Toward Better Storylines with Sentence-Level Language Models
Daphne Ippolito, David Grangier, Douglas Eck, Chris Callison-Burch
Generation Short Paper
Session 13A: Jul 8
(12:00-13:00 GMT)
Session 15B: Jul 8
(21:00-22:00 GMT)
Abstract:
We propose a sentence-level language model which selects the next sentence in a story from a finite set of fluent alternatives. Since it does not need to model fluency, the sentence-level language model can focus on longer range dependencies, which are crucial for multi-sentence coherence. Rather than dealing with individual words, our method treats the story so far as a list of pre-trained sentence embeddings and predicts an embedding for the next sentence, which is more efficient than predicting word embeddings. Notably this allows us to consider a large number of candidates for the next sentence during training. We demonstrate the effectiveness of our approach with state-of-the-art accuracy on the unsupervised Story Cloze task and with promising results on larger-scale next sentence prediction tasks.
You can open the
pre-recorded video
in a separate window.
NOTE: The SlidesLive video may display a random order of the authors.
The correct author list is shown at the top of this webpage.
Similar Papers
INSET: Sentence Infilling with INter-SEntential Transformer
Yichen Huang, Yizhe Zhang, Oussama Elachqar, Yu Cheng,
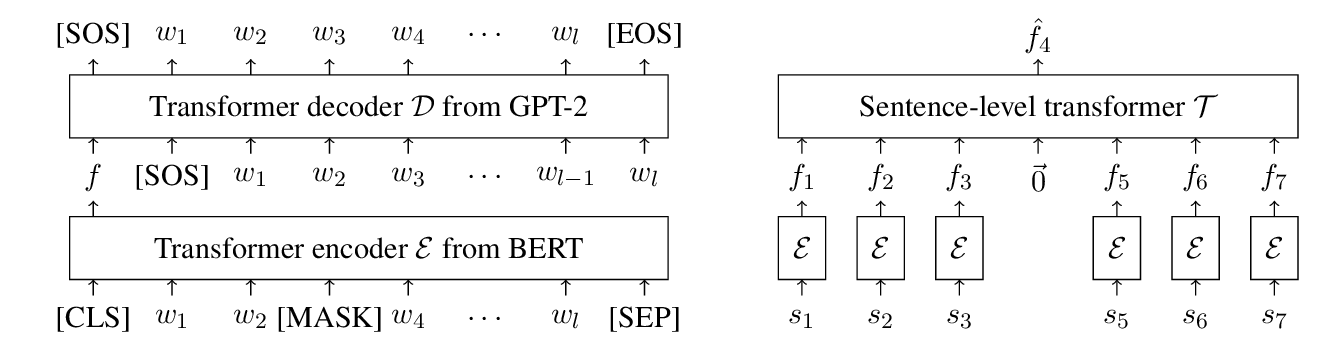
A Frame-based Sentence Representation for Machine Reading Comprehension
Shaoru Guo, Ru Li, Hongye Tan, Xiaoli Li, Yong Guan, Hongyan Zhao, Yueping Zhang,
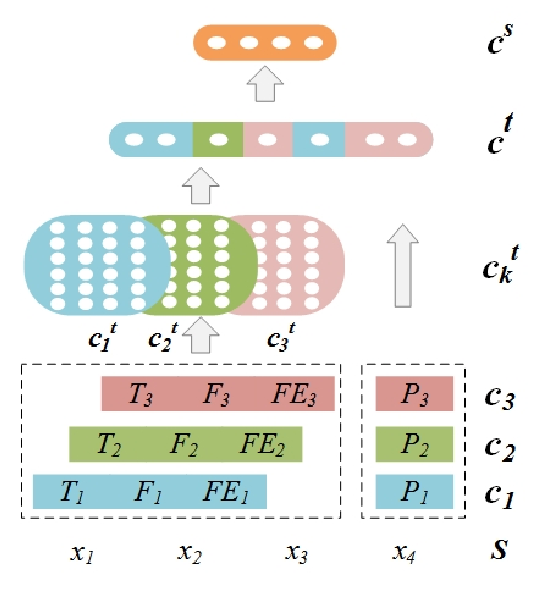
Towards Debiasing Sentence Representations
Paul Pu Liang, Irene Mengze Li, Emily Zheng, Yao Chong Lim, Ruslan Salakhutdinov, Louis-Philippe Morency,
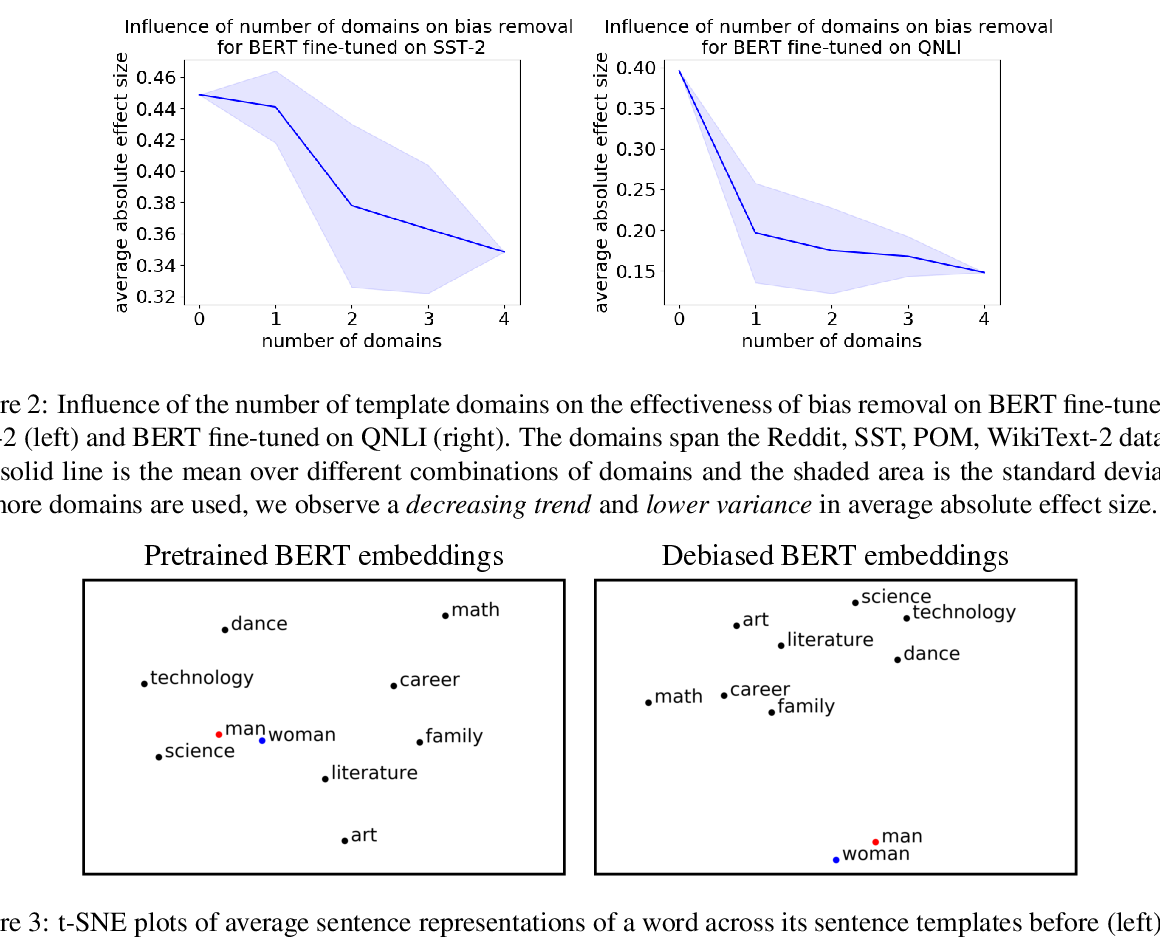
Leveraging Pre-trained Checkpoints for Sequence Generation Tasks
Sascha Rothe, Shashi Narayan and Aliaksei Severyn,
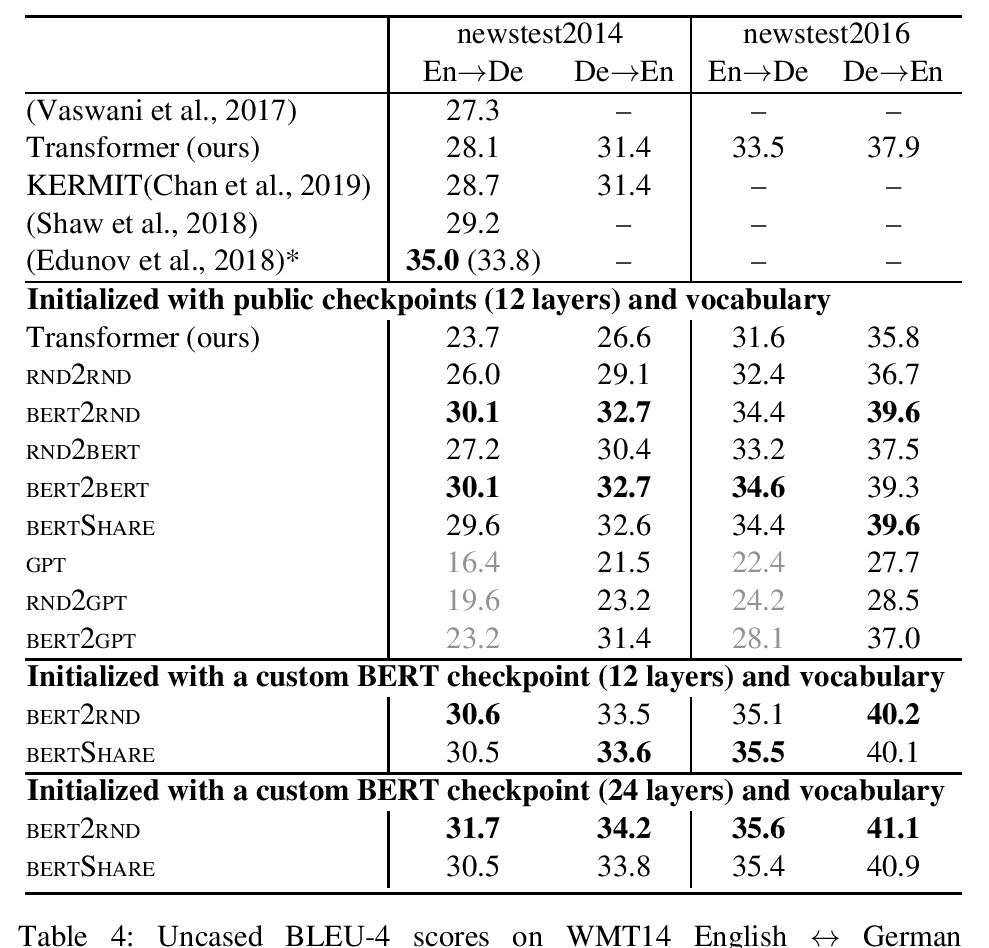