Improving Image Captioning with Better Use of Caption
Zhan Shi, Xu Zhou, Xipeng Qiu, Xiaodan Zhu
Generation Long Paper
Session 13A: Jul 8
(12:00-13:00 GMT)
Session 15A: Jul 8
(20:00-21:00 GMT)
Abstract:
Image captioning is a multimodal problem that has drawn extensive attention in both the natural language processing and computer vision community. In this paper, we present a novel image captioning architecture to better explore semantics available in captions and leverage that to enhance both image representation and caption generation. Our models first construct caption-guided visual relationship graphs that introduce beneficial inductive bias using weakly supervised multi-instance learning. The representation is then enhanced with neighbouring and contextual nodes with their textual and visual features. During generation, the model further incorporates visual relationships using multi-task learning for jointly predicting word and object/predicate tag sequences. We perform extensive experiments on the MSCOCO dataset, showing that the proposed framework significantly outperforms the baselines, resulting in the state-of-the-art performance under a wide range of evaluation metrics. The code of our paper has been made publicly available.
You can open the
pre-recorded video
in a separate window.
NOTE: The SlidesLive video may display a random order of the authors.
The correct author list is shown at the top of this webpage.
Similar Papers
Unsupervised Multimodal Neural Machine Translation with Pseudo Visual Pivoting
Po-Yao Huang, Junjie Hu, Xiaojun Chang, Alexander Hauptmann,
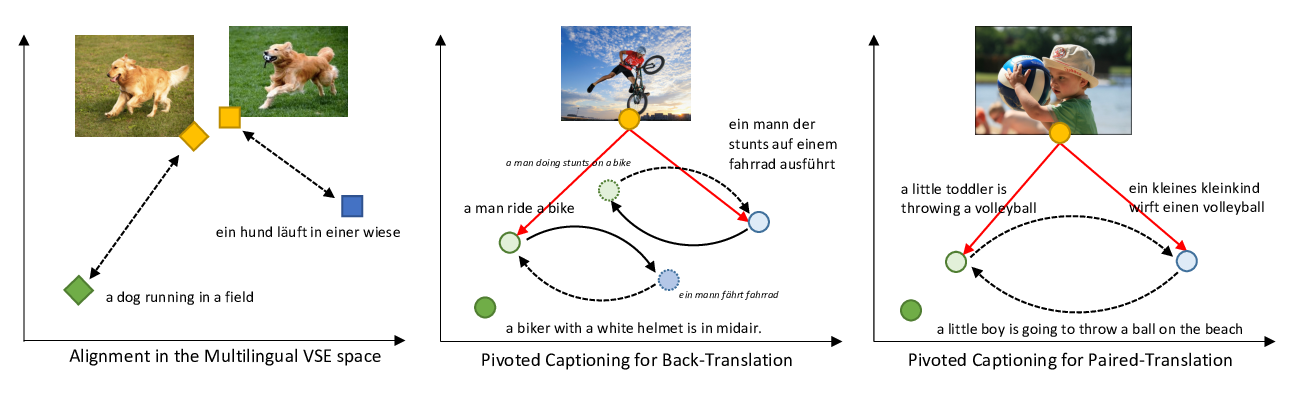
Improving Multimodal Named Entity Recognition via Entity Span Detection with Unified Multimodal Transformer
Jianfei Yu, Jing Jiang, Li Yang, Rui Xia,
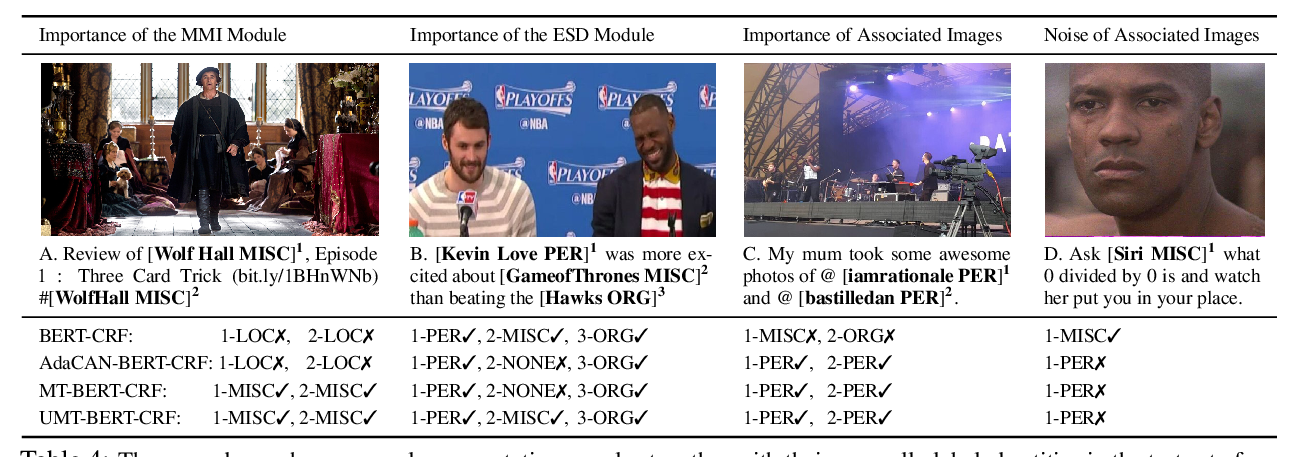
Cross-modal Coherence Modeling for Caption Generation
Malihe Alikhani, Piyush Sharma, Shengjie Li, Radu Soricut, Matthew Stone,
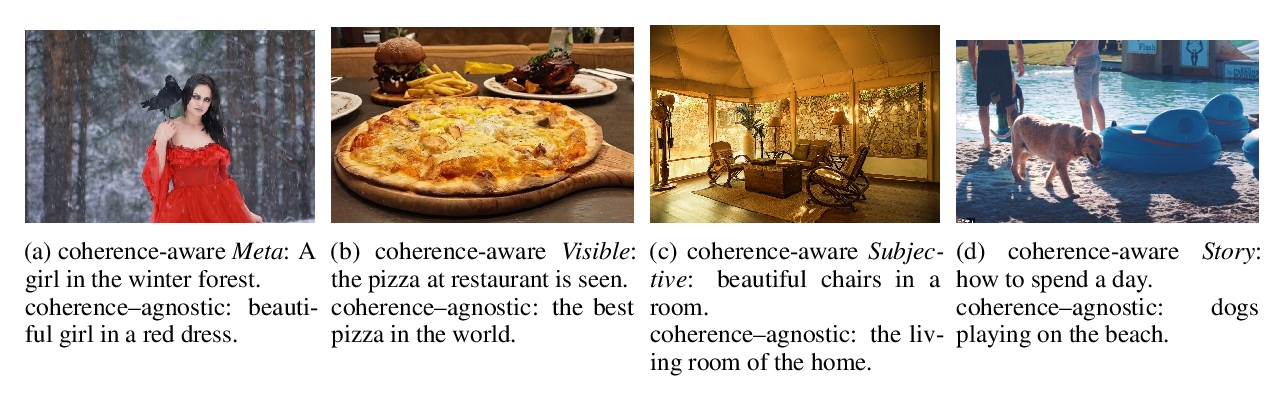
Cross-Modality Relevance for Reasoning on Language and Vision
Chen Zheng, Quan Guo, Parisa Kordjamshidi,
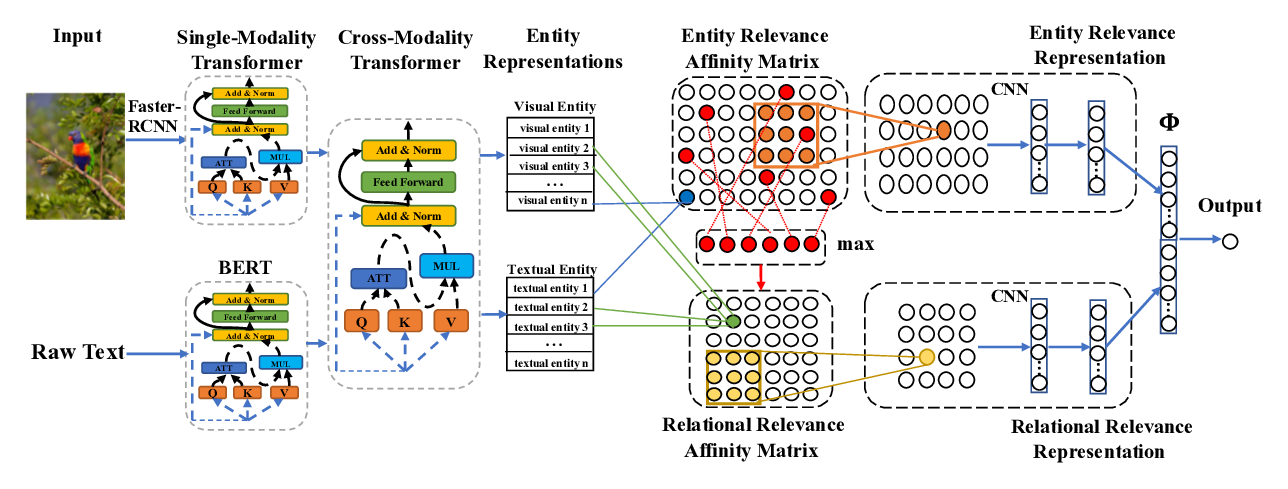