Multimodal and Multiresolution Speech Recognition with Transformers
Georgios Paraskevopoulos, Srinivas Parthasarathy, Aparna Khare, Shiva Sundaram
Speech and Multimodality Short Paper
Session 4A: Jul 6
(17:00-18:00 GMT)
Session 5A: Jul 6
(20:00-21:00 GMT)
Abstract:
This paper presents an audio visual automatic speech recognition (AV-ASR) system using a Transformer-based architecture. We particularly focus on the scene context provided by the visual information, to ground the ASR. We extract representations for audio features in the encoder layers of the transformer and fuse video features using an additional crossmodal multihead attention layer. Additionally, we incorporate a multitask training criterion for multiresolution ASR, where we train the model to generate both character and subword level transcriptions. Experimental results on the How2 dataset, indicate that multiresolution training can speed up convergence by around 50% and relatively improves word error rate (WER) performance by upto 18% over subword prediction models. Further, incorporating visual information improves performance with relative gains upto 3.76% over audio only models. Our results are comparable to state-of-the-art Listen, Attend and Spell-based architectures.
You can open the
pre-recorded video
in a separate window.
NOTE: The SlidesLive video may display a random order of the authors.
The correct author list is shown at the top of this webpage.
Similar Papers
A Novel Graph-based Multi-modal Fusion Encoder for Neural Machine Translation
Yongjing Yin, Fandong Meng, Jinsong Su, Chulun Zhou, Zhengyuan Yang, Jie Zhou, Jiebo Luo,
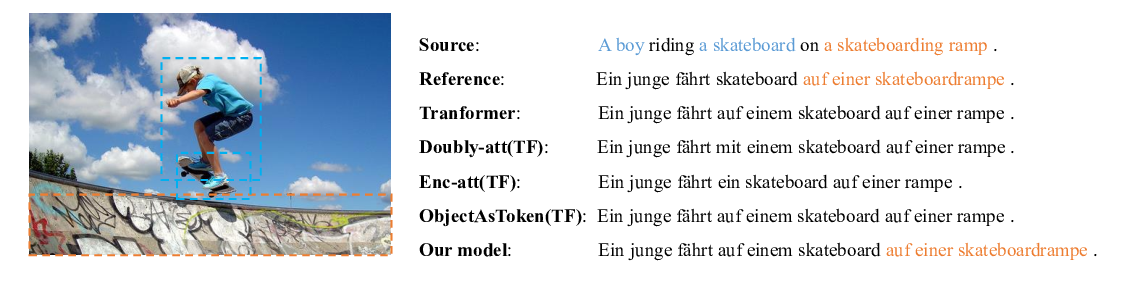
Lipschitz Constrained Parameter Initialization for Deep Transformers
Hongfei Xu, Qiuhui Liu, Josef van Genabith, Deyi Xiong, Jingyi Zhang,
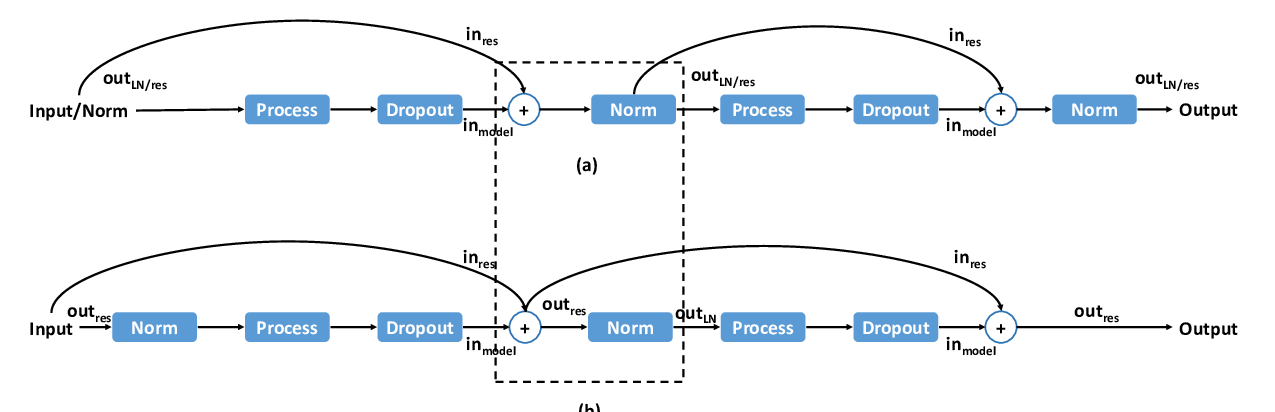
Cross-Modality Relevance for Reasoning on Language and Vision
Chen Zheng, Quan Guo, Parisa Kordjamshidi,
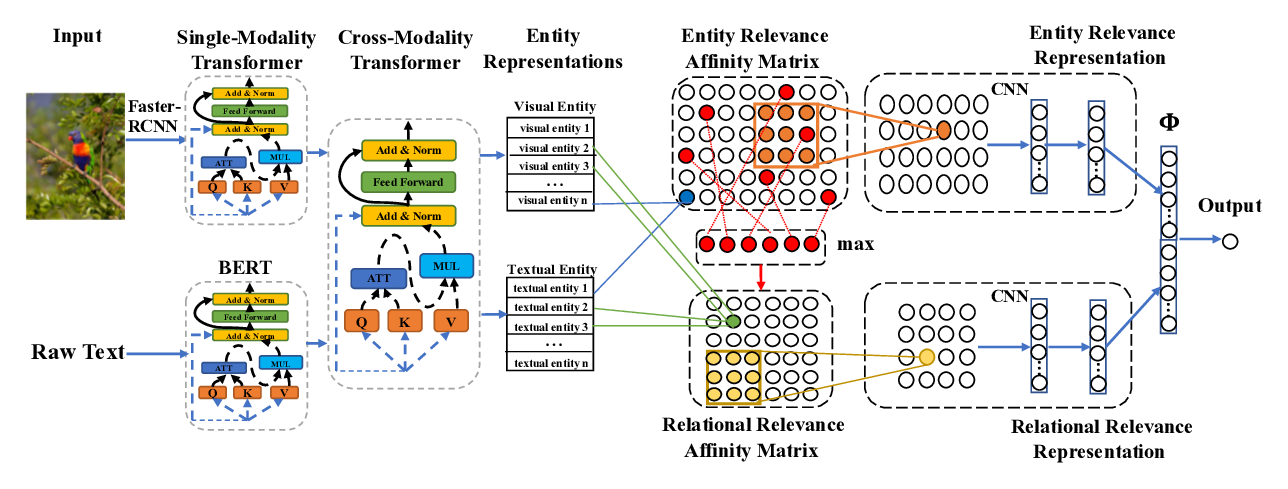
Transformers to Learn Hierarchical Contexts in Multiparty Dialogue for Span-based Question Answering
Changmao Li, Jinho D. Choi,
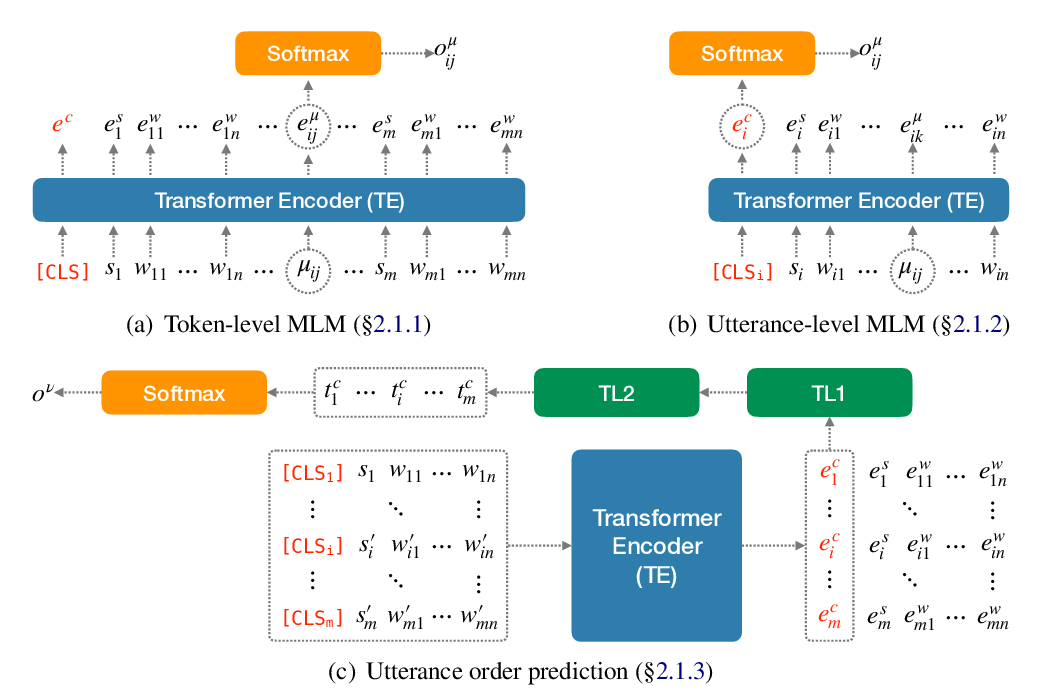