To Pretrain or Not to Pretrain: Examining the Benefits of Pretrainng on Resource Rich Tasks
Sinong Wang, Madian Khabsa, Hao Ma
Machine Learning for NLP Short Paper
Session 4A: Jul 6
(17:00-18:00 GMT)
Session 5A: Jul 6
(20:00-21:00 GMT)
Abstract:
Pretraining NLP models with variants of Masked Language Model (MLM) objectives has recently led to a significant improvements on many tasks. This paper examines the benefits of pretrained models as a function of the number of training samples used in the downstream task. On several text classification tasks, we show that as the number of training examples grow into the millions, the accuracy gap between finetuning BERT-based model and training vanilla LSTM from scratch narrows to within 1%. Our findings indicate that MLM-based models might reach a diminishing return point as the supervised data size increases significantly.
You can open the
pre-recorded video
in a separate window.
NOTE: The SlidesLive video may display a random order of the authors.
The correct author list is shown at the top of this webpage.
Similar Papers
Dice Loss for Data-imbalanced NLP Tasks
Xiaoya Li, Xiaofei Sun, Yuxian Meng, Junjun Liang, Fei Wu, Jiwei Li,
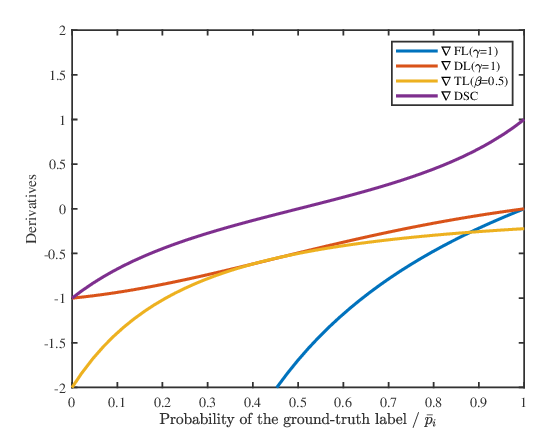
Distilling Knowledge Learned in BERT for Text Generation
Yen-Chun Chen, Zhe Gan, Yu Cheng, Jingzhou Liu, Jingjing Liu,
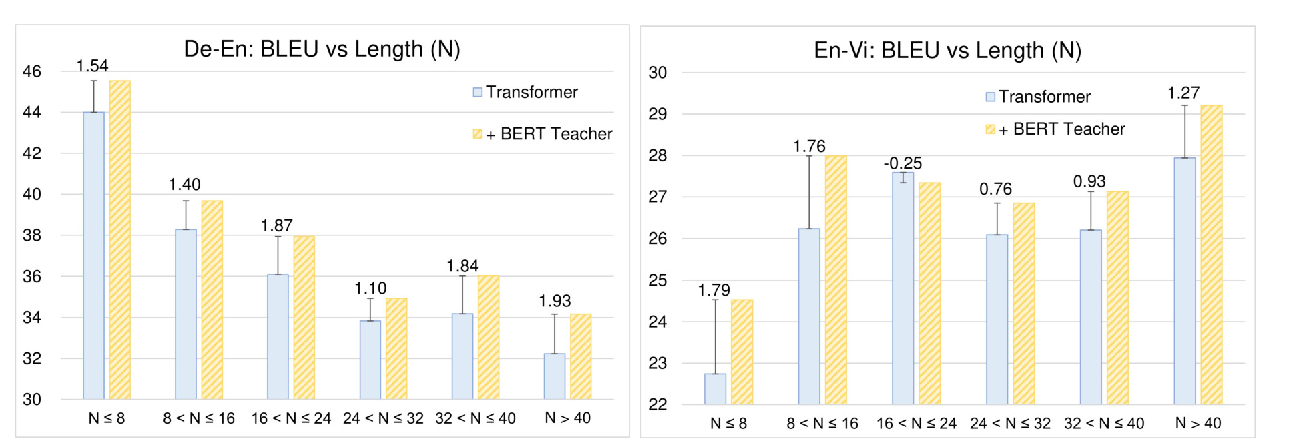
Probabilistically Masked Language Model Capable of Autoregressive Generation in Arbitrary Word Order
Yi Liao, Xin Jiang, Qun Liu,
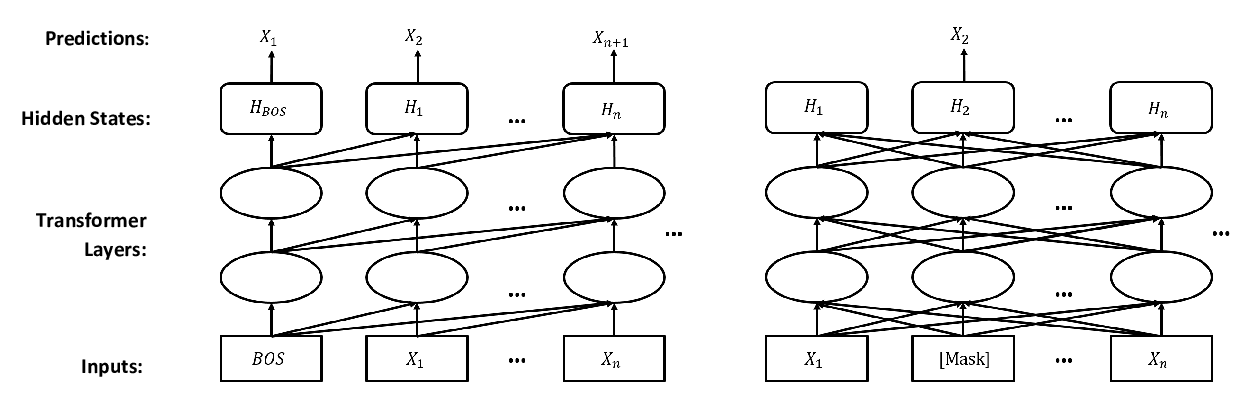
Fast and Accurate Deep Bidirectional Language Representations for Unsupervised Learning
Joongbo Shin, Yoonhyung Lee, Seunghyun Yoon, Kyomin Jung,
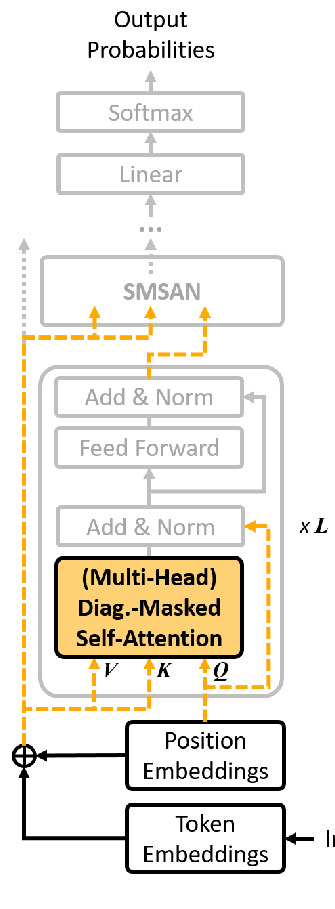