Heterogeneous Graph Neural Networks for Extractive Document Summarization
Danqing Wang, Pengfei Liu, Yining Zheng, Xipeng Qiu, Xuanjing Huang
Summarization Long Paper
Session 11A: Jul 8
(05:00-06:00 GMT)
Session 12A: Jul 8
(08:00-09:00 GMT)
Abstract:
As a crucial step in extractive document summarization, learning cross-sentence relations has been explored by a plethora of approaches. An intuitive way is to put them in the graph-based neural network, which has a more complex structure for capturing inter-sentence relationships. In this paper, we present a heterogeneous graph-based neural network for extractive summarization (HETERSUMGRAPH), which contains semantic nodes of different granularity levels apart from sentences. These additional nodes act as the intermediary between sentences and enrich the cross-sentence relations. Besides, our graph structure is flexible in natural extension from a single-document setting to multi-document via introducing document nodes. To our knowledge, we are the first one to introduce different types of nodes into graph-based neural networks for extractive document summarization and perform a comprehensive qualitative analysis to investigate their benefits. The code will be released on Github.
You can open the
pre-recorded video
in a separate window.
NOTE: The SlidesLive video may display a random order of the authors.
The correct author list is shown at the top of this webpage.
Similar Papers
Heterogeneous Graph Transformer for Graph-to-Sequence Learning
Shaowei Yao, Tianming Wang, Xiaojun Wan,
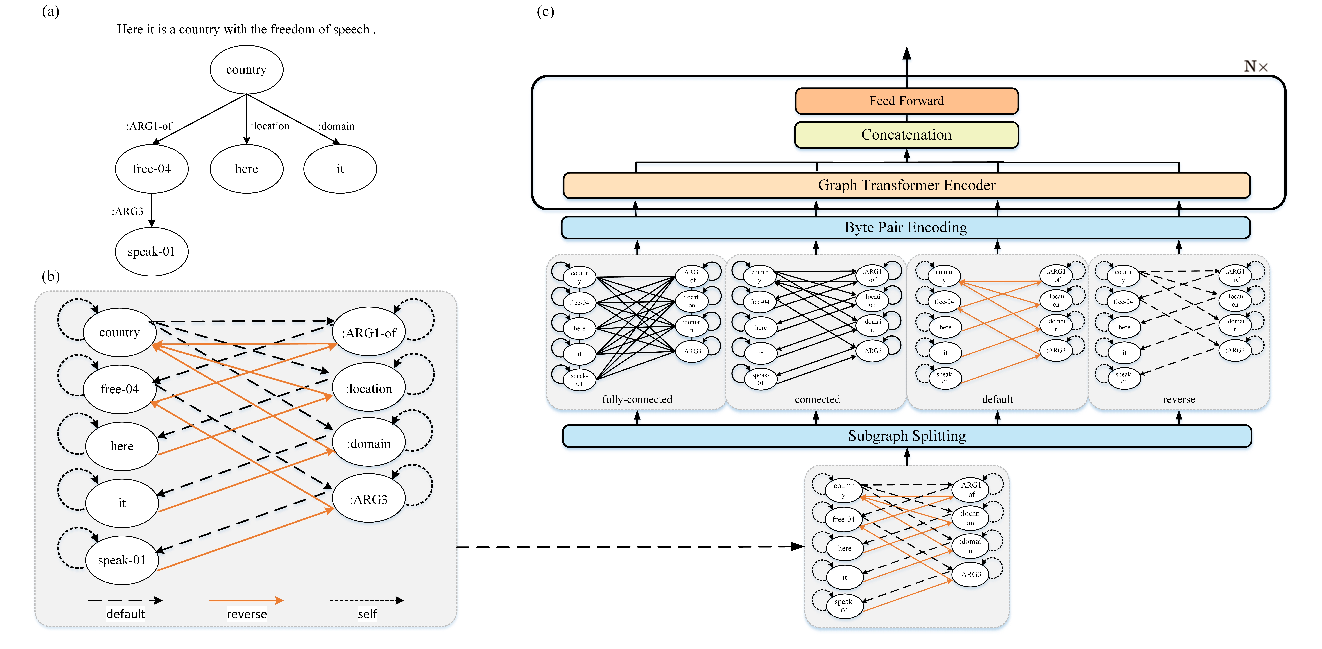
Orthogonal Relation Transforms with Graph Context Modeling for Knowledge Graph Embedding
Yun Tang, Jing Huang, Guangtao Wang, Xiaodong He, Bowen Zhou,
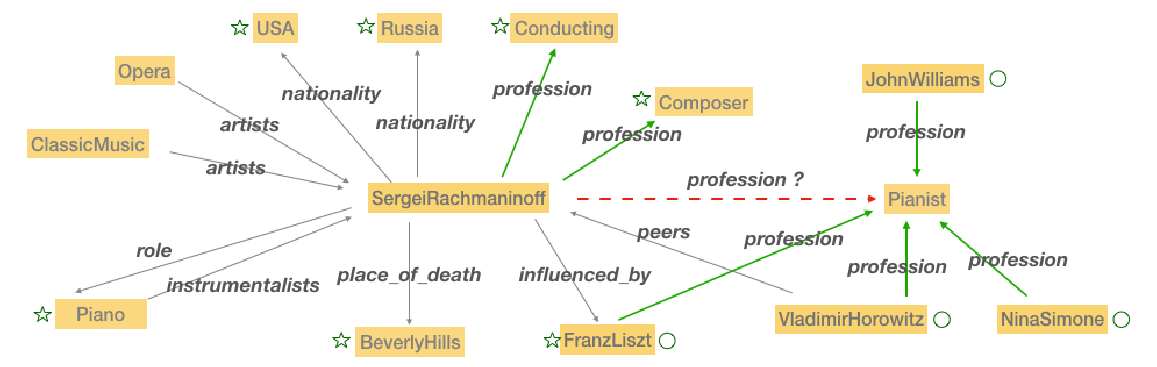
Extractive Summarization as Text Matching
Ming Zhong, Pengfei Liu, Yiran Chen, Danqing Wang, Xipeng Qiu, Xuanjing Huang,
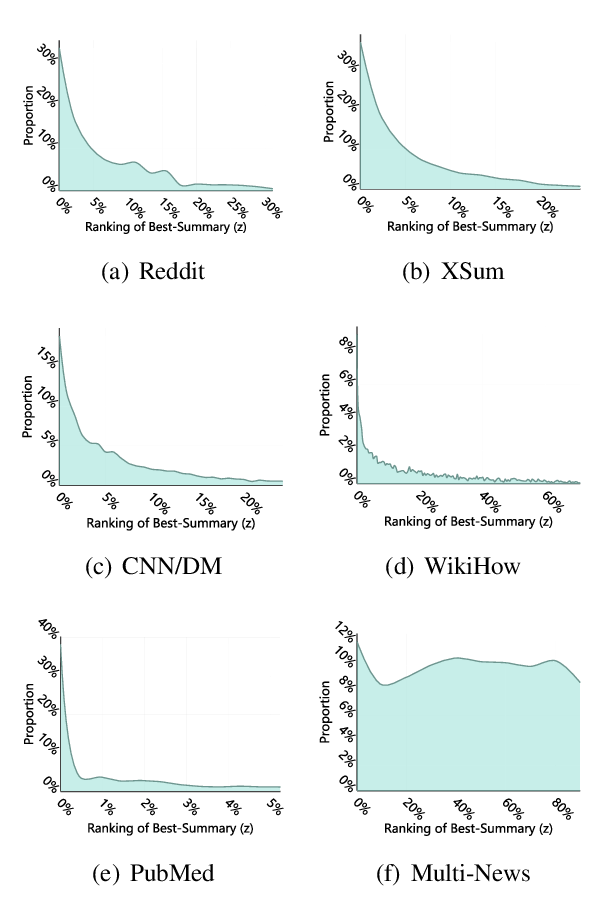
Leveraging Graph to Improve Abstractive Multi-Document Summarization
Wei Li, Xinyan Xiao, Jiachen Liu, Hua Wu, Haifeng Wang, Junping Du,
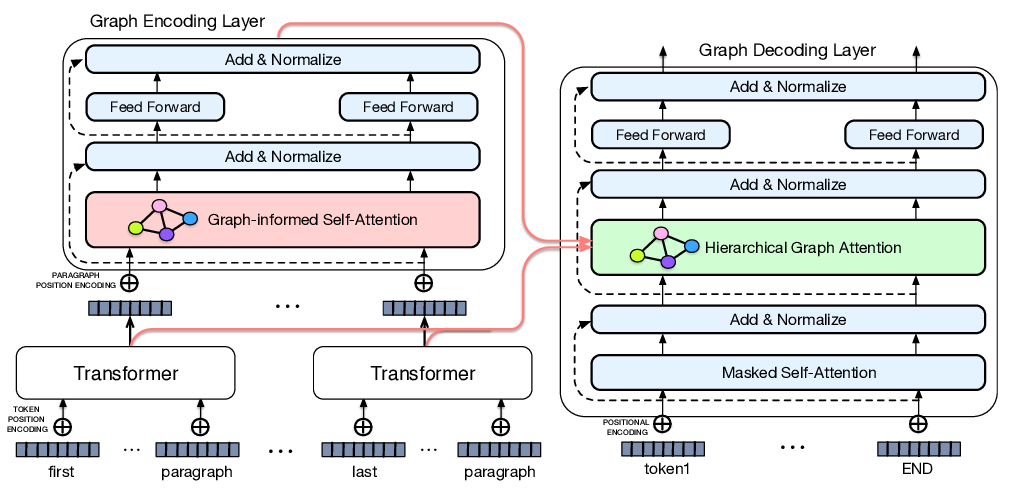