Unsupervised Multilingual Sentence Embeddings for Parallel Corpus Mining
Ivana Kvapilíková, Mikel Artetxe, Gorka Labaka, Eneko Agirre, Ondřej Bojar
Student Research Workshop SRW Paper
Session 7B: Jul 7
(09:00-10:00 GMT)
Session 8A: Jul 7
(12:00-13:00 GMT)
Abstract:
Existing models of multilingual sentence embeddings require large parallel data resources which are not available for low-resource languages. We propose a novel unsupervised method to derive multilingual sentence embeddings relying only on monolingual data. We first produce a synthetic parallel corpus using unsupervised machine translation, and use it to fine-tune a pretrained cross-lingual masked language model (XLM) to derive the multilingual sentence representations. The quality of the representations is evaluated on two parallel corpus mining tasks with improvements of up to 22 F1 points over vanilla XLM. In addition, we observe that a single synthetic bilingual corpus is able to improve results for other language pairs.
You can open the
pre-recorded video
in a separate window.
NOTE: The SlidesLive video may display a random order of the authors.
The correct author list is shown at the top of this webpage.
Similar Papers
Leveraging Monolingual Data with Self-Supervision for Multilingual Neural Machine Translation
Aditya Siddhant, Ankur Bapna, Yuan Cao, Orhan Firat, Mia Chen, Sneha Kudugunta, Naveen Arivazhagan, Yonghui Wu,
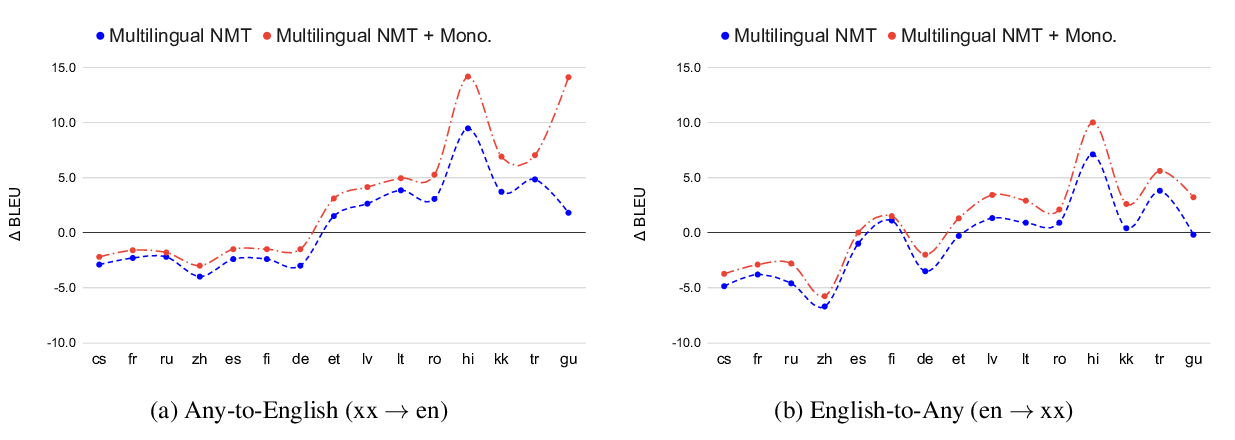
Knowledge Distillation for Multilingual Unsupervised Neural Machine Translation
Haipeng Sun, Rui Wang, Kehai Chen, Masao Utiyama, Eiichiro Sumita, Tiejun Zhao,
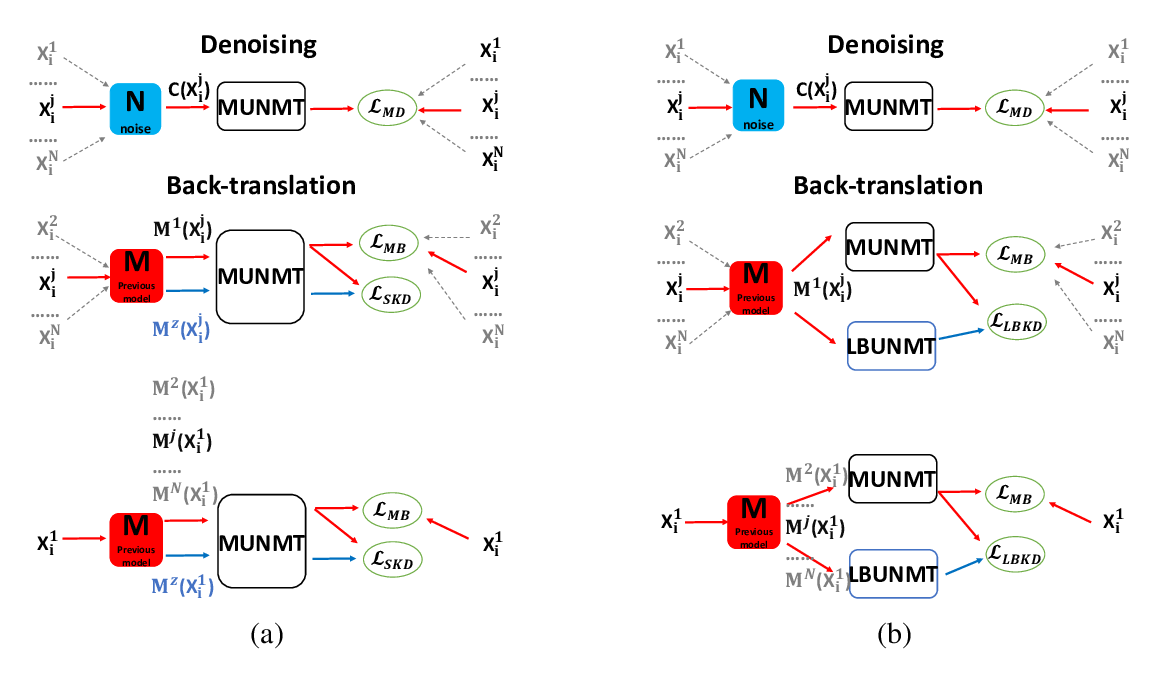
On the Cross-lingual Transferability of Monolingual Representations
Mikel Artetxe, Sebastian Ruder, Dani Yogatama,
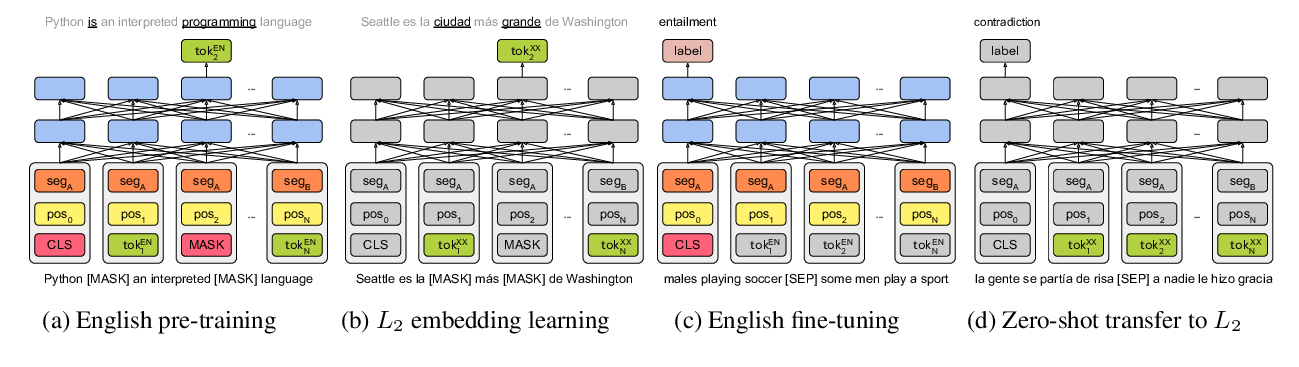
A Call for More Rigor in Unsupervised Cross-lingual Learning
Mikel Artetxe, Sebastian Ruder, Dani Yogatama, Gorka Labaka, Eneko Agirre,
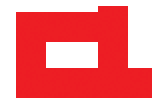