INSET: Sentence Infilling with INter-SEntential Transformer
Yichen Huang, Yizhe Zhang, Oussama Elachqar, Yu Cheng
Generation Long Paper
Session 4B: Jul 6
(18:00-19:00 GMT)
Session 5A: Jul 6
(20:00-21:00 GMT)
Abstract:
Missing sentence generation (or sentence in-filling) fosters a wide range of applications in natural language generation, such as document auto-completion and meeting note expansion. This task asks the model to generate intermediate missing sentences that can syntactically and semantically bridge the surrounding context. Solving the sentence infilling task requires techniques in natural language processing ranging from understanding to discourse-level planning to generation. In this paper, we propose a framework to decouple the challenge and address these three aspects respectively, leveraging the power of existing large-scale pre-trained models such as BERT and GPT-2. We empirically demonstrate the effectiveness of our model in learning a sentence representation for generation and further generating a missing sentence that fits the context.
You can open the
pre-recorded video
in a separate window.
NOTE: The SlidesLive video may display a random order of the authors.
The correct author list is shown at the top of this webpage.
Similar Papers
Distilling Knowledge Learned in BERT for Text Generation
Yen-Chun Chen, Zhe Gan, Yu Cheng, Jingzhou Liu, Jingjing Liu,
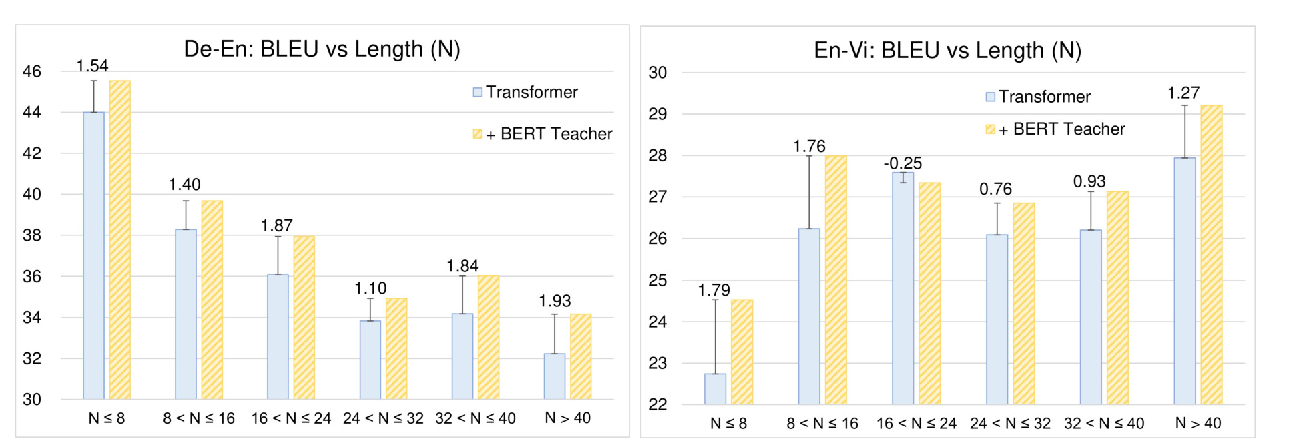
PLATO: Pre-trained Dialogue Generation Model with Discrete Latent Variable
Siqi Bao, Huang He, Fan Wang, Hua Wu, Haifeng Wang,
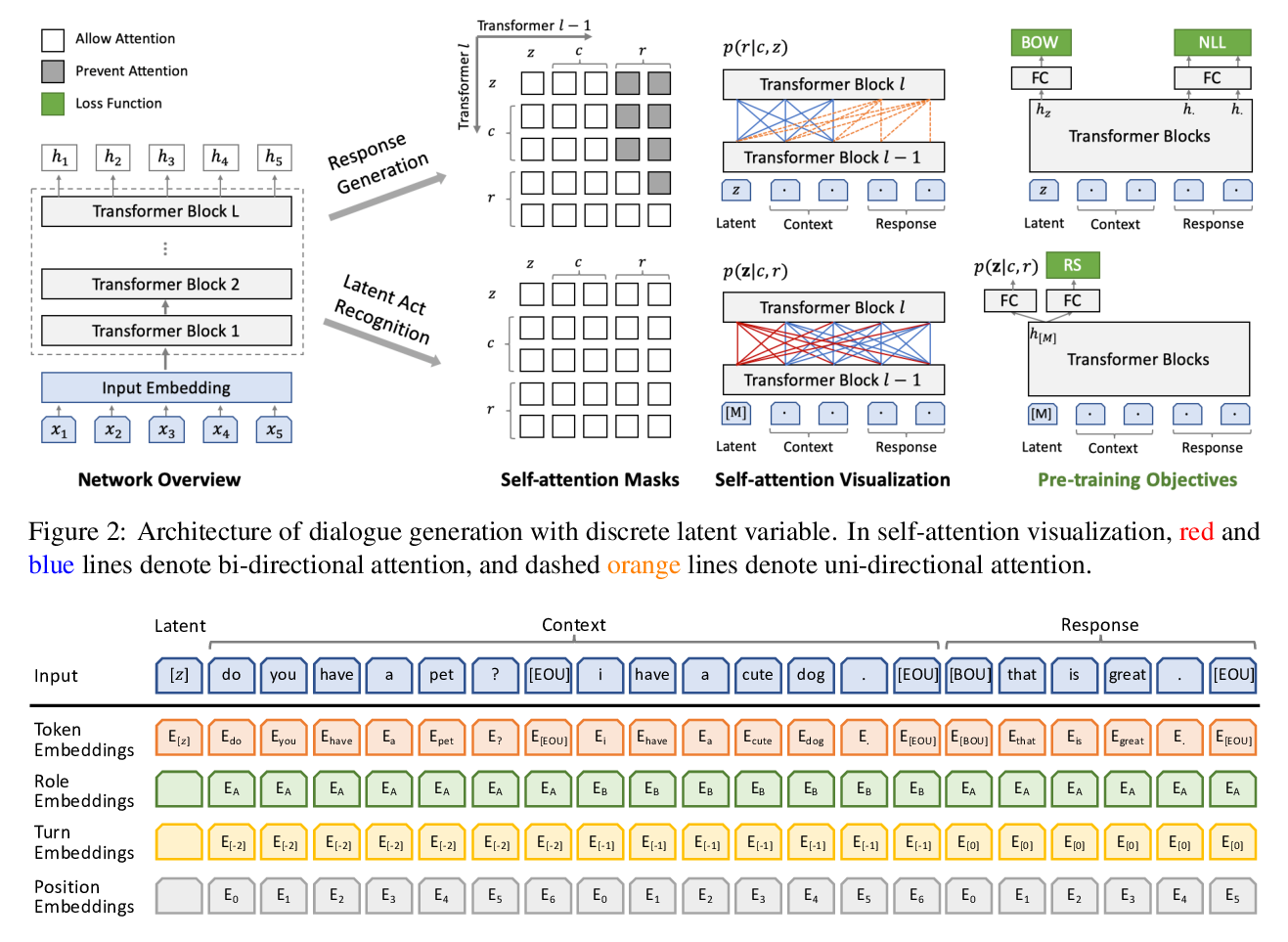
Toward Better Storylines with Sentence-Level Language Models
Daphne Ippolito, David Grangier, Douglas Eck, Chris Callison-Burch,
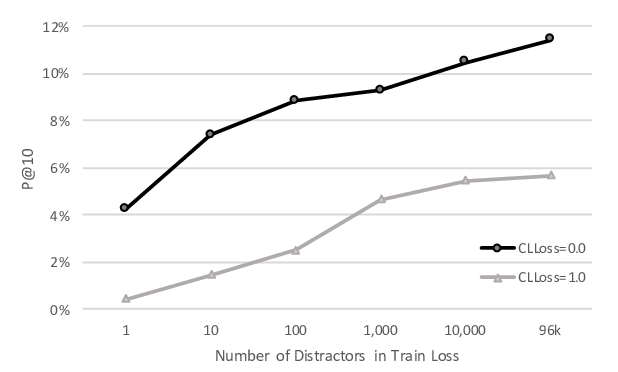
Diverse and Informative Dialogue Generation with Context-Specific Commonsense Knowledge Awareness
Sixing Wu, Ying Li, Dawei Zhang, Yang Zhou, Zhonghai Wu,
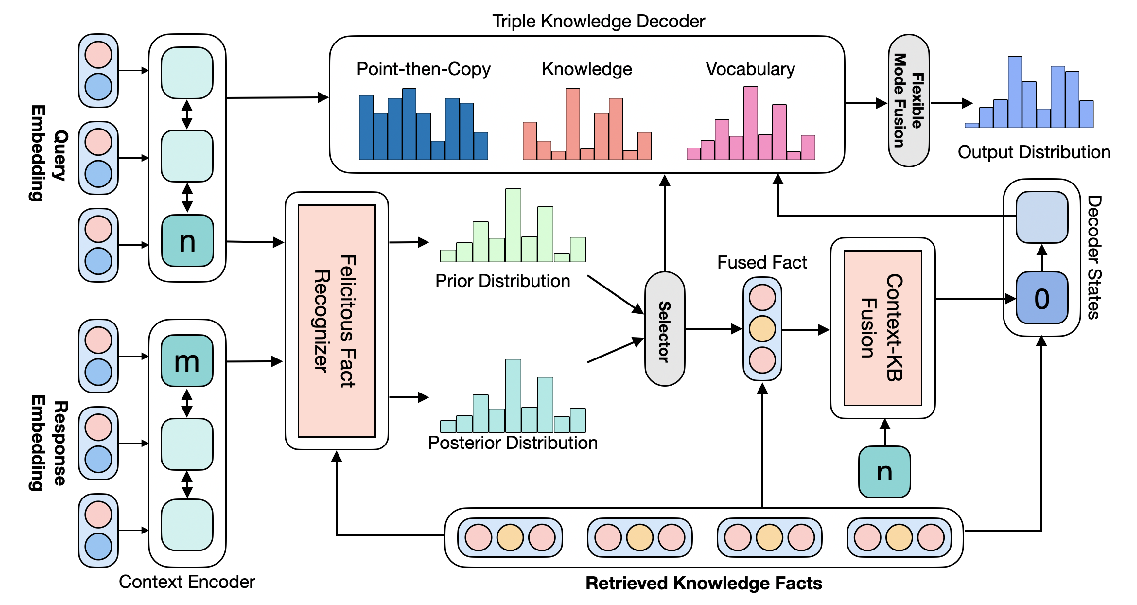