Towards end-2-end learning for predicting behavior codes from spoken utterances in psychotherapy conversations
Karan Singla, Zhuohao Chen, David Atkins, Shrikanth Narayanan
Speech and Multimodality Short Paper
Session 6B: Jul 7
(06:00-07:00 GMT)
Session 10B: Jul 7
(21:00-22:00 GMT)
Abstract:
Spoken language understanding tasks usually rely on pipelines involving complex processing blocks such as voice activity detection, speaker diarization and Automatic speech recognition (ASR). We propose a novel framework for predicting utterance level labels directly from speech features, thus removing the dependency on first generating transcripts, and transcription free behavioral coding. Our classifier uses a pretrained Speech-2-Vector encoder as bottleneck to generate word-level representations from speech features. This pretrained encoder learns to encode speech features for a word using an objective similar to Word2Vec. Our proposed approach just uses speech features and word segmentation information for predicting spoken utterance-level target labels. We show that our model achieves competitive results to other state-of-the-art approaches which use transcribed text for the task of predicting psychotherapy-relevant behavior codes.
You can open the
pre-recorded video
in a separate window.
NOTE: The SlidesLive video may display a random order of the authors.
The correct author list is shown at the top of this webpage.
Similar Papers
Investigating the effect of auxiliary objectives for the automated grading of learner English speech transcriptions
Hannah Craighead, Andrew Caines, Paula Buttery, Helen Yannakoudakis,
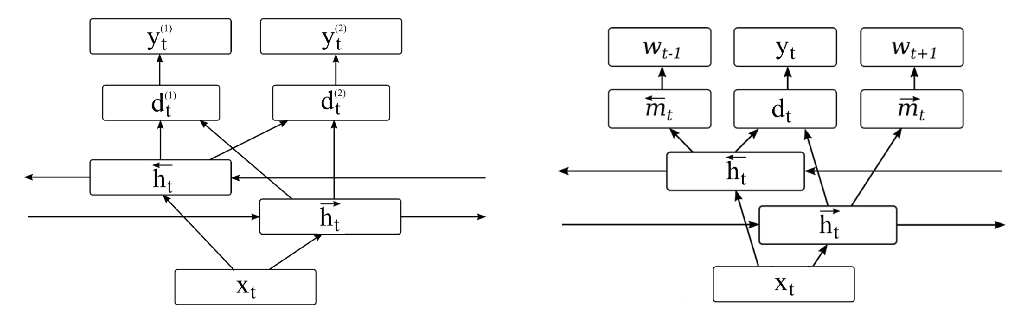
Curriculum Pre-training for End-to-End Speech Translation
Chengyi Wang, Yu Wu, Shujie Liu, Ming Zhou, Zhenglu Yang,
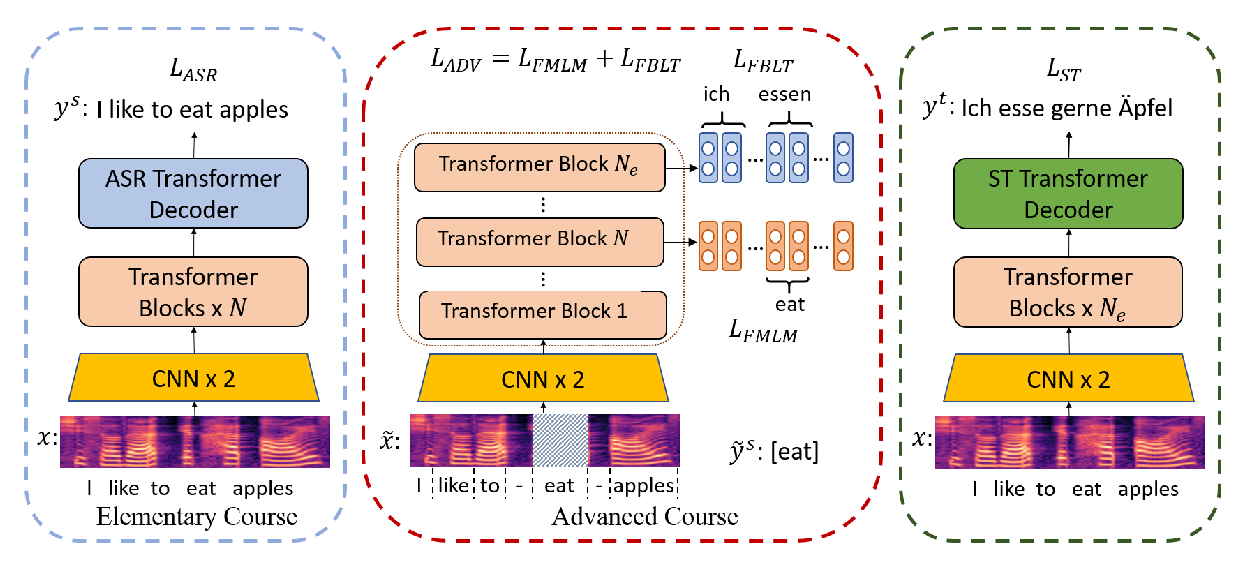
MultiQT: Multimodal learning for real-time question tracking in speech
Jakob D. Havtorn, Jan Latko, Joakim Edin, Lars Maaløe, Lasse Borgholt, Lorenzo Belgrano, Nicolai Jacobsen, Regitze Sdun, Željko Agić,
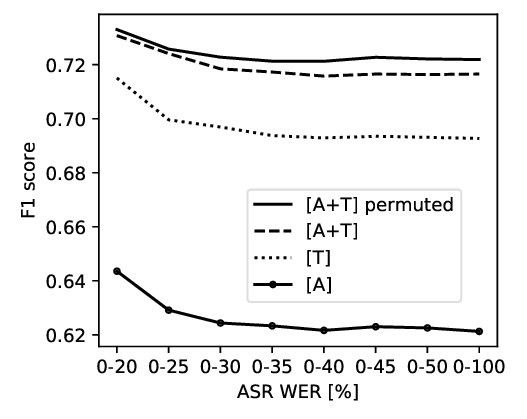
Learning to Understand Child-directed and Adult-directed Speech
Lieke Gelderloos, Grzegorz Chrupała, Afra Alishahi,
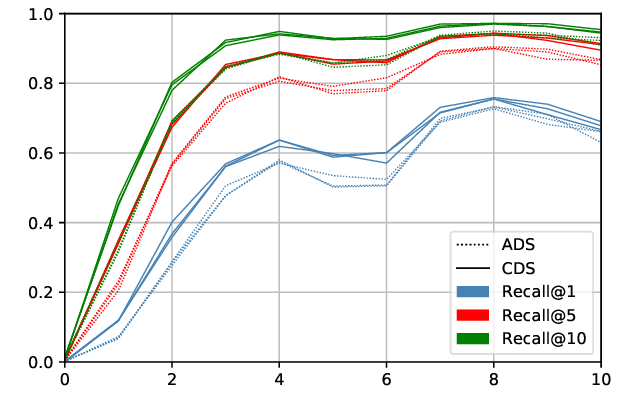