A Retrieve-and-Rewrite Initialization Method for Unsupervised Machine Translation
Shuo Ren, Yu Wu, Shujie Liu, Ming Zhou, Shuai Ma
Machine Translation Short Paper
Session 6B: Jul 7
(06:00-07:00 GMT)
Session 7B: Jul 7
(09:00-10:00 GMT)
Abstract:
The commonly used framework for unsupervised machine translation builds initial translation models of both translation directions, and then performs iterative back-translation to jointly boost their translation performance. The initialization stage is very important since bad initialization may wrongly squeeze the search space, and too much noise introduced in this stage may hurt the final performance. In this paper, we propose a novel retrieval and rewriting based method to better initialize unsupervised translation models. We first retrieve semantically comparable sentences from monolingual corpora of two languages and then rewrite the target side to minimize the semantic gap between the source and retrieved targets with a designed rewriting model. The rewritten sentence pairs are used to initialize SMT models which are used to generate pseudo data for two NMT models, followed by the iterative back-translation. Experiments show that our method can build better initial unsupervised translation models and improve the final translation performance by over 4 BLEU scores. Our code is released at https://github.com/Imagist-Shuo/RRforUNMT.git.
You can open the
pre-recorded video
in a separate window.
NOTE: The SlidesLive video may display a random order of the authors.
The correct author list is shown at the top of this webpage.
Similar Papers
It's Easier to Translate out of English than into it: Measuring Neural Translation Difficulty by Cross-Mutual Information
Emanuele Bugliarello, Sabrina J. Mielke, Antonios Anastasopoulos, Ryan Cotterell, Naoaki Okazaki,
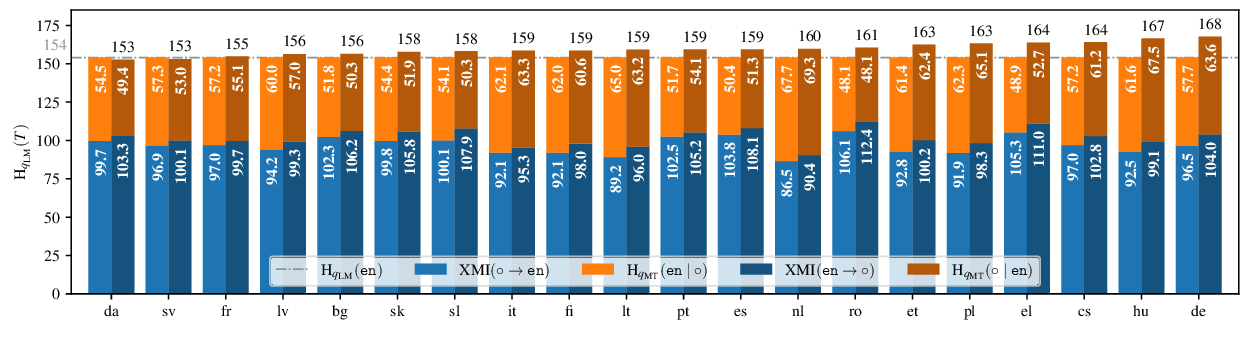
SUPERT: Towards New Frontiers in Unsupervised Evaluation Metrics for Multi-Document Summarization
Yang Gao, Wei Zhao, Steffen Eger,
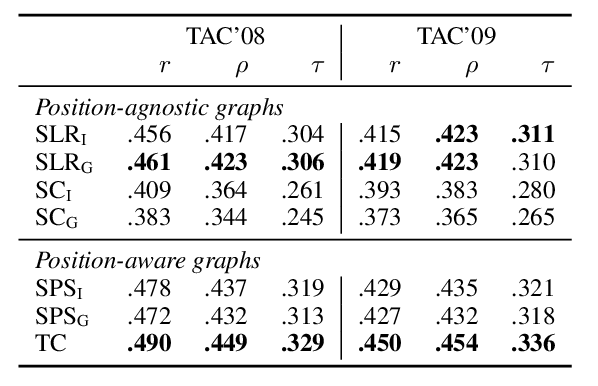
On The Evaluation of Machine Translation SystemsTrained With Back-Translation
Sergey Edunov, Myle Ott, Marc'Aurelio Ranzato, Michael Auli,
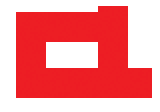
Improving Event Detection via Open-domain Trigger Knowledge
Meihan Tong, Bin Xu, Shuai Wang, Yixin Cao, Lei Hou, Juanzi Li, Jun Xie,
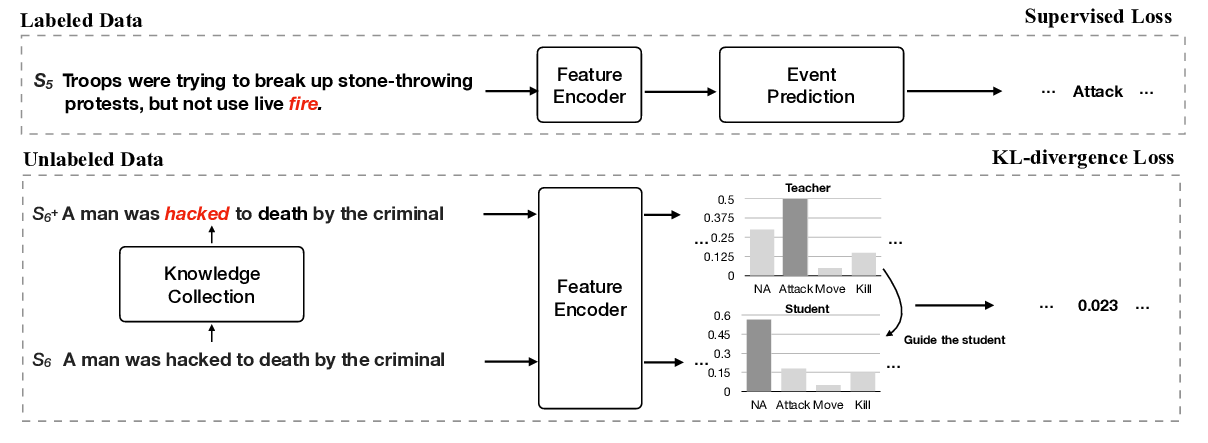