Syntactic Data Augmentation Increases Robustness to Inference Heuristics
Junghyun Min, R. Thomas McCoy, Dipanjan Das, Emily Pitler, Tal Linzen
Semantics: Textual Inference and Other Areas of Semantics Short Paper
Session 4A: Jul 6
(17:00-18:00 GMT)
Session 5A: Jul 6
(20:00-21:00 GMT)
Abstract:
Pretrained neural models such as BERT, when fine-tuned to perform natural language inference (NLI), often show high accuracy on standard datasets, but display a surprising lack of sensitivity to word order on controlled challenge sets. We hypothesize that this issue is not primarily caused by the pretrained model's limitations, but rather by the paucity of crowdsourced NLI examples that might convey the importance of syntactic structure at the fine-tuning stage. We explore several methods to augment standard training sets with syntactically informative examples, generated by applying syntactic transformations to sentences from the MNLI corpus. The best-performing augmentation method, subject/object inversion, improved BERT's accuracy on controlled examples that diagnose sensitivity to word order from 0.28 to 0.73, without affecting performance on the MNLI test set. This improvement generalized beyond the particular construction used for data augmentation, suggesting that augmentation causes BERT to recruit abstract syntactic representations.
You can open the
pre-recorded video
in a separate window.
NOTE: The SlidesLive video may display a random order of the authors.
The correct author list is shown at the top of this webpage.
Similar Papers
How does BERT's attention change when you fine-tune? An analysis methodology and a case study in negation scope
Yiyun Zhao, Steven Bethard,
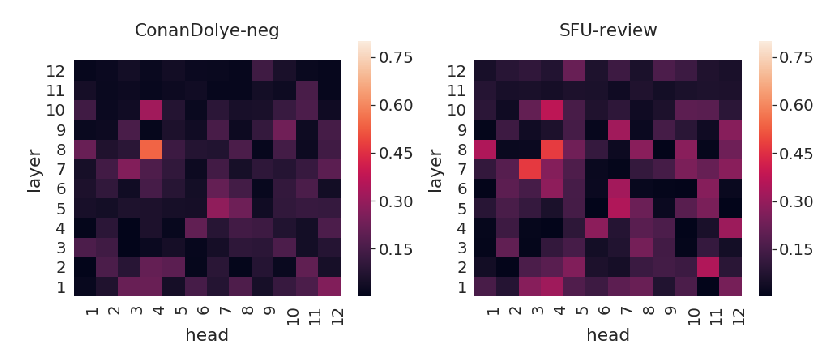
ExpBERT: Representation Engineering with Natural Language Explanations
Shikhar Murty, Pang Wei Koh, Percy Liang,
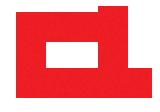
Representations of Syntax [MASK] Useful: Effects of Constituency and Dependency Structure in Recursive LSTMs
Michael Lepori, Tal Linzen, R. Thomas McCoy,
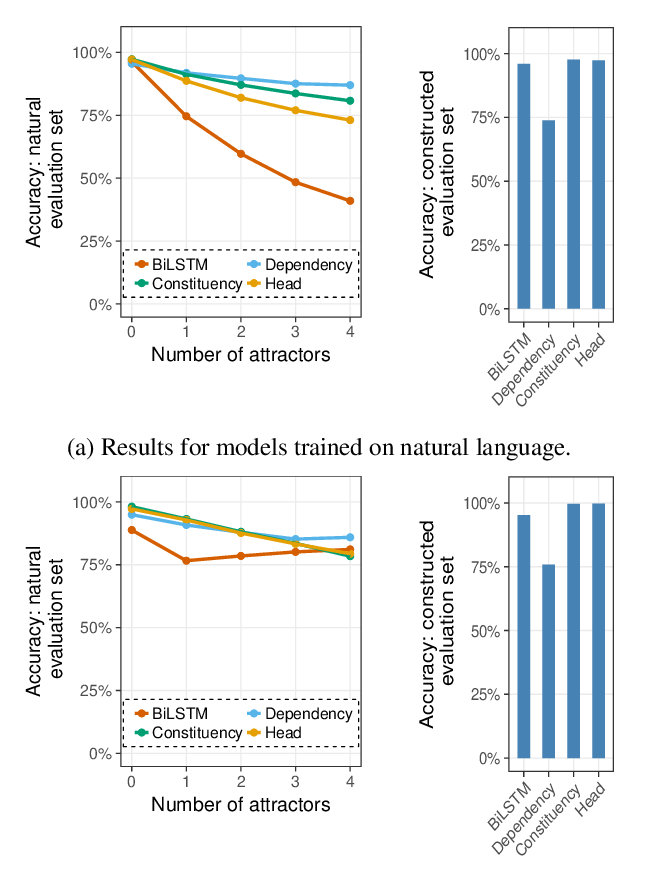
An Analysis of the Utility of Explicit Negative Examples to Improve the Syntactic Abilities of Neural Language Models
Hiroshi Noji, Hiroya Takamura,
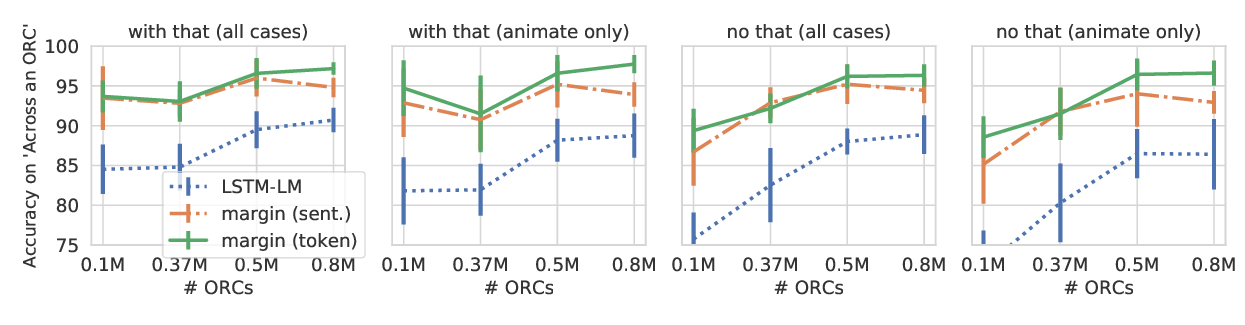