Machine Reading of Historical Events
Or Honovich, Lucas Torroba Hennigen, Omri Abend, Shay B. Cohen
Information Extraction Long Paper
Session 13A: Jul 8
(12:00-13:00 GMT)
Session 15B: Jul 8
(21:00-22:00 GMT)
Abstract:
Machine reading is an ambitious goal in NLP that subsumes a wide range of text understanding capabilities. Within this broad framework, we address the task of machine reading the time of historical events, compile datasets for the task, and develop a model for tackling it. Given a brief textual description of an event, we show that good performance can be achieved by extracting relevant sentences from Wikipedia, and applying a combination of task-specific and general-purpose feature embeddings for the classification. Furthermore, we establish a link between the historical event ordering task and the event focus time task from the information retrieval literature, showing they also provide a challenging test case for machine reading algorithms.
You can open the
pre-recorded video
in a separate window.
NOTE: The SlidesLive video may display a random order of the authors.
The correct author list is shown at the top of this webpage.
Similar Papers
You Don't Have Time to Read This: An Exploration of Document Reading Time Prediction
Orion Weller, Jordan Hildebrandt, Ilya Reznik, Christopher Challis, E. Shannon Tass, Quinn Snell, Kevin Seppi,
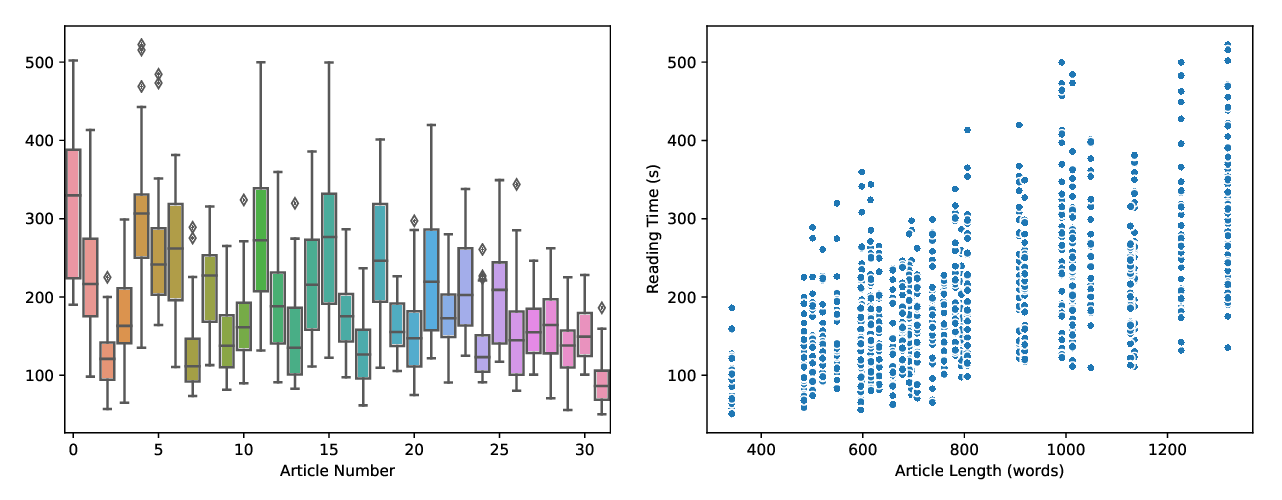
STARC: Structured Annotations for Reading Comprehension
Yevgeni Berzak, Jonathan Malmaud, Roger Levy,
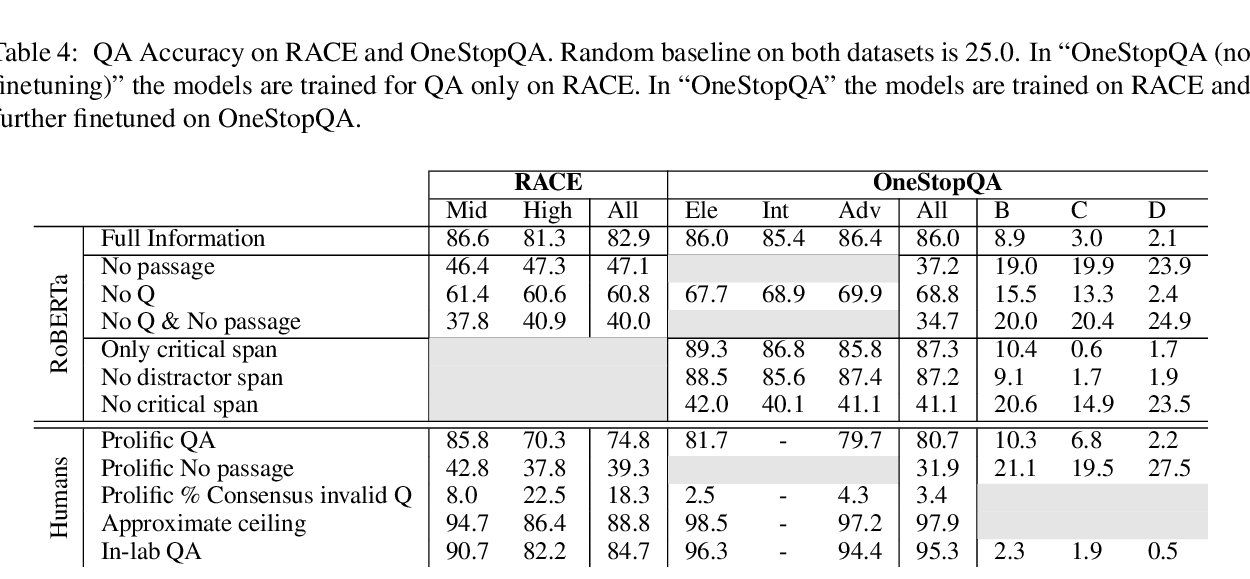
Evidence-Aware Inferential Text Generation with Vector Quantised Variational AutoEncoder
Daya Guo, Duyu Tang, Nan Duan, Jian Yin, Daxin Jiang, Ming Zhou,
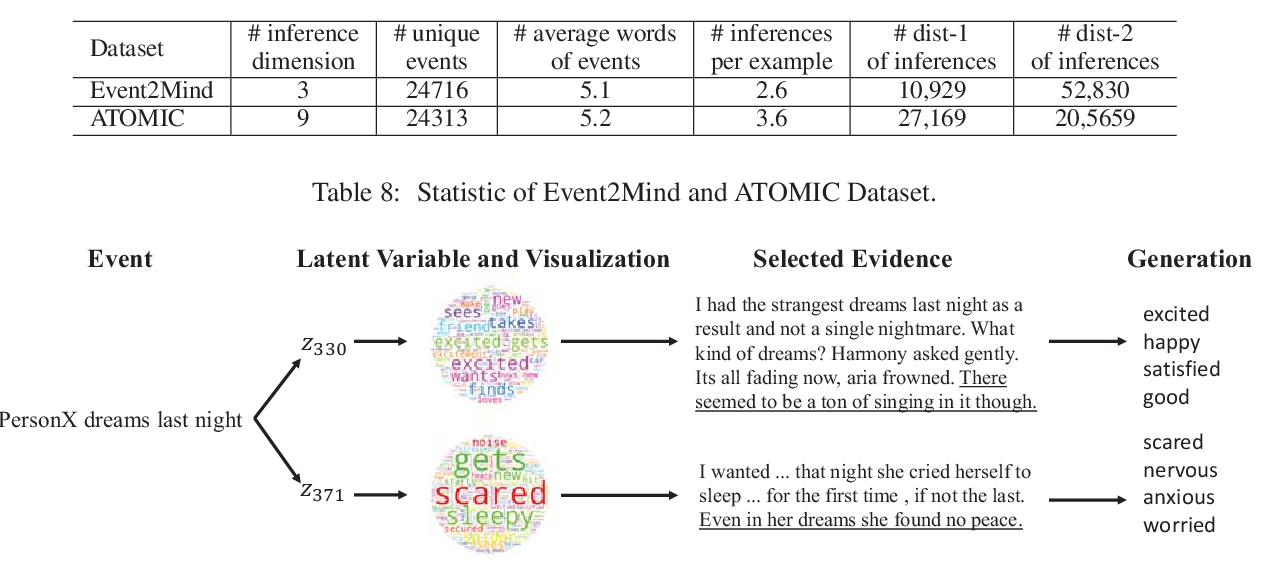
Temporal Common Sense Acquisition with Minimal Supervision
Ben Zhou, Qiang Ning, Daniel Khashabi, Dan Roth,
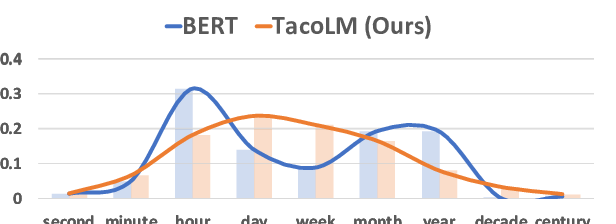