Learning to Recover from Multi-Modality Errors for Non-Autoregressive Neural Machine Translation
Qiu Ran, Yankai Lin, Peng Li, Jie Zhou
Machine Translation Long Paper
Session 6A: Jul 7
(05:00-06:00 GMT)
Session 7B: Jul 7
(09:00-10:00 GMT)
Abstract:
Non-autoregressive neural machine translation (NAT) predicts the entire target sequence simultaneously and significantly accelerates inference process. However, NAT discards the dependency information in a sentence, and thus inevitably suffers from the multi-modality problem: the target tokens may be provided by different possible translations, often causing token repetitions or missing. To alleviate this problem, we propose a novel semi-autoregressive model RecoverSAT in this work, which generates a translation as a sequence of segments. The segments are generated simultaneously while each segment is predicted token-by-token. By dynamically determining segment length and deleting repetitive segments, RecoverSAT is capable of recovering from repetitive and missing token errors. Experimental results on three widely-used benchmark datasets show that our proposed model achieves more than 4 times speedup while maintaining comparable performance compared with the corresponding autoregressive model.
You can open the
pre-recorded video
in a separate window.
NOTE: The SlidesLive video may display a random order of the authors.
The correct author list is shown at the top of this webpage.
Similar Papers
A Joint Model for Document Segmentation and Segment Labeling
Joe Barrow, Rajiv Jain, Vlad Morariu, Varun Manjunatha, Douglas Oard, Philip Resnik,
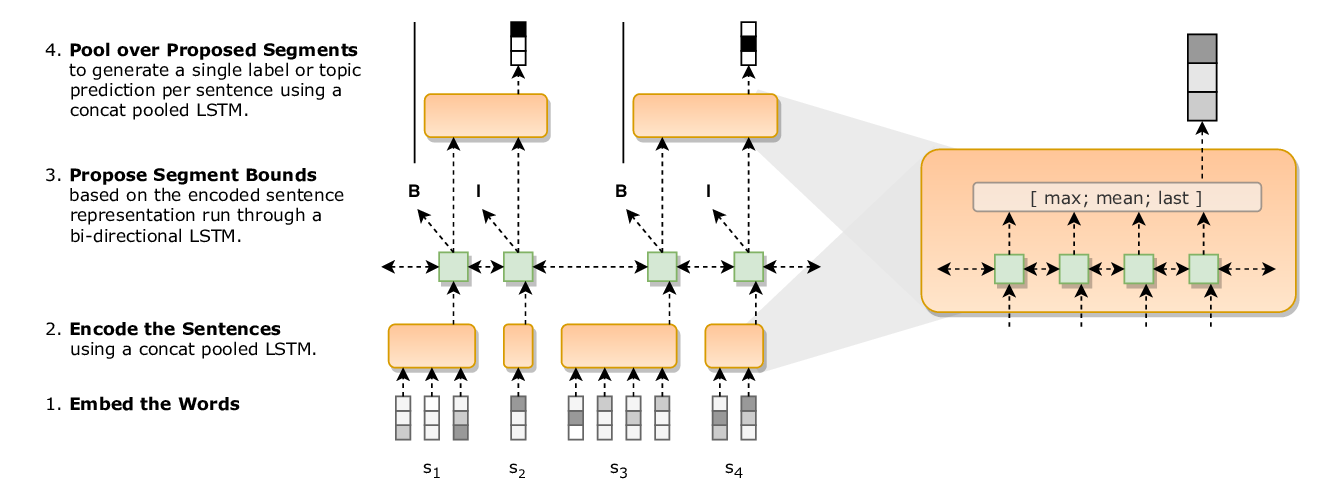
ENGINE: Energy-Based Inference Networks for Non-Autoregressive Machine Translation
Lifu Tu, Richard Yuanzhe Pang, Sam Wiseman, Kevin Gimpel,
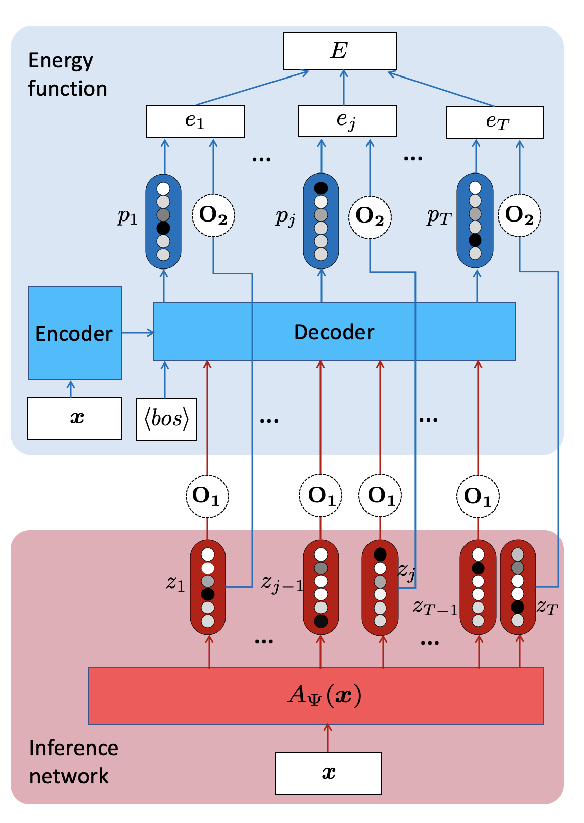
Neural Data-to-Text Generation via Jointly Learning the Segmentation and Correspondence
Xiaoyu Shen, Ernie Chang, Hui Su, Cheng Niu, Dietrich Klakow,
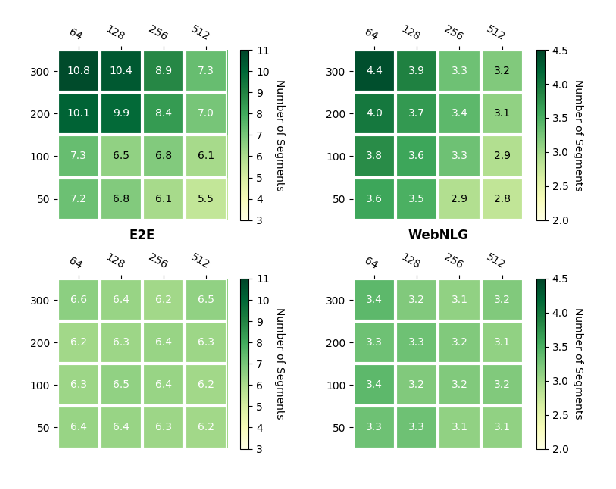
A Study of Non-autoregressive Model for Sequence Generation
Yi Ren, Jinglin Liu, Xu Tan, Zhou Zhao, Sheng Zhao, Tie-Yan Liu,
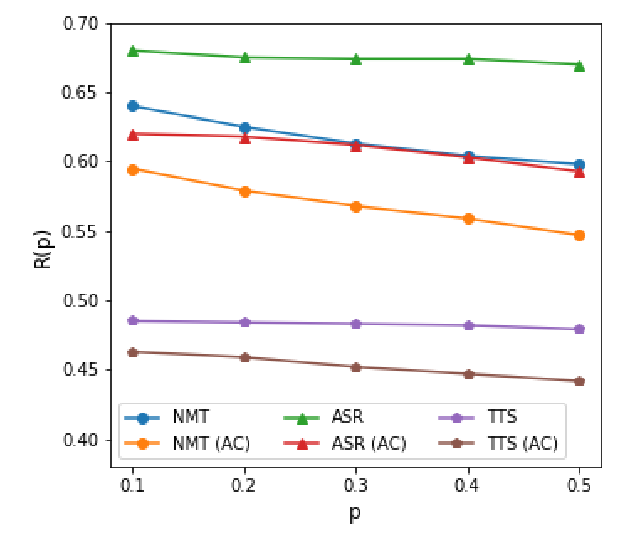