MixText: Linguistically-Informed Interpolation of Hidden Space for Semi-Supervised Text Classification
Jiaao Chen, Zichao Yang, Diyi Yang
Machine Learning for NLP Long Paper
Session 4A: Jul 6
(17:00-18:00 GMT)
Session 5A: Jul 6
(20:00-21:00 GMT)
Abstract:
This paper presents MixText, a semi-supervised learning method for text classification, which uses our newly designed data augmentation method called TMix. TMix creates a large amount of augmented training samples by interpolating text in hidden space. Moreover, we leverage recent advances in data augmentation to guess low-entropy labels for unlabeled data, hence making them as easy to use as labeled data. By mixing labeled, unlabeled and augmented data, MixText significantly outperformed current pre-trained and fined-tuned models and other state-of-the-art semi-supervised learning methods on several text classification benchmarks. The improvement is especially prominent when supervision is extremely limited. We have publicly released our code at https://github.com/GT-SALT/MixText.
You can open the
pre-recorded video
in a separate window.
NOTE: The SlidesLive video may display a random order of the authors.
The correct author list is shown at the top of this webpage.
Similar Papers
Improving Event Detection via Open-domain Trigger Knowledge
Meihan Tong, Bin Xu, Shuai Wang, Yixin Cao, Lei Hou, Juanzi Li, Jun Xie,
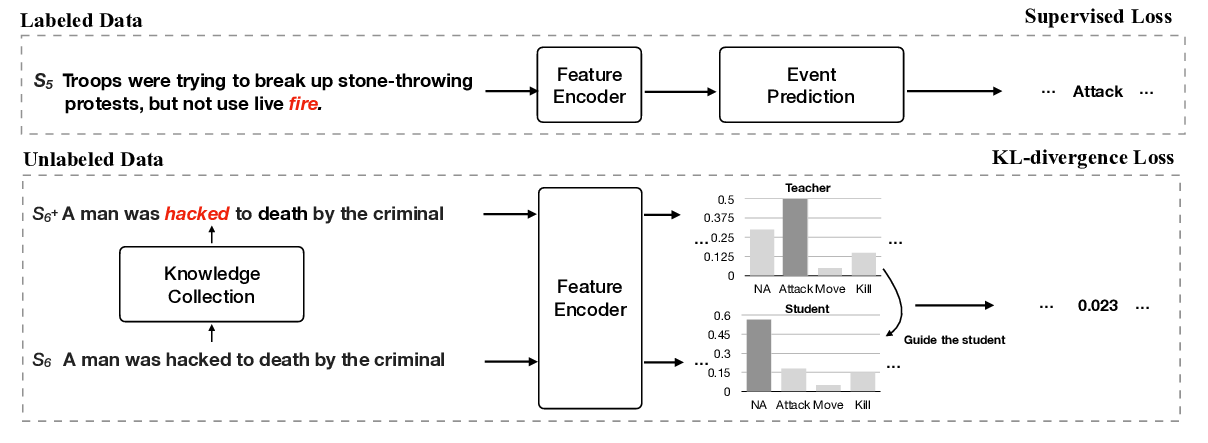
Incorporating External Knowledge through Pre-training for Natural Language to Code Generation
Frank F. Xu, Zhengbao Jiang, Pengcheng Yin, Bogdan Vasilescu, Graham Neubig,
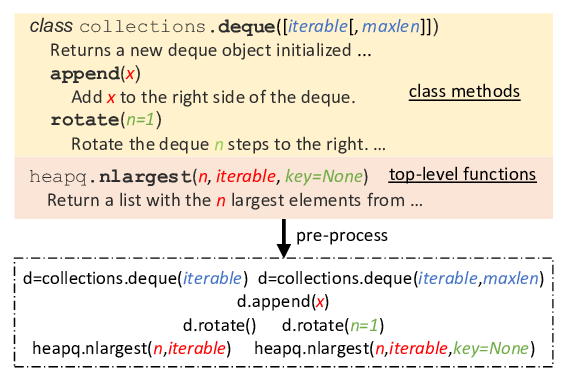
A Transformer-based Approach for Source Code Summarization
Wasi Ahmad, Saikat Chakraborty, Baishakhi Ray, Kai-Wei Chang,
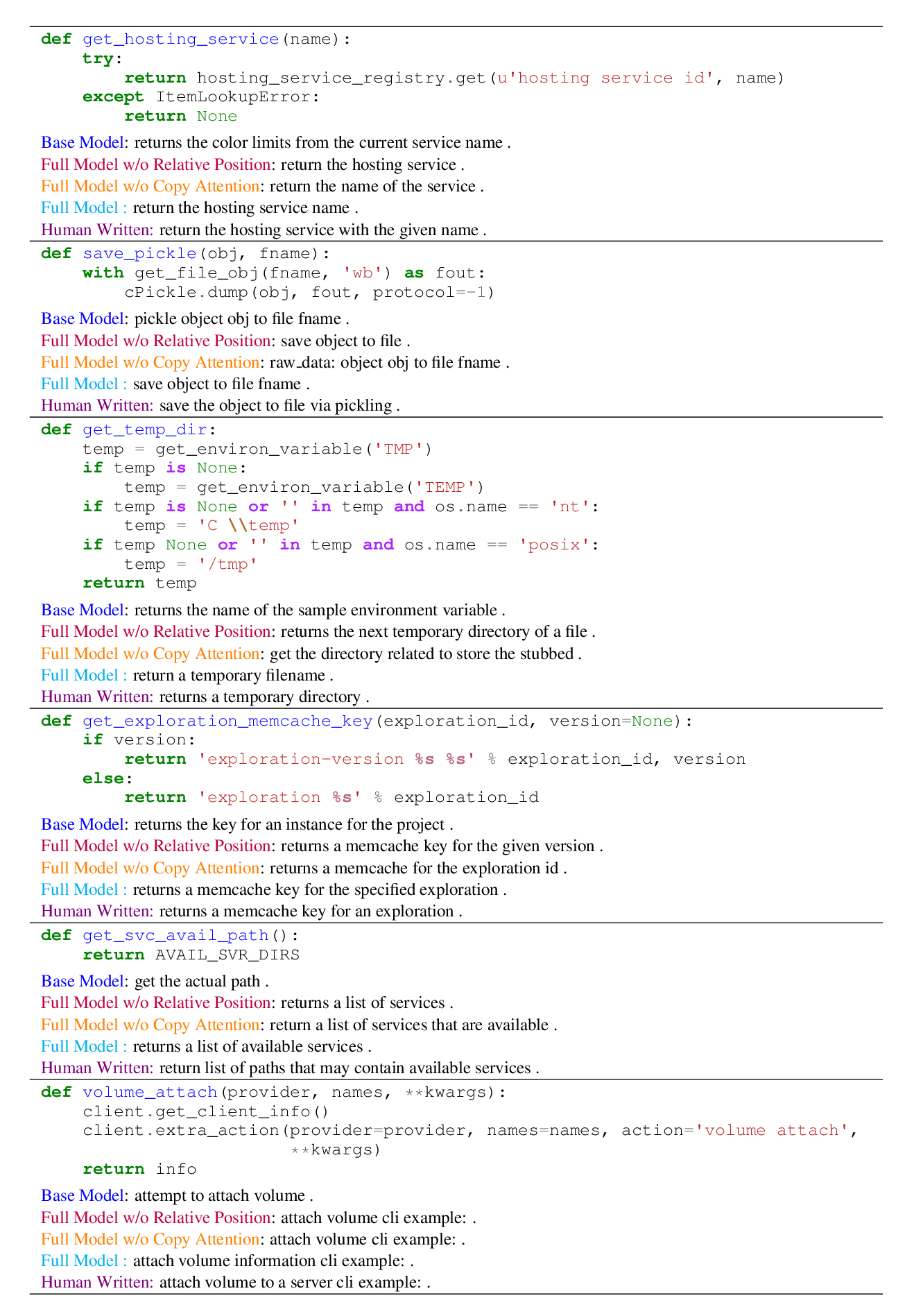
Interpretable Operational Risk Classification with Semi-Supervised Variational Autoencoder
Fan Zhou, Shengming Zhang, Yi Yang,
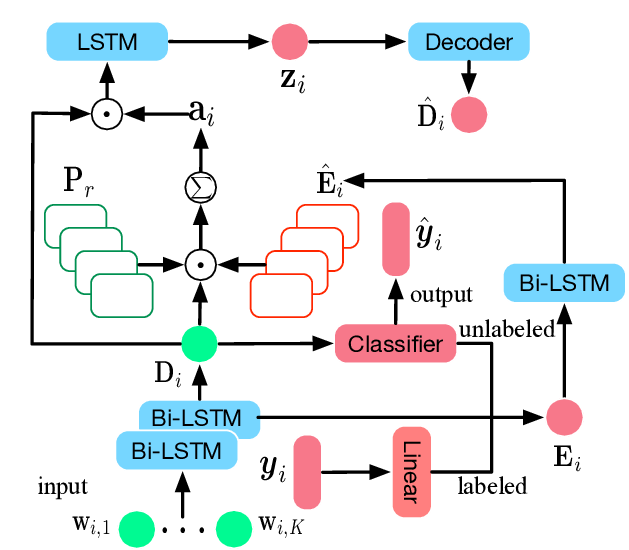