Abstract:
Masked language model and autoregressive language model are two types of language models. While pretrained masked language models such as BERT overwhelm the line of natural language understanding (NLU) tasks, autoregressive language models such as GPT are especially capable in natural language generation (NLG). In this paper, we propose a probabilistic masking scheme for the masked language model, which we call probabilistically masked language model (PMLM). We implement a specific PMLM with a uniform prior distribution on the masking ratio named u-PMLM. We prove that u-PMLM is equivalent to an autoregressive permutated language model. One main advantage of the model is that it supports text generation in arbitrary order with surprisingly good quality, which could potentially enable new applications over traditional unidirectional generation. Besides, the pretrained u-PMLM also outperforms BERT on a bunch of downstream NLU tasks.
You can open the
pre-recorded video
in a separate window.
NOTE: The SlidesLive video may display a random order of the authors.
The correct author list is shown at the top of this webpage.
Similar Papers
Distilling Knowledge Learned in BERT for Text Generation
Yen-Chun Chen, Zhe Gan, Yu Cheng, Jingzhou Liu, Jingjing Liu,
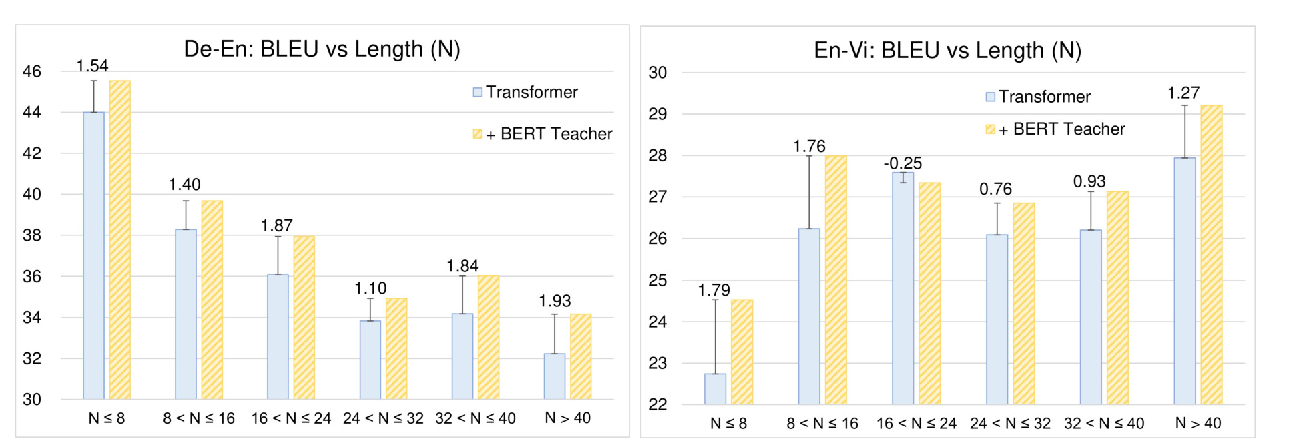
To Pretrain or Not to Pretrain: Examining the Benefits of Pretrainng on Resource Rich Tasks
Sinong Wang, Madian Khabsa, Hao Ma,
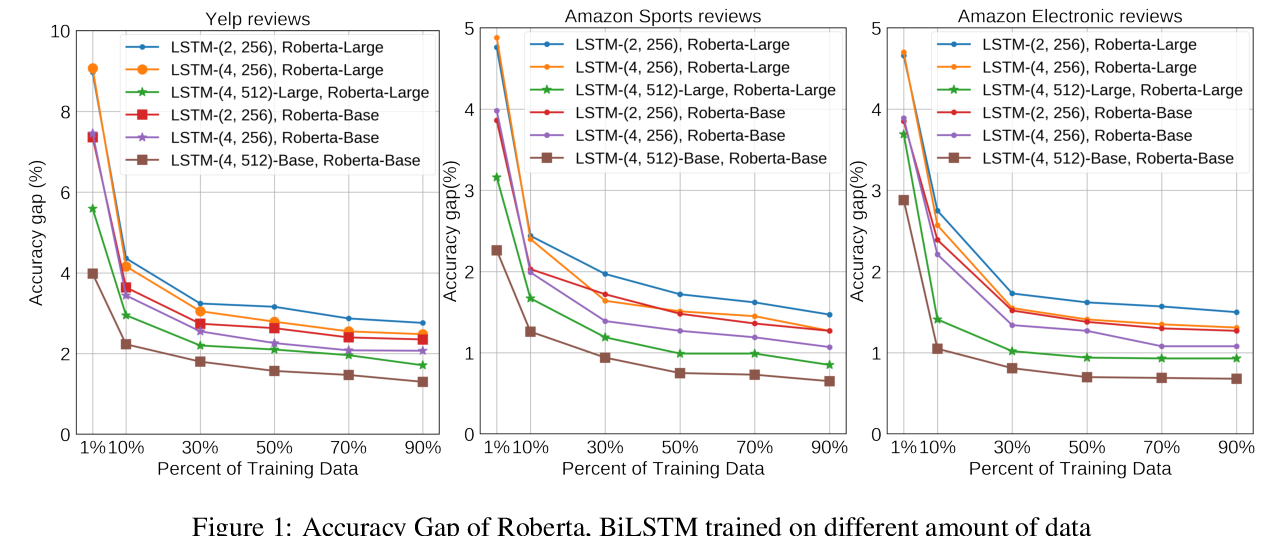
Jointly Masked Sequence-to-Sequence Model for Non-Autoregressive Neural Machine Translation
Junliang Guo, Linli Xu, Enhong Chen,
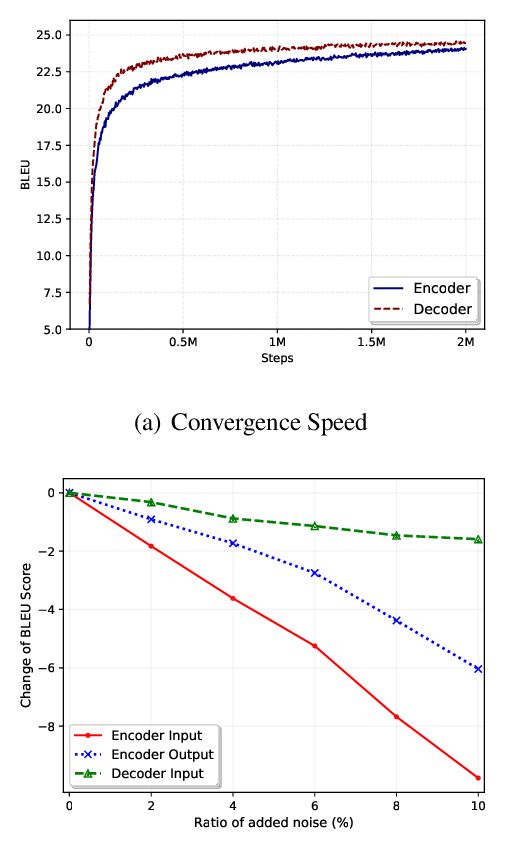
Curriculum Learning for Natural Language Understanding
Benfeng Xu, Licheng Zhang, Zhendong Mao, Quan Wang, Hongtao Xie, Yongdong Zhang,
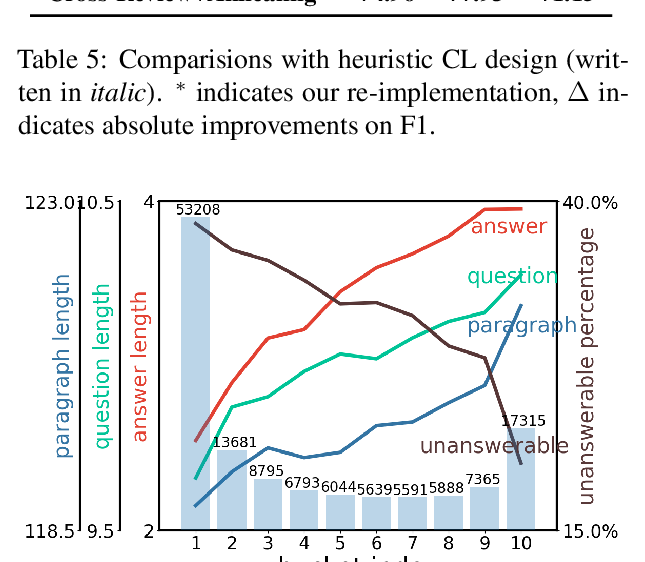