Heterogeneous Graph Transformer for Graph-to-Sequence Learning
Shaowei Yao, Tianming Wang, Xiaojun Wan
Generation Long Paper
Session 12B: Jul 8
(09:00-10:00 GMT)
Session 13A: Jul 8
(12:00-13:00 GMT)
Abstract:
The graph-to-sequence (Graph2Seq) learning aims to transduce graph-structured representations to word sequences for text generation. Recent studies propose various models to encode graph structure. However, most previous works ignore the indirect relations between distance nodes, or treat indirect relations and direct relations in the same way. In this paper, we propose the Heterogeneous Graph Transformer to independently model the different relations in the individual subgraphs of the original graph, including direct relations, indirect relations and multiple possible relations between nodes. Experimental results show that our model strongly outperforms the state of the art on all four standard benchmarks of AMR-to-text generation and syntax-based neural machine translation.
You can open the
pre-recorded video
in a separate window.
NOTE: The SlidesLive video may display a random order of the authors.
The correct author list is shown at the top of this webpage.
Similar Papers
Line Graph Enhanced AMR-to-Text Generation with Mix-Order Graph Attention Networks
Yanbin Zhao, Lu Chen, Zhi Chen, Ruisheng Cao, Su Zhu, Kai Yu,
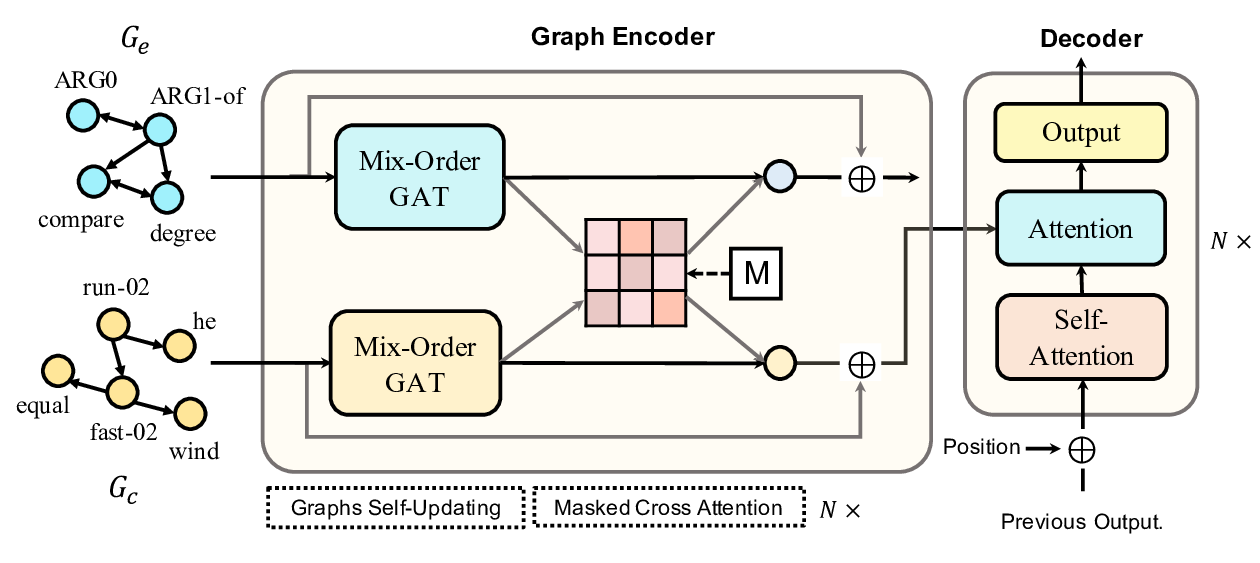
Orthogonal Relation Transforms with Graph Context Modeling for Knowledge Graph Embedding
Yun Tang, Jing Huang, Guangtao Wang, Xiaodong He, Bowen Zhou,
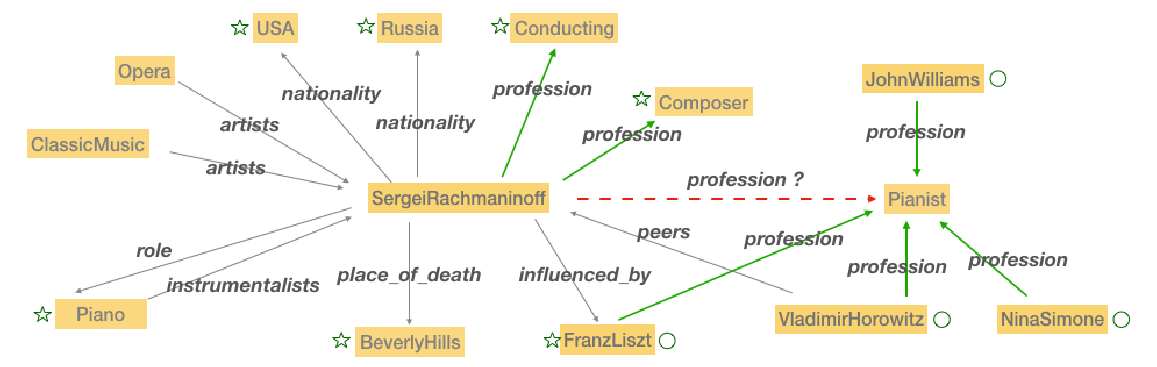
Bridging the Structural Gap Between Encoding and Decoding for Data-To-Text Generation
Chao Zhao, Marilyn Walker, Snigdha Chaturvedi,
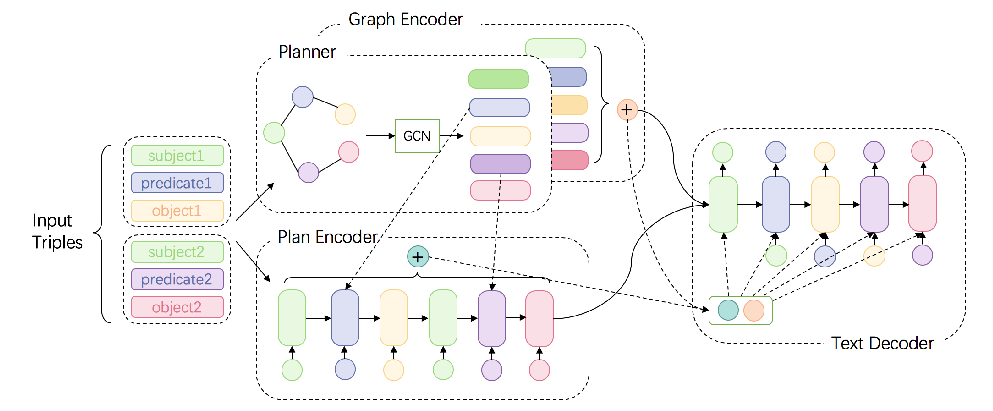