Geometry-aware domain adaptation for unsupervised alignment of word embeddings
Pratik Jawanpuria, Mayank Meghwanshi, Bamdev Mishra
Machine Translation Short Paper
Session 6A: Jul 7
(05:00-06:00 GMT)
Session 8A: Jul 7
(12:00-13:00 GMT)
Abstract:
We propose a novel manifold based geometric approach for learning unsupervised alignment of word embeddings between the source and the target languages. Our approach formulates the alignment learning problem as a domain adaptation problem over the manifold of doubly stochastic matrices. This viewpoint arises from the aim to align the second order information of the two language spaces. The rich geometry of the doubly stochastic manifold allows to employ efficient Riemannian conjugate gradient algorithm for the proposed formulation. Empirically, the proposed approach outperforms state-of-the-art optimal transport based approach on the bilingual lexicon induction task across several language pairs. The performance improvement is more significant for distant language pairs.
You can open the
pre-recorded video
in a separate window.
NOTE: The SlidesLive video may display a random order of the authors.
The correct author list is shown at the top of this webpage.
Similar Papers
Knowledge Distillation for Multilingual Unsupervised Neural Machine Translation
Haipeng Sun, Rui Wang, Kehai Chen, Masao Utiyama, Eiichiro Sumita, Tiejun Zhao,
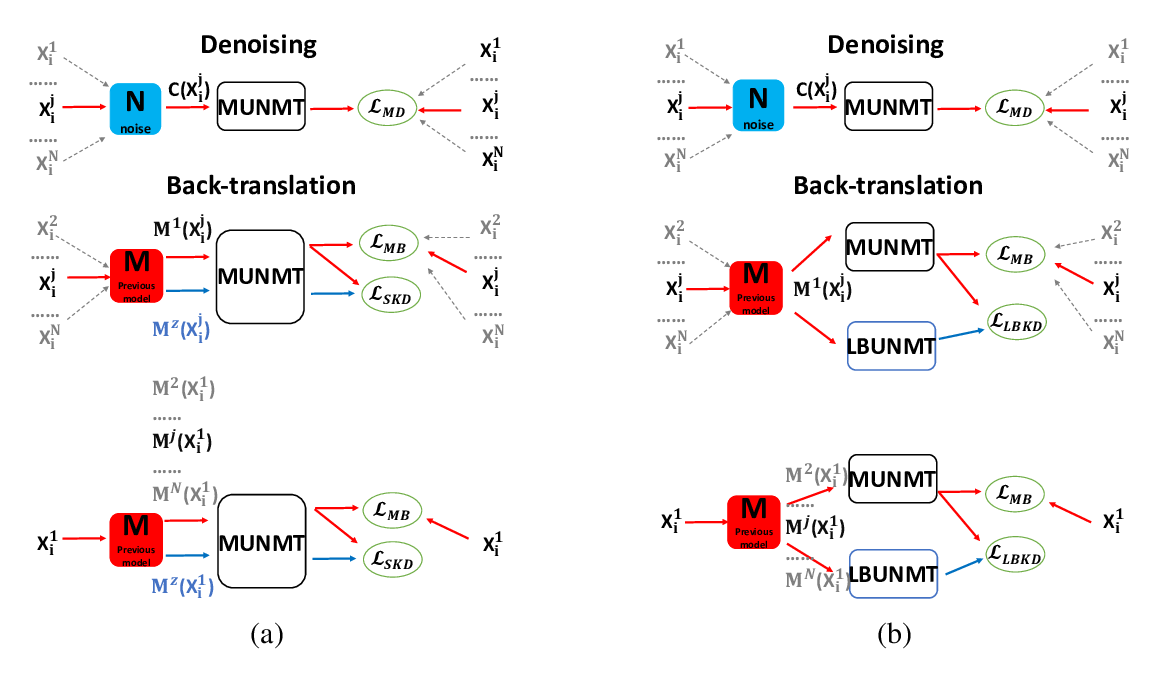
A Graph-based Coarse-to-fine Method for Unsupervised Bilingual Lexicon Induction
Shuo Ren, Shujie Liu, Ming Zhou, Shuai Ma,
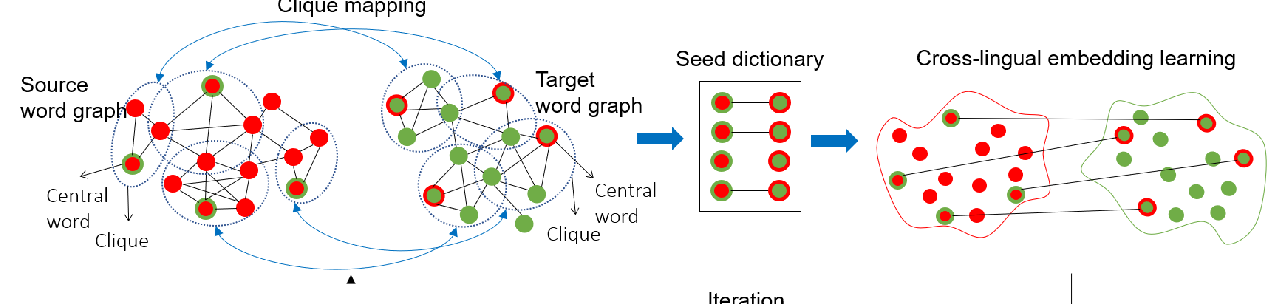
Unsupervised Multilingual Sentence Embeddings for Parallel Corpus Mining
Ivana Kvapilíková, Mikel Artetxe, Gorka Labaka, Eneko Agirre, Ondřej Bojar,
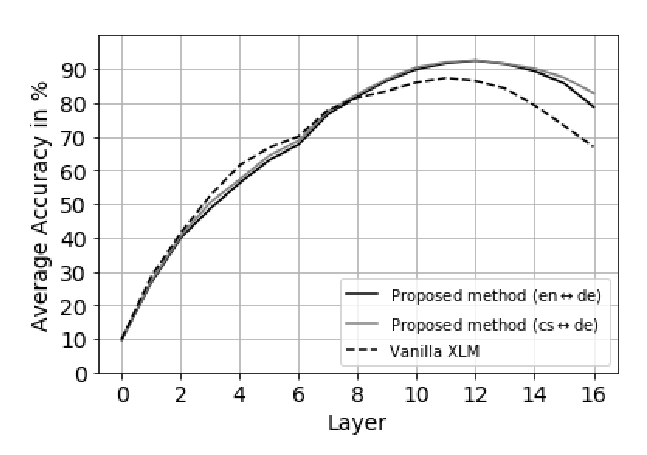