Improved Speech Representations with Multi-Target Autoregressive Predictive Coding
Yu-An Chung, James Glass
Speech and Multimodality Short Paper
Session 4A: Jul 6
(17:00-18:00 GMT)
Session 5A: Jul 6
(20:00-21:00 GMT)
Abstract:
Training objectives based on predictive coding have recently been shown to be very effective at learning meaningful representations from unlabeled speech. One example is Autoregressive Predictive Coding (Chung et al., 2019), which trains an autoregressive RNN to generate an unseen future frame given a context such as recent past frames. The basic hypothesis of these approaches is that hidden states that can accurately predict future frames are a useful representation for many downstream tasks. In this paper we extend this hypothesis and aim to enrich the information encoded in the hidden states by training the model to make more accurate future predictions. We propose an auxiliary objective that serves as a regularization to improve generalization of the future frame prediction task. Experimental results on phonetic classification, speech recognition, and speech translation not only support the hypothesis, but also demonstrate the effectiveness of our approach in learning representations that contain richer phonetic content.
You can open the
pre-recorded video
in a separate window.
NOTE: The SlidesLive video may display a random order of the authors.
The correct author list is shown at the top of this webpage.
Similar Papers
Towards end-2-end learning for predicting behavior codes from spoken utterances in psychotherapy conversations
Karan Singla, Zhuohao Chen, David Atkins, Shrikanth Narayanan,
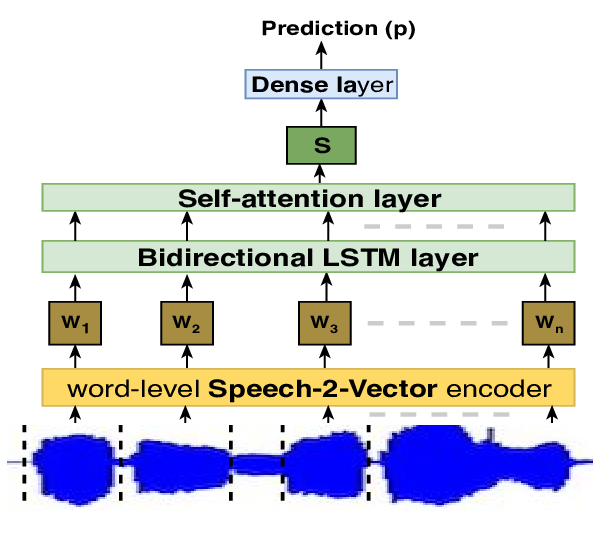
Learning Constraints for Structured Prediction Using Rectifier Networks
Xingyuan Pan, Maitrey Mehta, Vivek Srikumar,
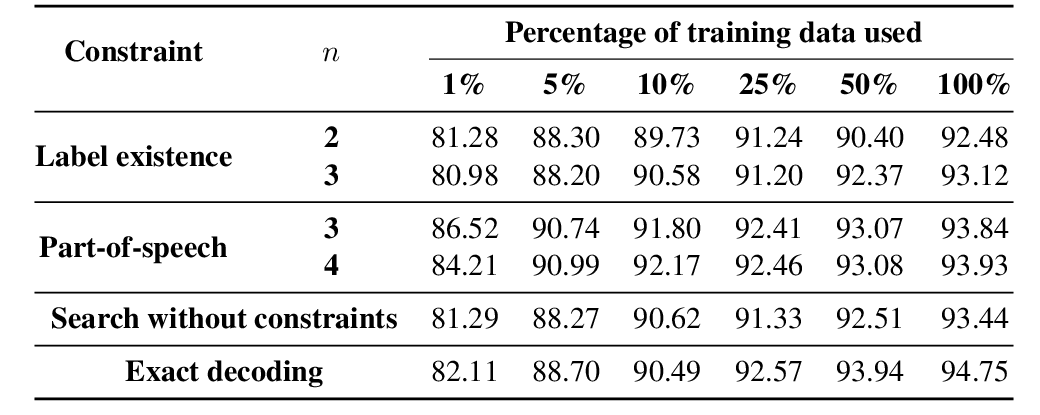
Learning to Understand Child-directed and Adult-directed Speech
Lieke Gelderloos, Grzegorz Chrupała, Afra Alishahi,
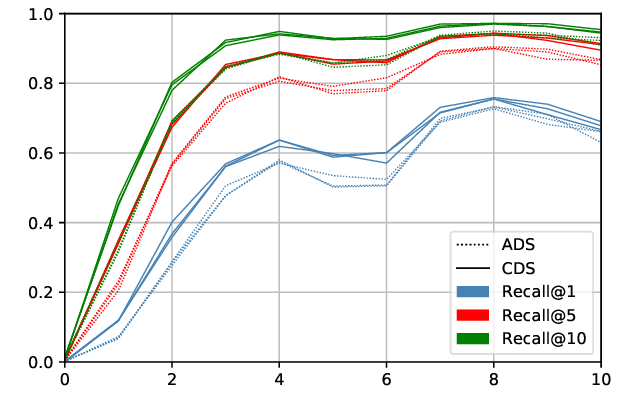