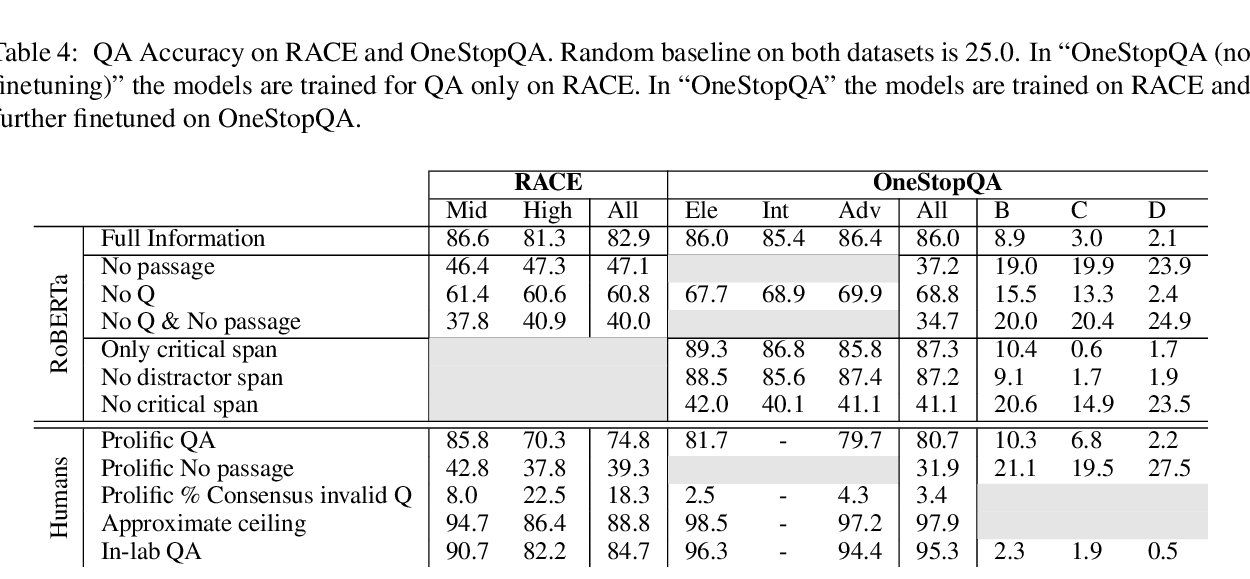
Machine Reading of Historical Events
Or Honovich, Lucas Torroba Hennigen, Omri Abend, Shay B. Cohen,
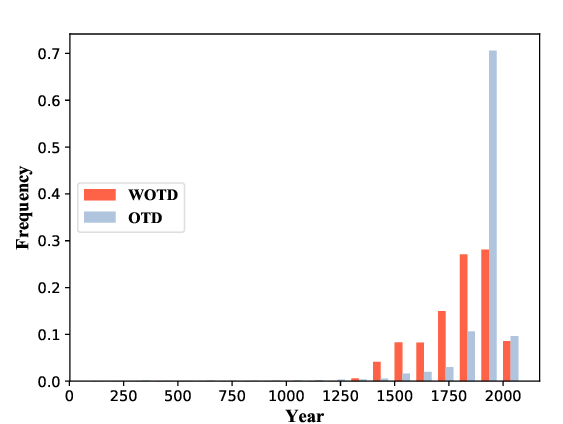
Dynamic Sampling Strategies for Multi-Task Reading Comprehension
Ananth Gottumukkala, Dheeru Dua, Sameer Singh, Matt Gardner,
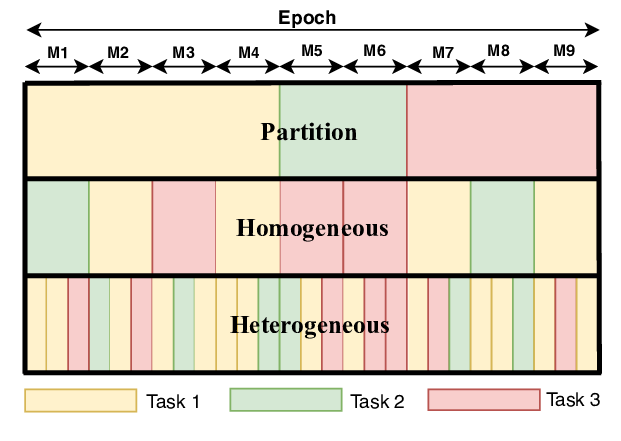