On The Evaluation of Machine Translation SystemsTrained With Back-Translation
Sergey Edunov, Myle Ott, Marc'Aurelio Ranzato, Michael Auli
Machine Translation Long Paper
Session 4B: Jul 6
(18:00-19:00 GMT)
Session 5A: Jul 6
(20:00-21:00 GMT)
Abstract:
Back-translation is a widely used data augmentation technique which leverages target monolingual data. However, its effectiveness has been challenged since automatic metrics such as BLEU only show significant improvements for test examples where the source itself is a translation, or translationese. This is believed to be due to translationese inputs better matching the back-translated training data. In this work, we show that this conjecture is not empirically supported and that back-translation improves translation quality of both naturally occurring text as well as translationese according to professional human translators. We provide empirical evidence to support the view that back-translation is preferred by humans because it produces more fluent outputs. BLEU cannot capture human preferences because references are translationese when source sentences are natural text. We recommend complementing BLEU with a language model score to measure fluency.
You can open the
pre-recorded video
in a separate window.
NOTE: The SlidesLive video may display a random order of the authors.
The correct author list is shown at the top of this webpage.
Similar Papers
Translationese as a Language in "Multilingual" NMT
Parker Riley, Isaac Caswell, Markus Freitag, David Grangier,
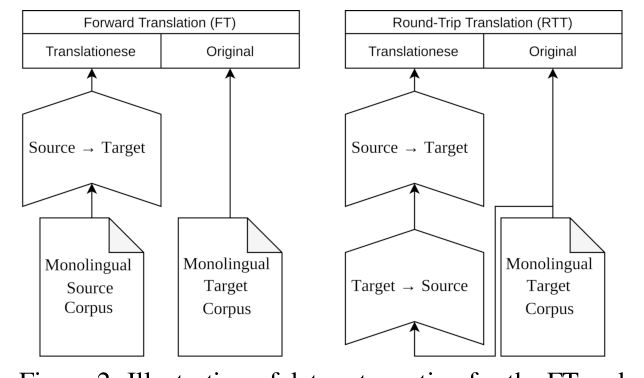
Tagged Back-translation Revisited: Why Does It Really Work?
Benjamin Marie, Raphael Rubino, Atsushi Fujita,
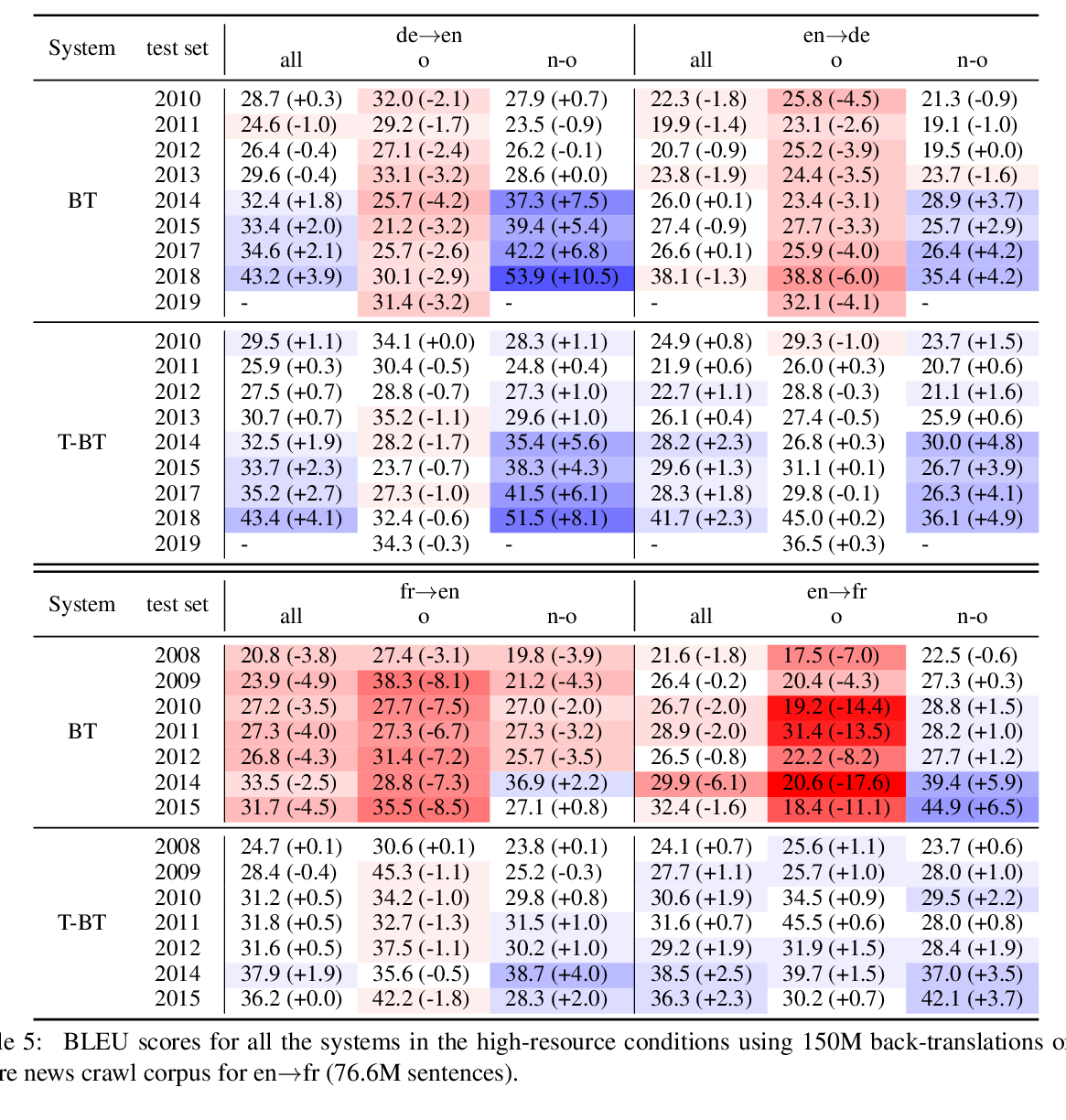
A Retrieve-and-Rewrite Initialization Method for Unsupervised Machine Translation
Shuo Ren, Yu Wu, Shujie Liu, Ming Zhou, Shuai Ma,
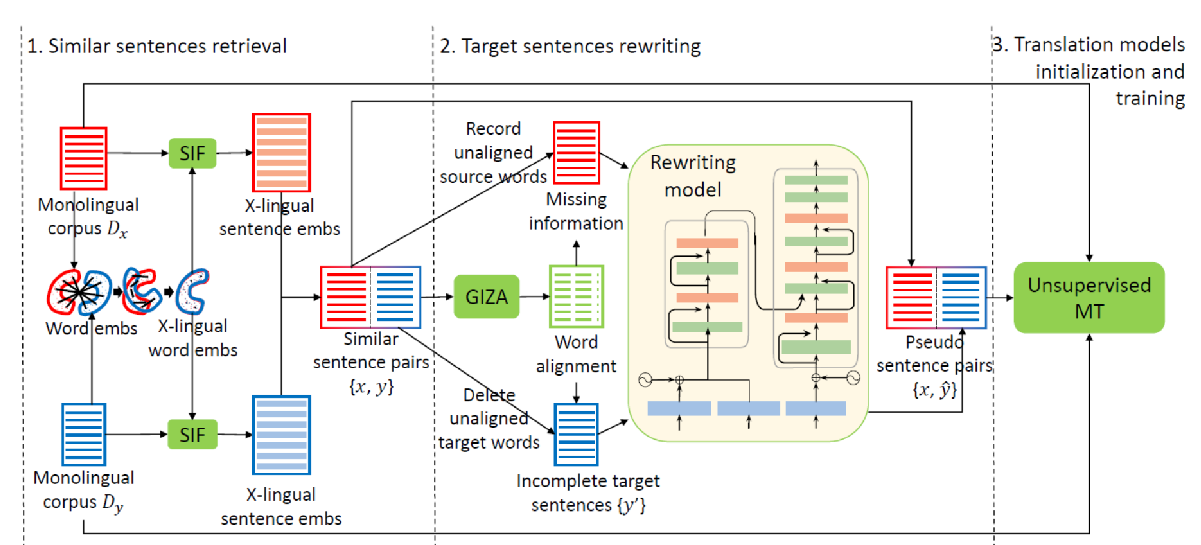