Dynamic Sampling Strategies for Multi-Task Reading Comprehension
Ananth Gottumukkala, Dheeru Dua, Sameer Singh, Matt Gardner
Question Answering Short Paper
Session 1B: Jul 6
(06:00-07:00 GMT)
Session 4A: Jul 6
(17:00-18:00 GMT)
Abstract:
Building general reading comprehension systems, capable of solving multiple datasets at the same time, is a recent aspirational goal in the research community. Prior work has focused on model architecture or generalization to held out datasets, and largely passed over the particulars of the multi-task learning set up. We show that a simple dynamic sampling strategy, selecting instances for training proportional to the multi-task model's current performance on a dataset relative to its single task performance, gives substantive gains over prior multi-task sampling strategies, mitigating the catastrophic forgetting that is common in multi-task learning. We also demonstrate that allowing instances of different tasks to be interleaved as much as possible between each epoch and batch has a clear benefit in multitask performance over forcing task homogeneity at the epoch or batch level. Our final model shows greatly increased performance over the best model on ORB, a recently-released multitask reading comprehension benchmark.
You can open the
pre-recorded video
in a separate window.
NOTE: The SlidesLive video may display a random order of the authors.
The correct author list is shown at the top of this webpage.
Similar Papers
The Dialogue Dodecathlon: Open-Domain Knowledge and Image Grounded Conversational Agents
Kurt Shuster, Da JU, Stephen Roller, Emily Dinan, Y-Lan Boureau, Jason Weston,
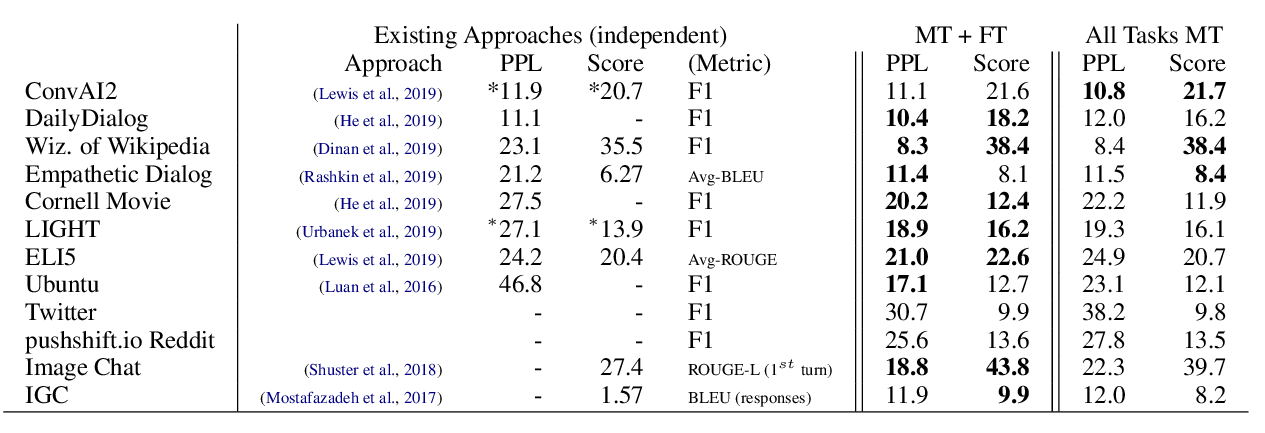
Learning a Multi-Domain Curriculum for Neural Machine Translation
Wei Wang, Ye Tian, Jiquan Ngiam, Yinfei Yang, Isaac Caswell, Zarana Parekh,
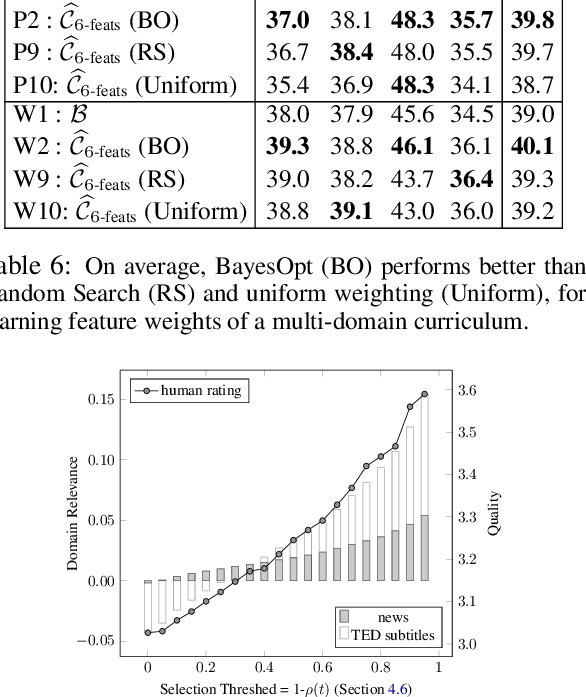
Can You Put it All Together: Evaluating Conversational Agents' Ability to Blend Skills
Eric Michael Smith, Mary Williamson, Kurt Shuster, Jason Weston, Y-Lan Boureau,
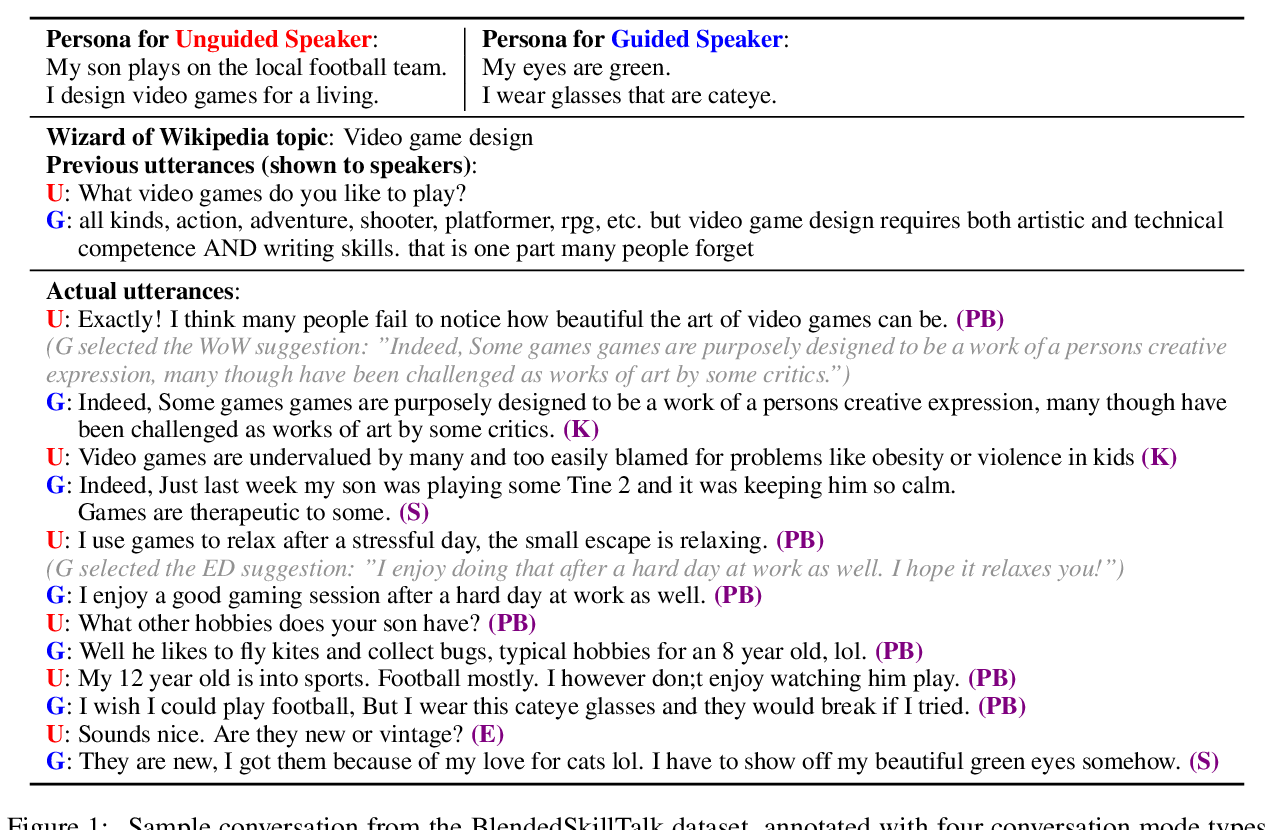