How does BERT's attention change when you fine-tune? An analysis methodology and a case study in negation scope
Yiyun Zhao, Steven Bethard
Interpretability and Analysis of Models for NLP Long Paper
Session 9A: Jul 7
(17:00-18:00 GMT)
Session 10A: Jul 7
(20:00-21:00 GMT)
Abstract:
Large pretrained language models like BERT, after fine-tuning to a downstream task, have achieved high performance on a variety of NLP problems. Yet explaining their decisions is difficult despite recent work probing their internal representations. We propose a procedure and analysis methods that take a hypothesis of how a transformer-based model might encode a linguistic phenomenon, and test the validity of that hypothesis based on a comparison between knowledge-related downstream tasks with downstream control tasks, and measurement of cross-dataset consistency. We apply this methodology to test BERT and RoBERTa on a hypothesis that some attention heads will consistently attend from a word in negation scope to the negation cue. We find that after fine-tuning BERT and RoBERTa on a negation scope task, the average attention head improves its sensitivity to negation and its attention consistency across negation datasets compared to the pre-trained models. However, only the base models (not the large models) improve compared to a control task, indicating there is evidence for a shallow encoding of negation only in the base models.
You can open the
pre-recorded video
in a separate window.
NOTE: The SlidesLive video may display a random order of the authors.
The correct author list is shown at the top of this webpage.
Similar Papers
Roles and Utilization of Attention Heads in Transformer-based Neural Language Models
Jae-young Jo, Sung-Hyon Myaeng,
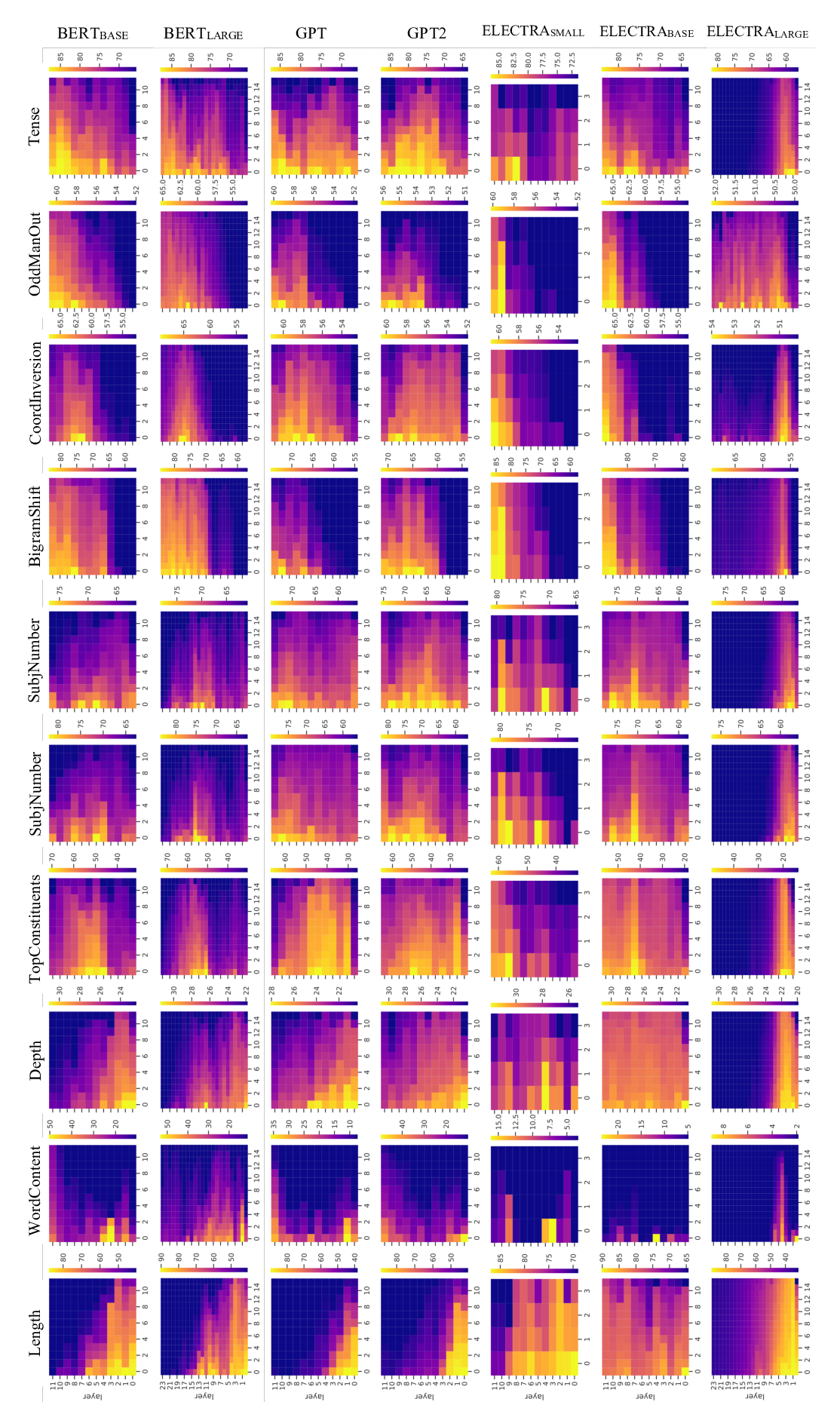
Perturbed Masking: Parameter-free Probing for Analyzing and Interpreting BERT
Zhiyong Wu, Yun Chen, Ben Kao, Qun Liu,
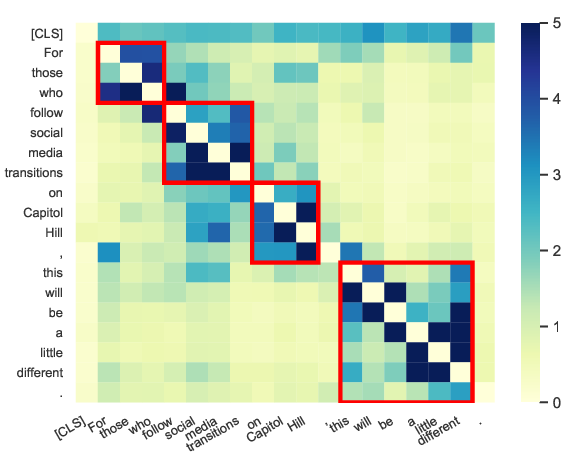
Predicting the Focus of Negation: Model and Error Analysis
Md Mosharaf Hossain, Kathleen Hamilton, Alexis Palmer, Eduardo Blanco,
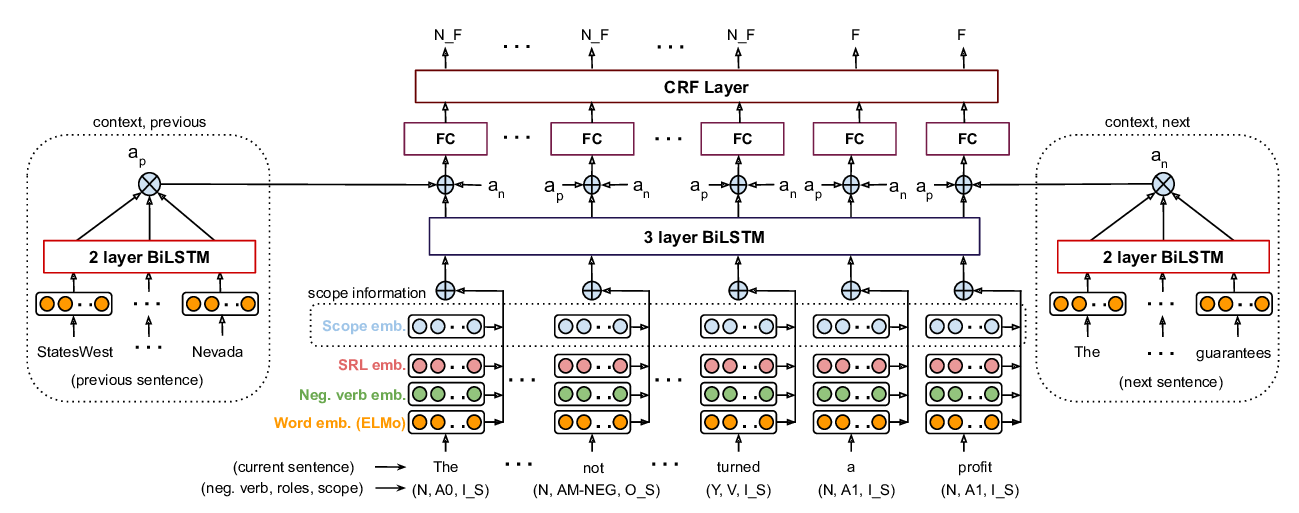
Syntactic Data Augmentation Increases Robustness to Inference Heuristics
Junghyun Min, R. Thomas McCoy, Dipanjan Das, Emily Pitler, Tal Linzen,
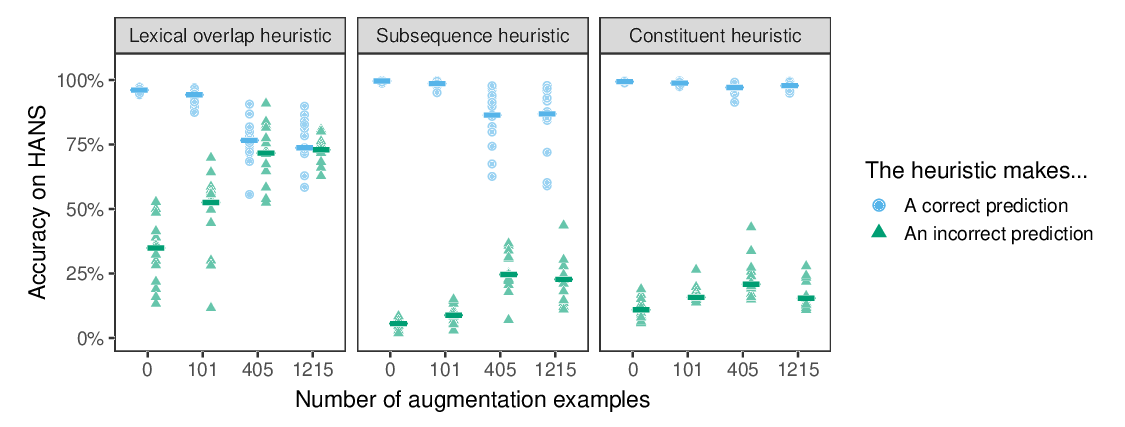