Biomedical Entity Representations with Synonym Marginalization
Mujeen Sung, Hwisang Jeon, Jinhyuk Lee, Jaewoo Kang
Semantics: Lexical Long Paper
Session 6B: Jul 7
(06:00-07:00 GMT)
Session 7A: Jul 7
(08:00-09:00 GMT)
Abstract:
Biomedical named entities often play important roles in many biomedical text mining tools. However, due to the incompleteness of provided synonyms and numerous variations in their surface forms, normalization of biomedical entities is very challenging. In this paper, we focus on learning representations of biomedical entities solely based on the synonyms of entities. To learn from the incomplete synonyms, we use a model-based candidate selection and maximize the marginal likelihood of the synonyms present in top candidates. Our model-based candidates are iteratively updated to contain more difficult negative samples as our model evolves. In this way, we avoid the explicit pre-selection of negative samples from more than 400K candidates. On four biomedical entity normalization datasets having three different entity types (disease, chemical, adverse reaction), our model BioSyn consistently outperforms previous state-of-the-art models almost reaching the upper bound on each dataset.
You can open the
pre-recorded video
in a separate window.
NOTE: The SlidesLive video may display a random order of the authors.
The correct author list is shown at the top of this webpage.
Similar Papers
An Effective Transition-based Model for Discontinuous NER
Xiang Dai, Sarvnaz Karimi, Ben Hachey, Cecile Paris,
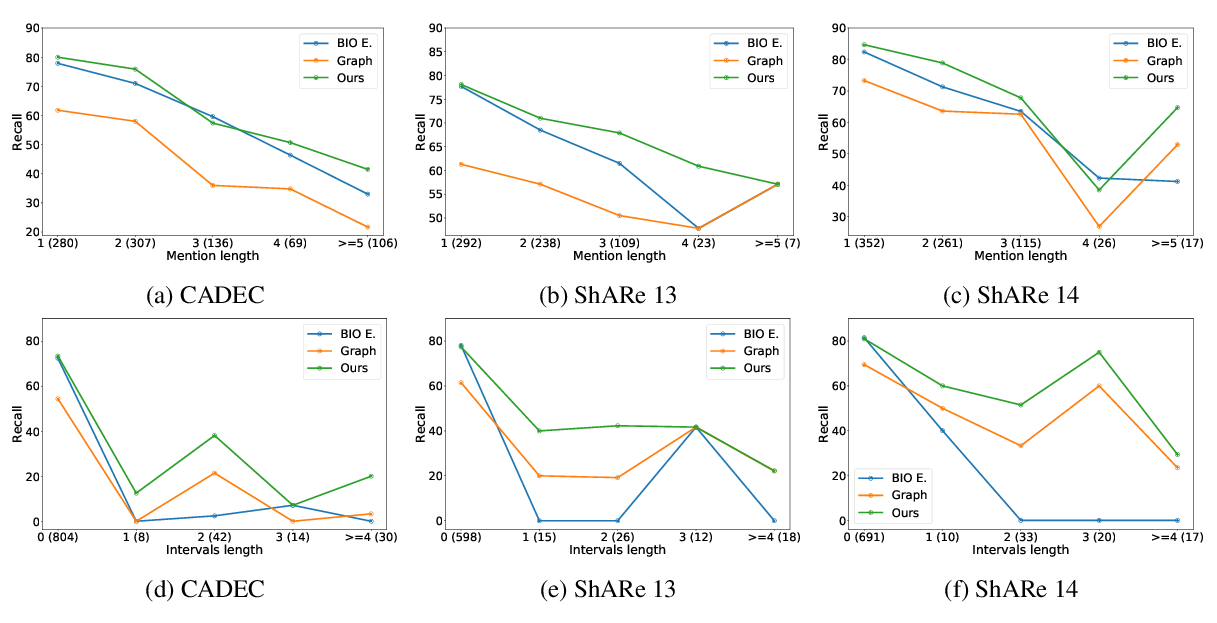