Transformers to Learn Hierarchical Contexts in Multiparty Dialogue for Span-based Question Answering
Changmao Li, Jinho D. Choi
Question Answering Short Paper
Session 9B: Jul 7
(18:00-19:00 GMT)
Session 10B: Jul 7
(21:00-22:00 GMT)
Abstract:
We introduce a novel approach to transformers that learns hierarchical representations in multiparty dialogue. First, three language modeling tasks are used to pre-train the transformers, token- and utterance-level language modeling and utterance order prediction, that learn both token and utterance embeddings for better understanding in dialogue contexts. Then, multi-task learning between the utterance prediction and the token span prediction is applied to fine-tune for span-based question answering (QA). Our approach is evaluated on the FriendsQA dataset and shows improvements of 3.8% and 1.4% over the two state-of-the-art transformer models, BERT and RoBERTa, respectively.
You can open the
pre-recorded video
in a separate window.
NOTE: The SlidesLive video may display a random order of the authors.
The correct author list is shown at the top of this webpage.
Similar Papers
SpanBERT: Improving Pre-training by Representing and Predicting Spans
Mandar Joshi, Danqi Chen, Yinhan Liu, Daniel S. Weld, Luke Zettlemoyer, Omer Levy,
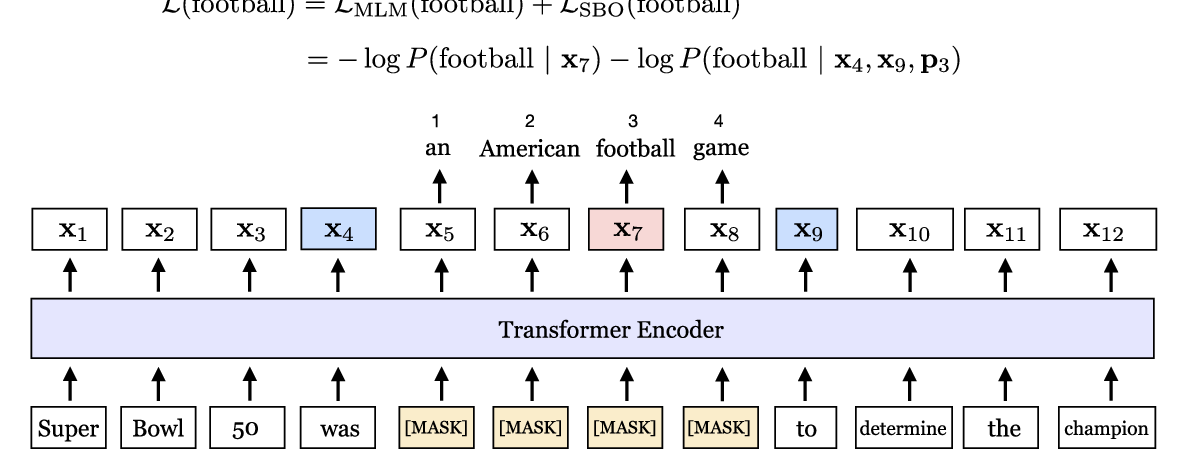
Span Selection Pre-training for Question Answering
Michael Glass, Alfio Gliozzo, Rishav Chakravarti, Anthony Ferritto, Lin Pan, G P Shrivatsa Bhargav, Dinesh Garg, Avi Sil,
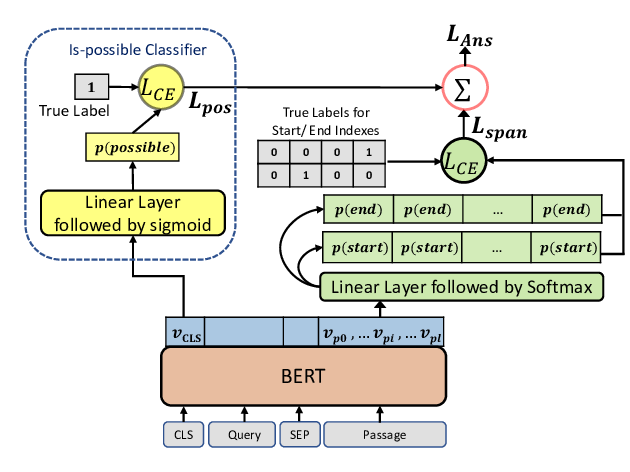
Multimodal and Multiresolution Speech Recognition with Transformers
Georgios Paraskevopoulos, Srinivas Parthasarathy, Aparna Khare, Shiva Sundaram,
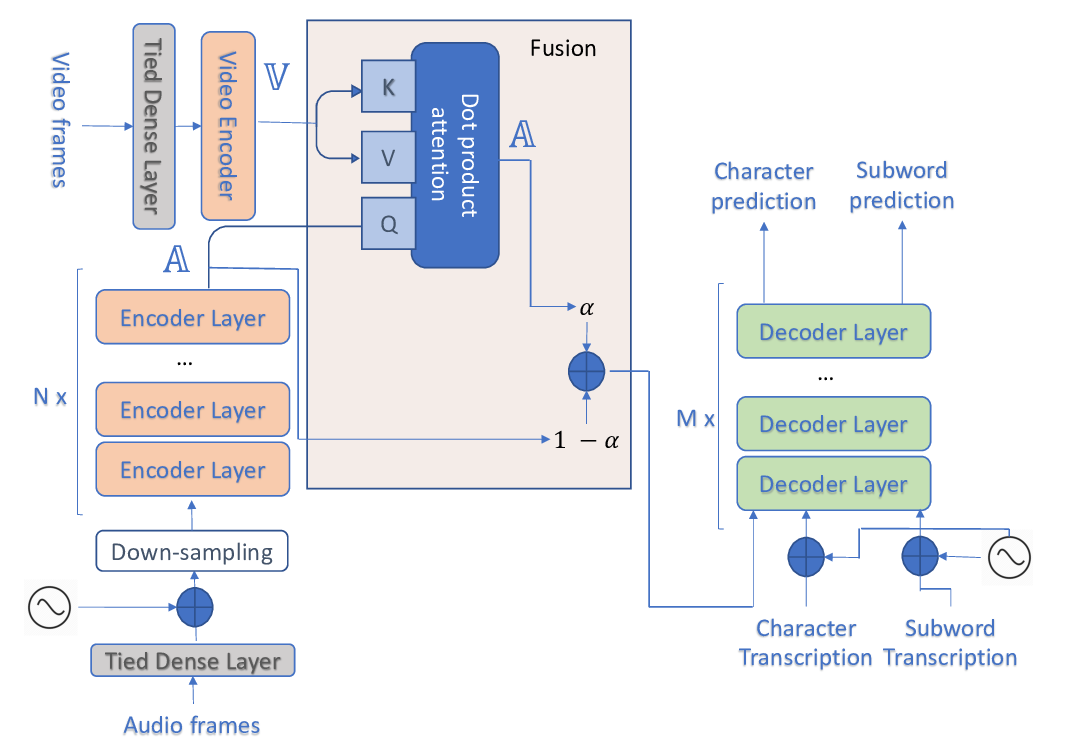