Improving Multimodal Named Entity Recognition via Entity Span Detection with Unified Multimodal Transformer
Jianfei Yu, Jing Jiang, Li Yang, Rui Xia
Computational Social Science and Social Media Long Paper
Session 6B: Jul 7
(06:00-07:00 GMT)
Session 8A: Jul 7
(12:00-13:00 GMT)
Abstract:
In this paper, we study Multimodal Named Entity Recognition (MNER) for social media posts. Existing approaches for MNER mainly suffer from two drawbacks: (1) despite generating word-aware visual representations, their word representations are insensitive to the visual context; (2) most of them ignore the bias brought by the visual context. To tackle the first issue, we propose a multimodal interaction module to obtain both image-aware word representations and word-aware visual representations. To alleviate the visual bias, we further propose to leverage purely text-based entity span detection as an auxiliary module, and design a Unified Multimodal Transformer to guide the final predictions with the entity span predictions. Experiments show that our unified approach achieves the new state-of-the-art performance on two benchmark datasets.
You can open the
pre-recorded video
in a separate window.
NOTE: The SlidesLive video may display a random order of the authors.
The correct author list is shown at the top of this webpage.
Similar Papers
Unsupervised Multimodal Neural Machine Translation with Pseudo Visual Pivoting
Po-Yao Huang, Junjie Hu, Xiaojun Chang, Alexander Hauptmann,
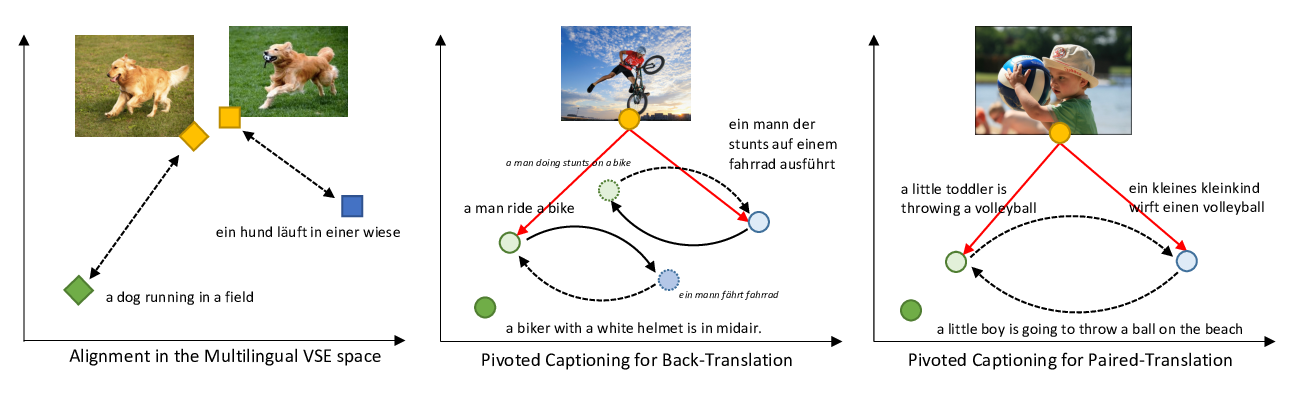
Cross-Modality Relevance for Reasoning on Language and Vision
Chen Zheng, Quan Guo, Parisa Kordjamshidi,
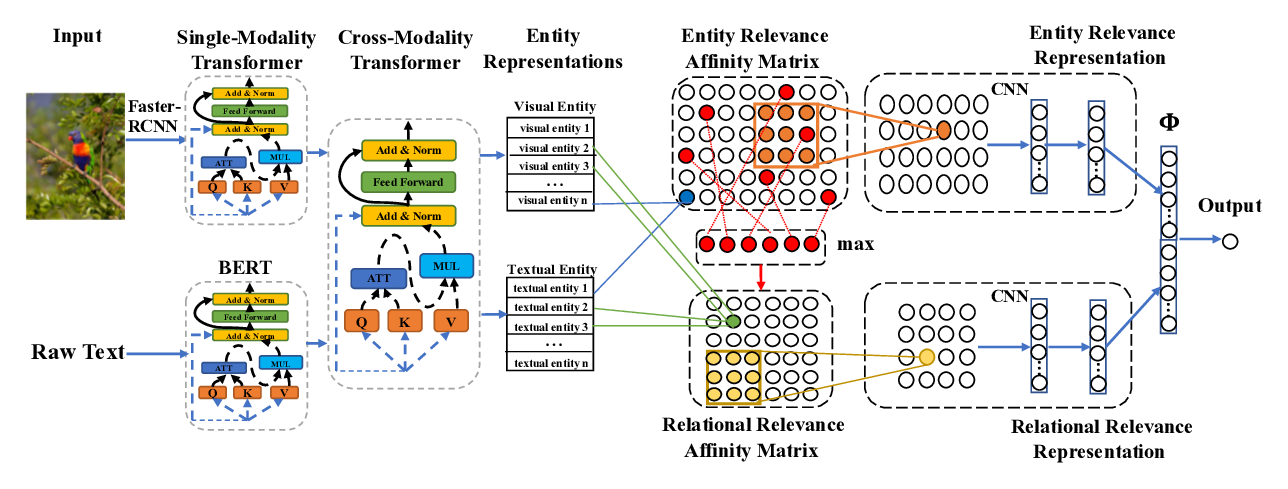