Every Document Owns Its Structure: Inductive Text Classification via Graph Neural Networks
Yufeng Zhang, Xueli Yu, Zeyu Cui, Shu Wu, Zhongzhen Wen, Liang Wang
Information Retrieval and Text Mining Short Paper
Session 1A: Jul 6
(05:00-06:00 GMT)
Session 2A: Jul 6
(08:00-09:00 GMT)
Abstract:
Text classification is fundamental in natural language processing (NLP) and Graph Neural Networks (GNN) are recently applied in this task. However, the existing graph-based works can neither capture the contextual word relationships within each document nor fulfil the inductive learning of new words. Therefore in this work, to overcome such problems, we propose TextING for inductive text classification via GNN. We first build individual graphs for each document and then use GNN to learn the fine-grained word representations based on their local structure, which can also effectively produce embeddings for unseen words in the new document. Finally, the word nodes are aggregated as the document embedding. Extensive experiments on four benchmark datasets show that our method outperforms state-of-the-art text classification methods.
You can open the
pre-recorded video
in a separate window.
NOTE: The SlidesLive video may display a random order of the authors.
The correct author list is shown at the top of this webpage.
Similar Papers
Taxonomy Construction of Unseen Domains via Graph-based Cross-Domain Knowledge Transfer
Chao Shang, Sarthak Dash, Md. Faisal Mahbub Chowdhury, Nandana Mihindukulasooriya, Alfio Gliozzo,
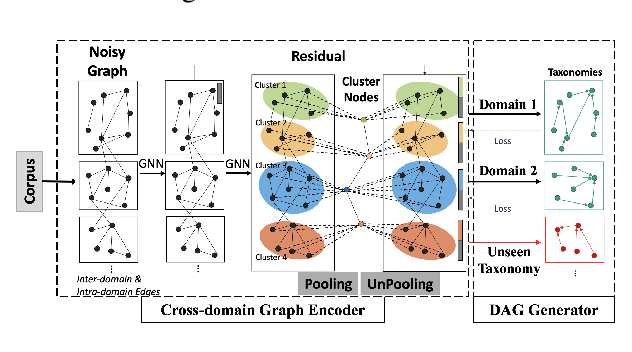
Heterogeneous Graph Neural Networks for Extractive Document Summarization
Danqing Wang, Pengfei Liu, Yining Zheng, Xipeng Qiu, Xuanjing Huang,
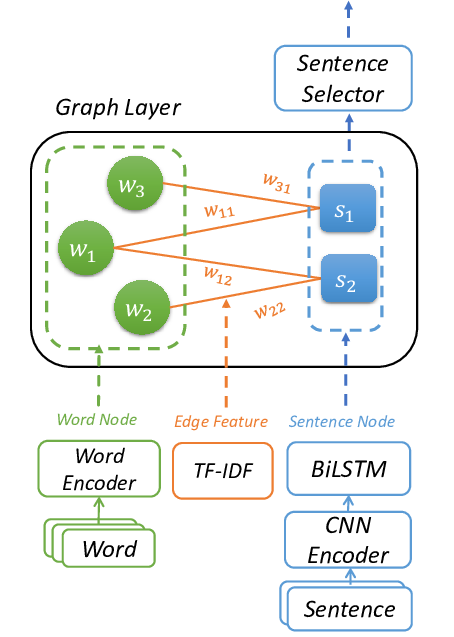
SPECTER: Document-level Representation Learning using Citation-informed Transformers
Arman Cohan, Sergey Feldman, Iz Beltagy, Doug Downey, Daniel Weld,
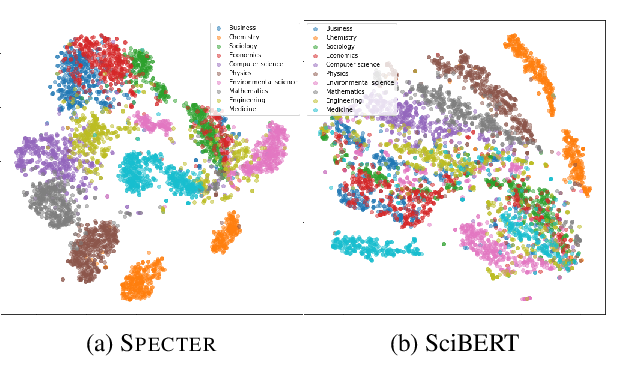
Autoencoding Keyword Correlation Graph for Document Clustering
Billy Chiu, Sunil Kumar Sahu, Derek Thomas, Neha Sengupta, Mohammady Mahdy,
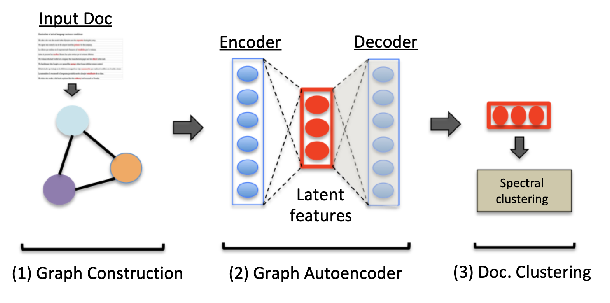