Multimodal Neural Graph Memory Networks for Visual Question Answering
Mahmoud Khademi
Language Grounding to Vision, Robotics and Beyond Long Paper
Session 12B: Jul 8
(09:00-10:00 GMT)
Session 13B: Jul 8
(13:00-14:00 GMT)
Abstract:
We introduce a new neural network architecture, Multimodal Neural Graph Memory Networks (MN-GMN), for visual question answering. Our novel approach uses graph structure with different region features as node attributes and applies a recently proposed powerful graph neural network model, Graph Network (GN), to reason about objects and their interactions in the scene context. The input module of the MN-GMN generates a set of visual features plus a set of region-grounded captions (RGCs) for the image. The RGCs capture object attributes and their relationships. Two GNs are constructed from the input module using visual features and RGCs. Each node of the GNs iteratively computes a question-guided contextualized representation of the visual/textual information assigned to it. To combine the information from both GNs, each node writes the updated representations to an external spatial memory. The final states of the memory cells are fed into an answer module to predict an answer. Experiments show that MN-GMN rivals the state-of-the-art models on the Visual7W, VQA-v2.0, and CLEVR datasets.
You can open the
pre-recorded video
in a separate window.
NOTE: The SlidesLive video may display a random order of the authors.
The correct author list is shown at the top of this webpage.
Similar Papers
Improving Multimodal Named Entity Recognition via Entity Span Detection with Unified Multimodal Transformer
Jianfei Yu, Jing Jiang, Li Yang, Rui Xia,
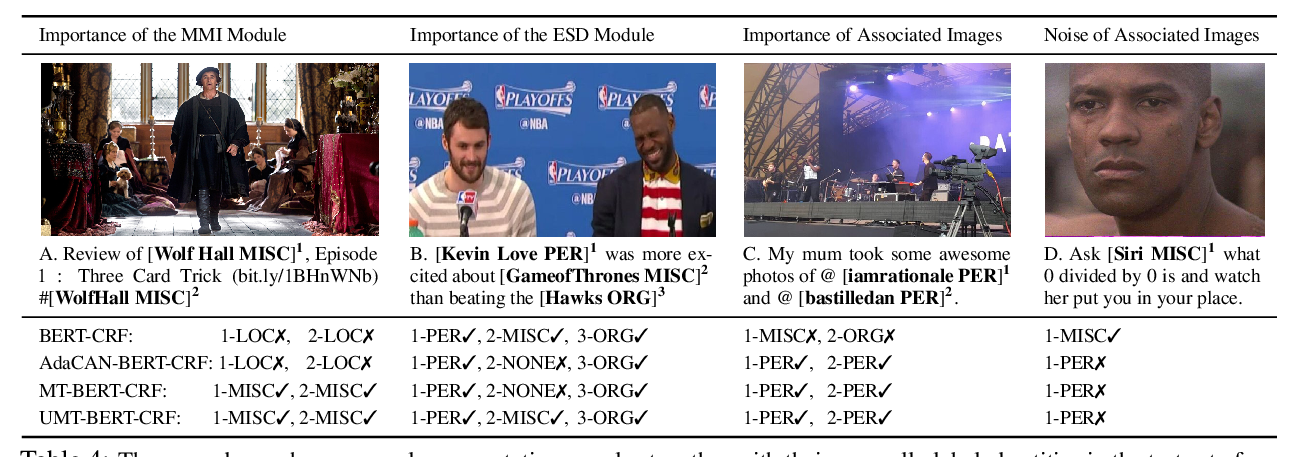
Aligned Dual Channel Graph Convolutional Network for Visual Question Answering
Qingbao Huang, Jielong Wei, Yi Cai, Changmeng Zheng, Junying Chen, Ho-fung Leung, Qing Li,
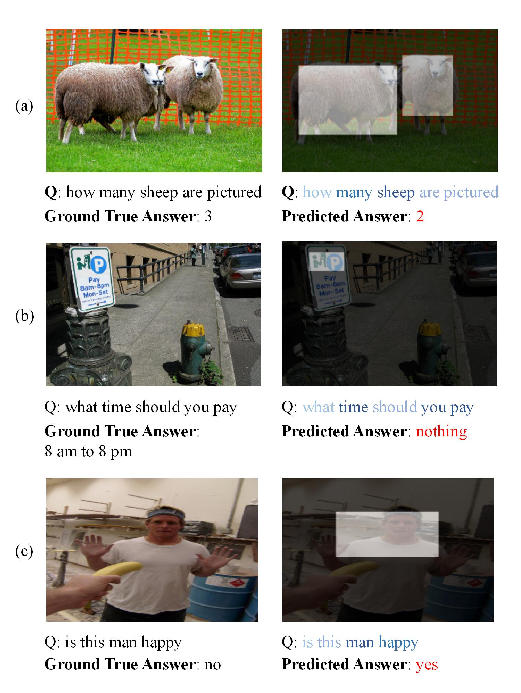
Cross-Modality Relevance for Reasoning on Language and Vision
Chen Zheng, Quan Guo, Parisa Kordjamshidi,
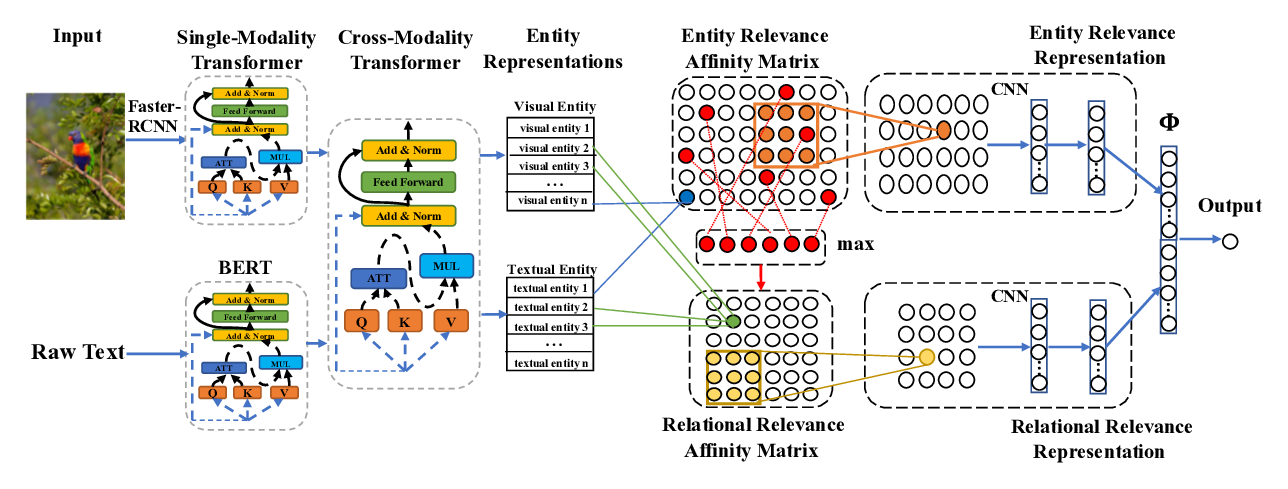