A Simple and Effective Unified Encoder for Document-Level Machine Translation
Shuming Ma, Dongdong Zhang, Ming Zhou
Machine Translation Short Paper
Session 6B: Jul 7
(06:00-07:00 GMT)
Session 7B: Jul 7
(09:00-10:00 GMT)
Abstract:
Most of the existing models for document-level machine translation adopt dual-encoder structures. The representation of the source sentences and the document-level contexts (In this work, document-level contexts denote the surrounding sentences of the current source sentence.) are modeled with two separate encoders. Although these models can make use of the document-level contexts, they do not fully model the interaction between the contexts and the source sentences, and can not directly adapt to the recent pre-training models (e.g., BERT) which encodes multiple sentences with a single encoder. In this work, we propose a simple and effective unified encoder that can outperform the baseline models of dual-encoder models in terms of BLEU and METEOR scores. Moreover, the pre-training models can further boost the performance of our proposed model.
You can open the
pre-recorded video
in a separate window.
NOTE: The SlidesLive video may display a random order of the authors.
The correct author list is shown at the top of this webpage.
Similar Papers
SPECTER: Document-level Representation Learning using Citation-informed Transformers
Arman Cohan, Sergey Feldman, Iz Beltagy, Doug Downey, Daniel Weld,
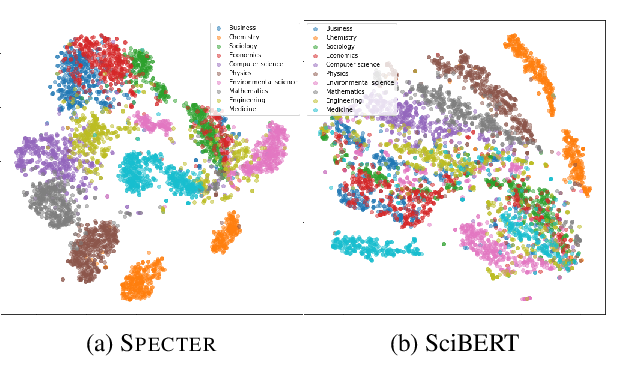
Reasoning with Latent Structure Refinement for Document-Level Relation Extraction
Guoshun Nan, Zhijiang Guo, Ivan Sekulic, Wei Lu,
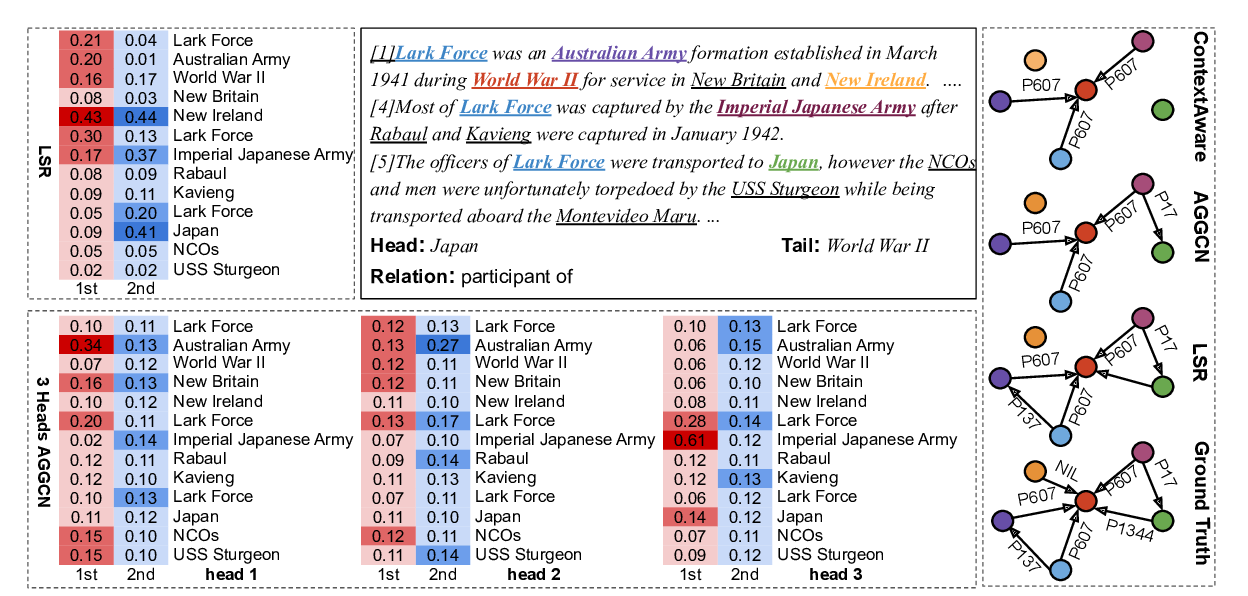
Does Multi-Encoder Help? A Case Study on Context-Aware Neural Machine Translation
Bei Li, Hui Liu, Ziyang Wang, Yufan Jiang, Tong Xiao, Jingbo Zhu, Tongran Liu, Changliang Li,
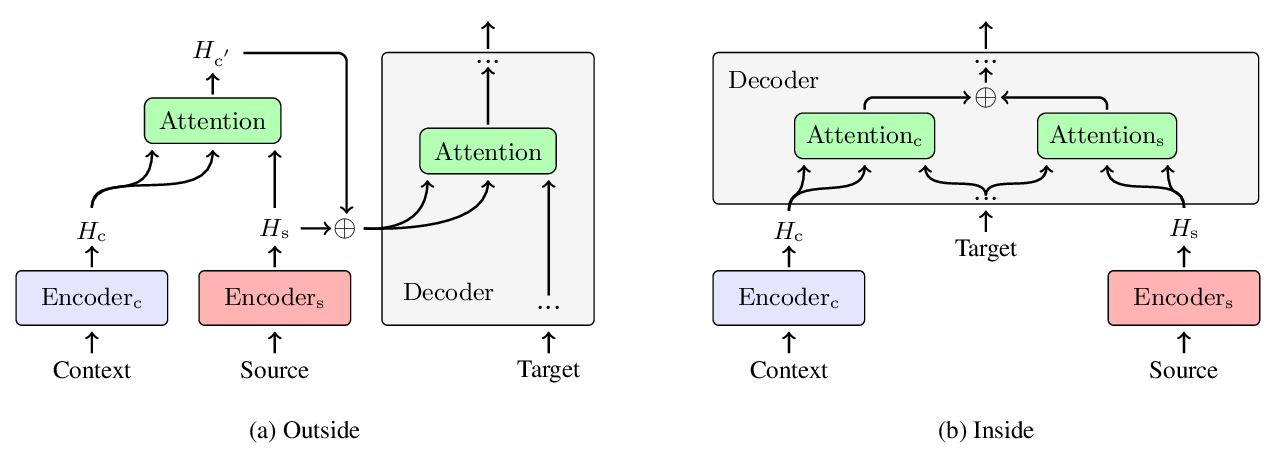
SciREX: A Challenge Dataset for Document-Level Information Extraction
Sarthak Jain, Madeleine van Zuylen, Hannaneh Hajishirzi, Iz Beltagy,
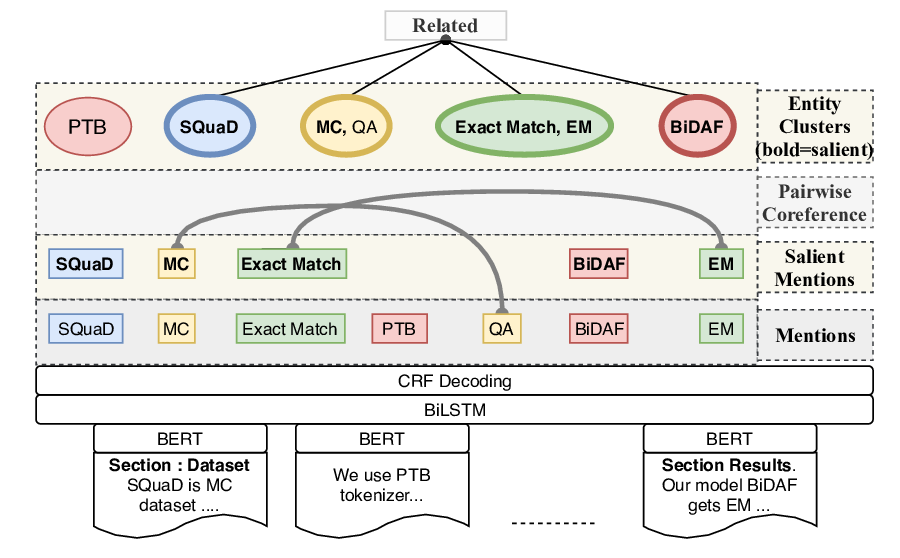