Interpretable Operational Risk Classification with Semi-Supervised Variational Autoencoder
Fan Zhou, Shengming Zhang, Yi Yang
NLP Applications Short Paper
Session 1B: Jul 6
(06:00-07:00 GMT)
Session 2B: Jul 6
(09:00-10:00 GMT)
Abstract:
Operational risk management is one of the biggest challenges nowadays faced by financial institutions. There are several major challenges of building a text classification system for automatic operational risk prediction, including imbalanced labeled/unlabeled data and lacking interpretability. To tackle these challenges, we present a semi-supervised text classification framework that integrates multi-head attention mechanism with Semi-supervised variational inference for Operational Risk Classification (SemiORC). We empirically evaluate the framework on a real-world dataset. The results demonstrate that our method can better utilize unlabeled data and learn visually interpretable document representations. SemiORC also outperforms other baseline methods on operational risk classification.
You can open the
pre-recorded video
in a separate window.
NOTE: The SlidesLive video may display a random order of the authors.
The correct author list is shown at the top of this webpage.
Similar Papers
MixText: Linguistically-Informed Interpolation of Hidden Space for Semi-Supervised Text Classification
Jiaao Chen, Zichao Yang, Diyi Yang,
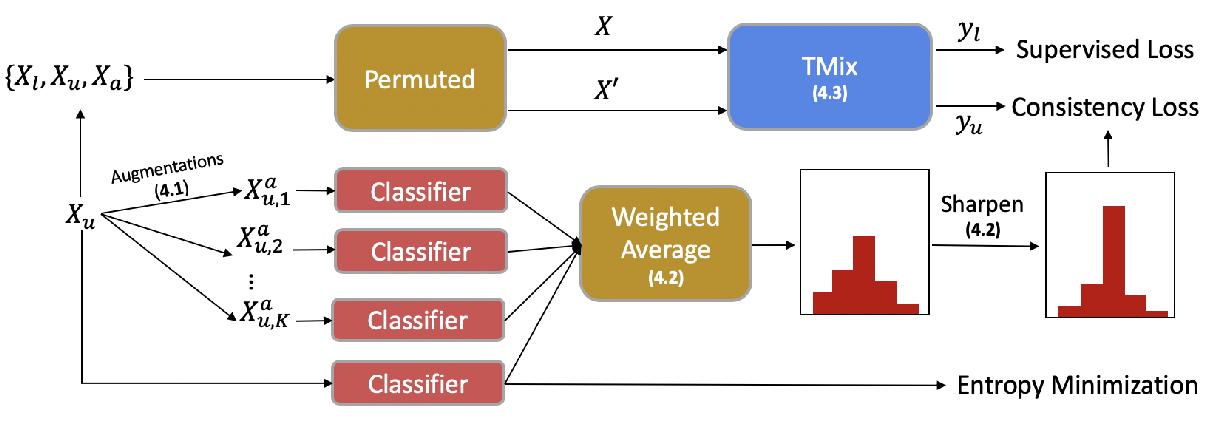
Camouflaged Chinese Spam Content Detection with Semi-supervised Generative Active Learning
Zhuoren Jiang, Zhe Gao, Yu Duan, Yangyang Kang, Changlong Sun, Qiong Zhang, Xiaozhong Liu,
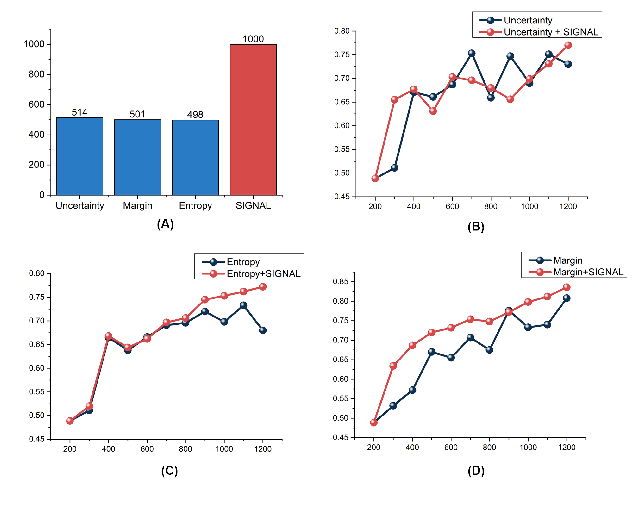
GAN-BERT: Generative Adversarial Learning for Robust Text Classification with a Bunch of Labeled Examples
Danilo Croce, Giuseppe Castellucci, Roberto Basili,
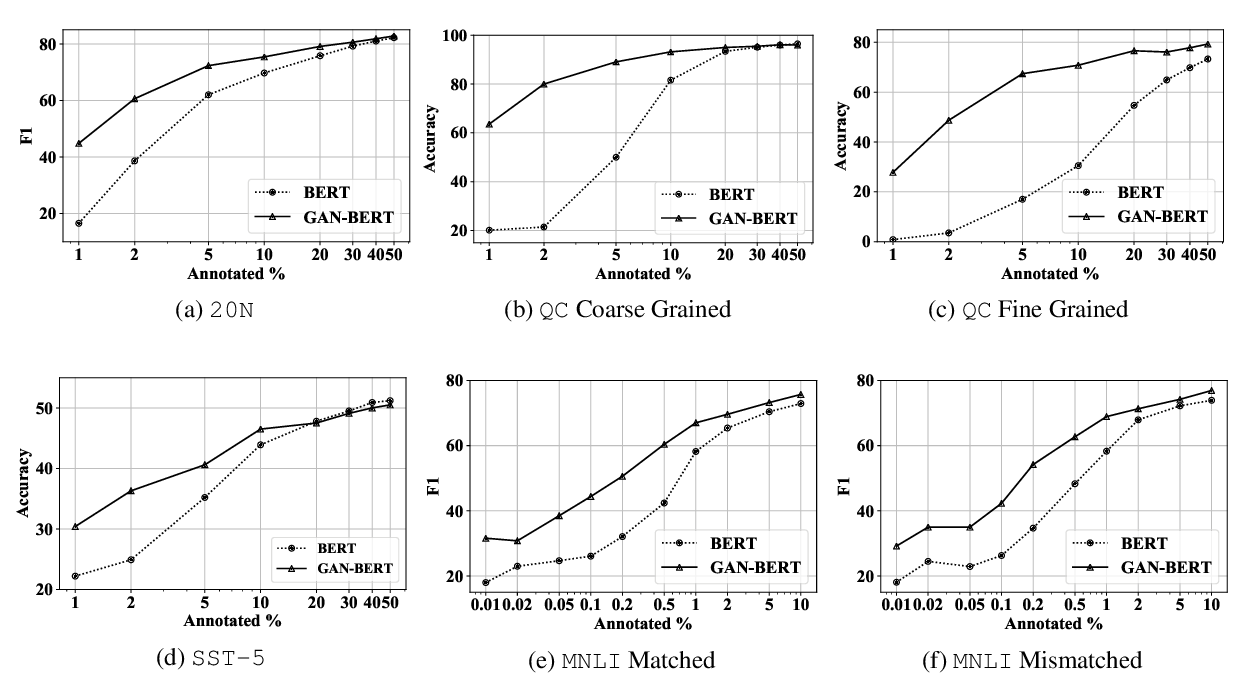