ExpBERT: Representation Engineering with Natural Language Explanations
Shikhar Murty, Pang Wei Koh, Percy Liang
Machine Learning for NLP Short Paper
Session 4A: Jul 6
(17:00-18:00 GMT / 17:00-18:00 GMT)
Session 5A: Jul 6
(20:00-21:00 GMT / 20:00-21:00 GMT)
Abstract:
Suppose we want to specify the inductive bias that married couples typically go on honeymoons for the task of extracting pairs of spouses from text. In this paper, we allow model developers to specify these types of inductive biases as natural language explanations. We use BERT fine-tuned on MultiNLI to "interpret" these explanations with respect to the input sentence, producing explanation-guided representations of the input. Across three relation extraction tasks, our method, ExpBERT, matches a BERT baseline but with 3--20x less labeled data and improves on the baseline by 3--10 F1 points with the same amount of labeled data.
You can open the
pre-recorded video
in a separate window.
NOTE: The SlidesLive video may display a random order of the authors.
The correct author list is shown at the top of this webpage.
Similar Papers
Syntactic Data Augmentation Increases Robustness to Inference Heuristics
Junghyun Min, R. Thomas McCoy, Dipanjan Das, Emily Pitler, Tal Linzen,
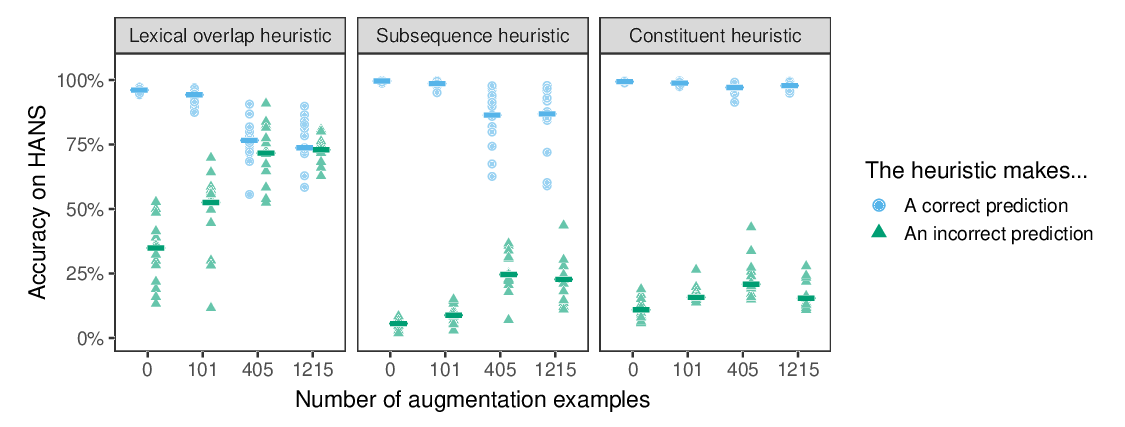
MobileBERT: a Compact Task-Agnostic BERT for Resource-Limited Devices
Zhiqing Sun, Hongkun Yu, Xiaodan Song, Renjie Liu, Yiming Yang, Denny Zhou,
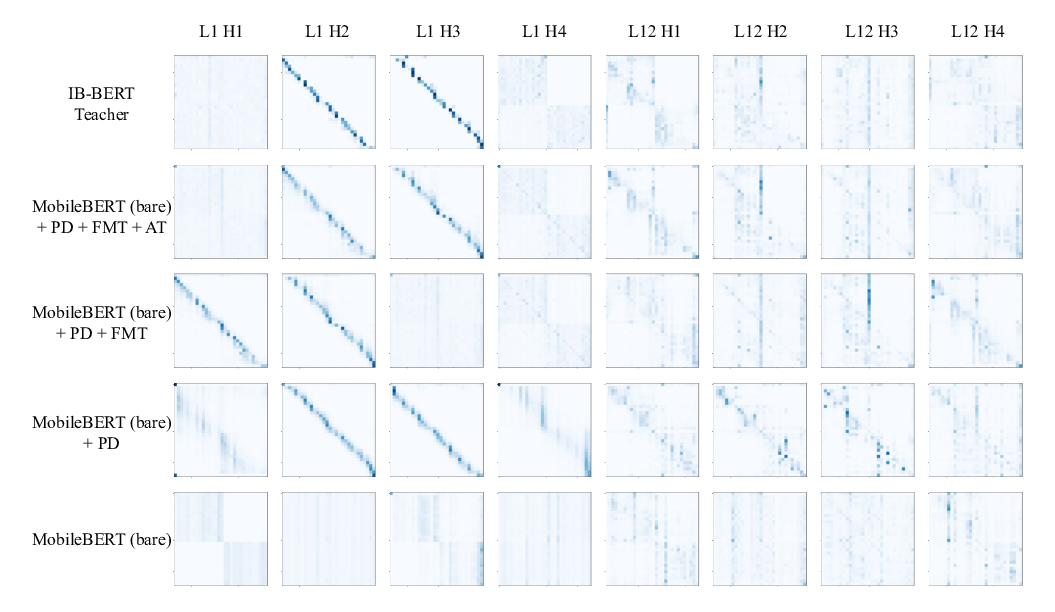
Are Natural Language Inference Models IMPPRESsive? Learning IMPlicature and PRESupposition
Paloma Jeretic, Alex Warstadt, Suvrat Bhooshan, Adina Williams,
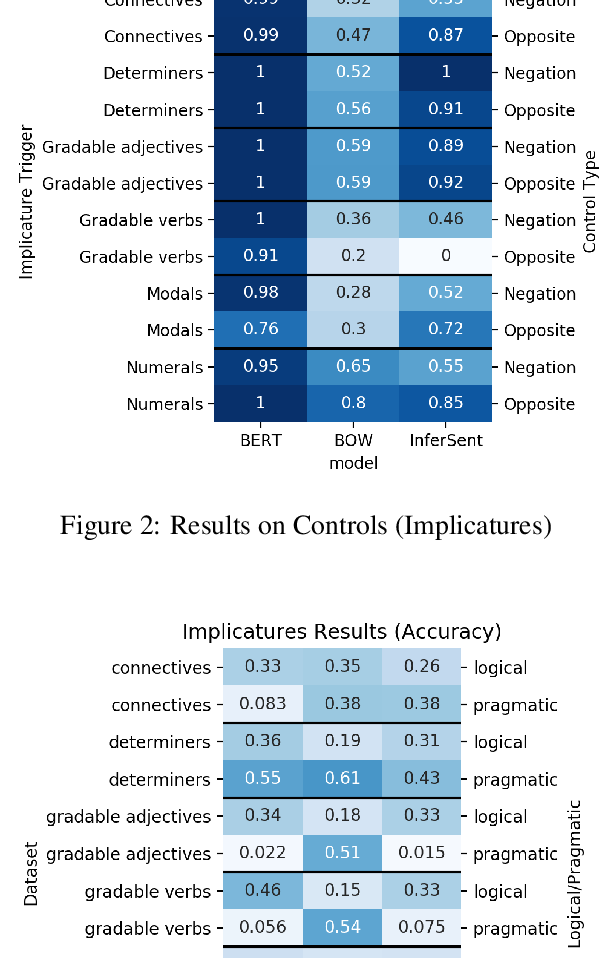
NILE : Natural Language Inference with Faithful Natural Language Explanations
Sawan Kumar, Partha Talukdar,
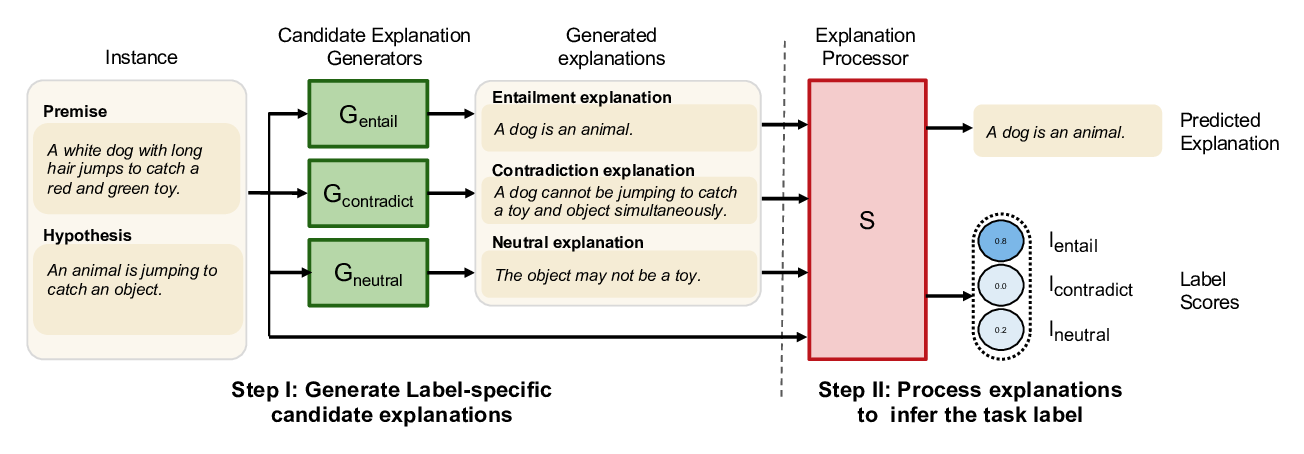