Bridging the Structural Gap Between Encoding and Decoding for Data-To-Text Generation
Chao Zhao, Marilyn Walker, Snigdha Chaturvedi
Generation Long Paper
Session 4B: Jul 6
(18:00-19:00 GMT)
Session 5A: Jul 6
(20:00-21:00 GMT)
Abstract:
Generating sequential natural language descriptions from graph-structured data (e.g., knowledge graph) is challenging, partly because of the structural differences between the input graph and the output text. Hence, popular sequence-to-sequence models, which require serialized input, are not a natural fit for this task. Graph neural networks, on the other hand, can better encode the input graph but broaden the structural gap between the encoder and decoder, making faithful generation difficult. To narrow this gap, we propose DualEnc, a dual encoding model that can not only incorporate the graph structure, but can also cater to the linear structure of the output text. Empirical comparisons with strong single-encoder baselines demonstrate that dual encoding can significantly improve the quality of the generated text.
You can open the
pre-recorded video
in a separate window.
NOTE: The SlidesLive video may display a random order of the authors.
The correct author list is shown at the top of this webpage.
Similar Papers
Line Graph Enhanced AMR-to-Text Generation with Mix-Order Graph Attention Networks
Yanbin Zhao, Lu Chen, Zhi Chen, Ruisheng Cao, Su Zhu, Kai Yu,
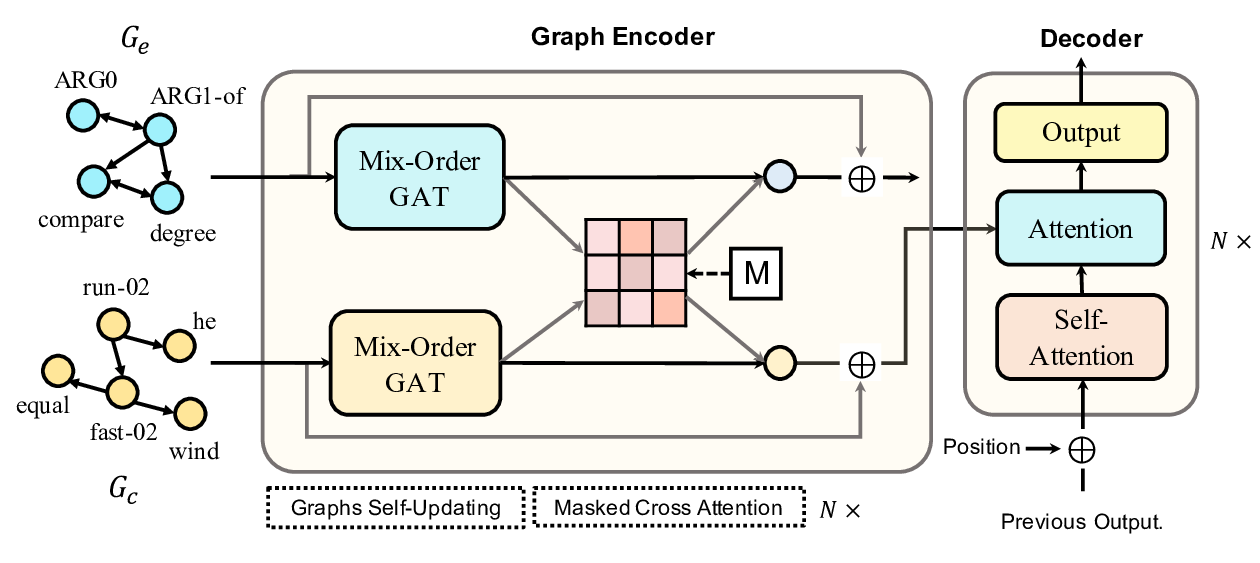
Heterogeneous Graph Transformer for Graph-to-Sequence Learning
Shaowei Yao, Tianming Wang, Xiaojun Wan,
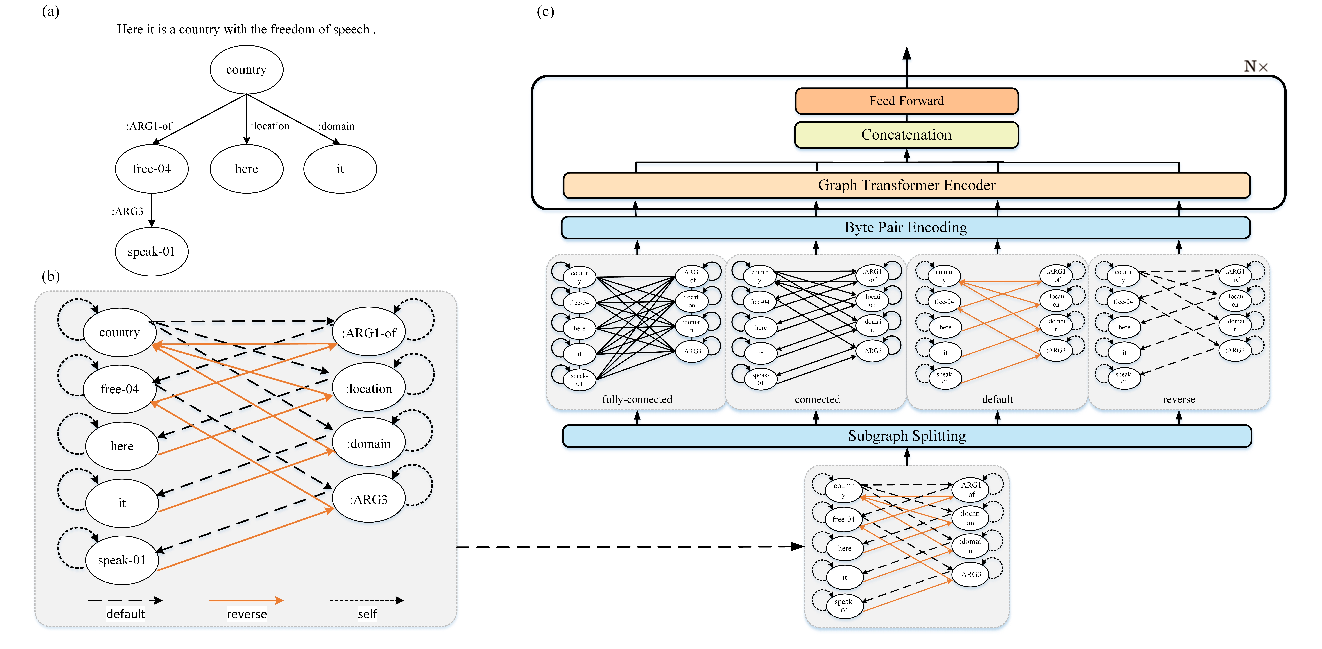
Structural Information Preserving for Graph-to-Text Generation
Linfeng Song, Ante Wang, Jinsong Su, Yue Zhang, Kun Xu, Yubin Ge, Dong Yu,
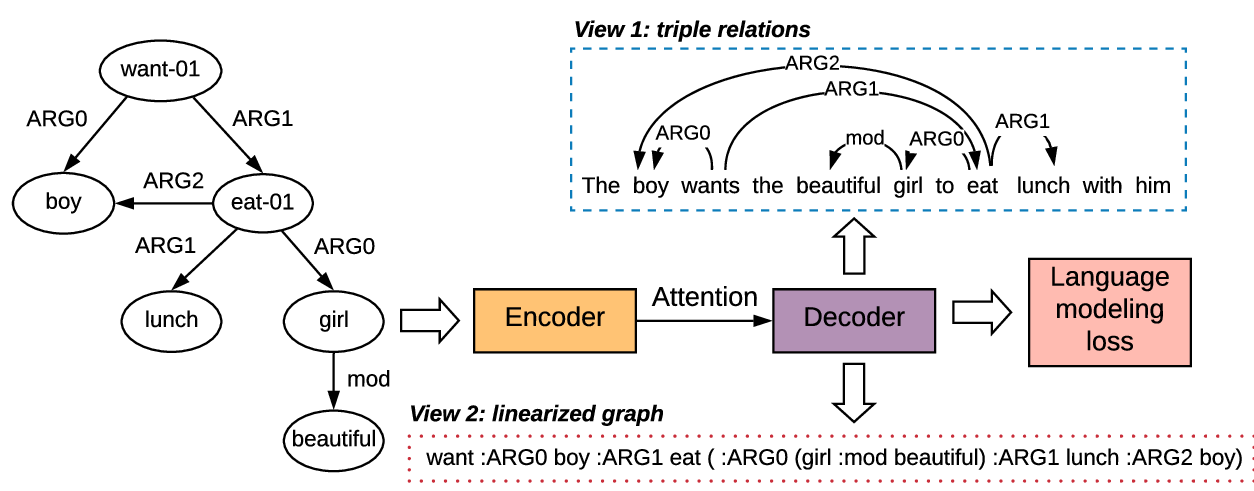
LogicalFactChecker: Leveraging Logical Operations for Fact Checking with Graph Module Network
Wanjun Zhong, Duyu Tang, Zhangyin Feng, Nan Duan, Ming Zhou, Ming Gong, Linjun Shou, Daxin Jiang, Jiahai Wang, Jian Yin,
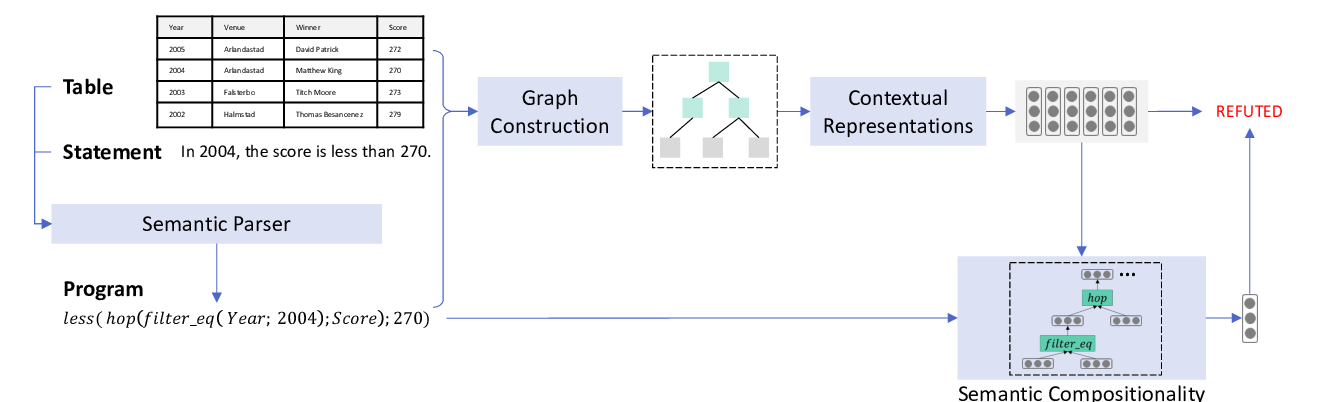