SPECTER: Document-level Representation Learning using Citation-informed Transformers
Arman Cohan, Sergey Feldman, Iz Beltagy, Doug Downey, Daniel Weld
NLP Applications Long Paper
Session 4A: Jul 6
(17:00-18:00 GMT)
Session 5B: Jul 6
(21:00-22:00 GMT)
Abstract:
Representation learning is a critical ingredient for natural language processing systems. Recent Transformer language models like BERT learn powerful textual representations, but these models are targeted towards token- and sentence-level training objectives and do not leverage information on inter-document relatedness, which limits their document-level representation power. For applications on scientific documents, such as classification and recommendation, accurate embeddings of documents are a necessity. We propose SPECTER, a new method to generate document-level embedding of scientific papers based on pretraining a Transformer language model on a powerful signal of document-level relatedness: the citation graph. Unlike existing pretrained language models, Specter can be easily applied to downstream applications without task-specific fine-tuning. Additionally, to encourage further research on document-level models, we introduce SciDocs, a new evaluation benchmark consisting of seven document-level tasks ranging from citation prediction, to document classification and recommendation. We show that Specter outperforms a variety of competitive baselines on the benchmark.
You can open the
pre-recorded video
in a separate window.
NOTE: The SlidesLive video may display a random order of the authors.
The correct author list is shown at the top of this webpage.
Similar Papers
SciREX: A Challenge Dataset for Document-Level Information Extraction
Sarthak Jain, Madeleine van Zuylen, Hannaneh Hajishirzi, Iz Beltagy,
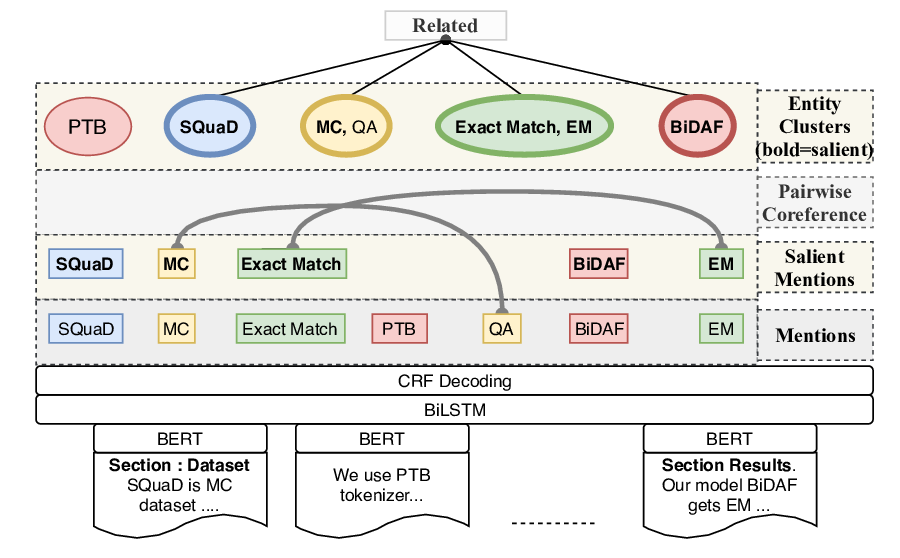
Reasoning with Latent Structure Refinement for Document-Level Relation Extraction
Guoshun Nan, Zhijiang Guo, Ivan Sekulic, Wei Lu,
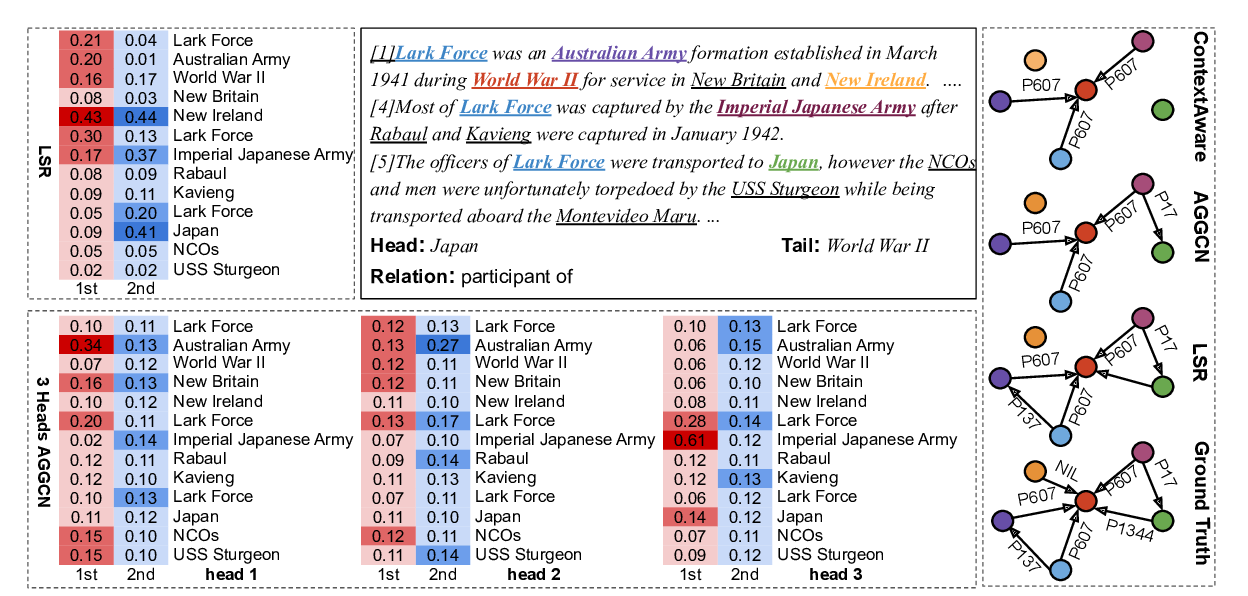
Using Context in Neural Machine Translation Training Objectives
Danielle Saunders, Felix Stahlberg, Bill Byrne,
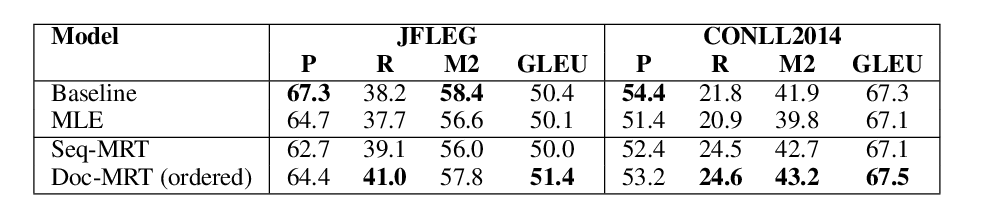
A Simple and Effective Unified Encoder for Document-Level Machine Translation
Shuming Ma, Dongdong Zhang, Ming Zhou,
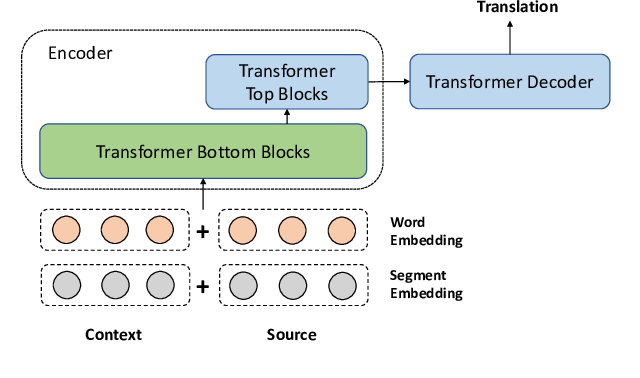